A Lightweight Transformer Network for Hyperspectral Image Classification.
IEEE Trans. Geosci. Remote. Sens.(2023)
摘要
Transformer is a powerful tool for capturing long-range dependencies and has shown impressive performance in hyperspectral image (HSI) classification. However, such power comes with a heavy memory footprint and huge computation burden. In this article, we propose two types of lightweight self-attention modules (a channel lightweight multihead self-attention (CLMSA) module and a position lightweight multihead self-attention (PLMSA) module) to reduce both memory and computation while associating each pixel or channel with global information. Moreover, we discover that transformers are ineffective in explicitly extracting local and multiscale features due to the fixed input size and tend to overfit when dealing with a small number of training samples. Therefore, a lightweight transformer (LiT) network, built with the proposed lightweight self-attention modules, is presented. LiT adopts convolutional blocks to explicitly extract local information in early layers and employs transformers to capture long-range dependencies in deep layers. Furthermore, we design a controlled multiclass stratified (CMS) sampling strategy to generate appropriately sized input data, ensure balanced sampling, and reduce the overlap of feature extraction regions between training and test samples. With appropriate training data, convolutional tokenization, and LiTs, LiT mitigates overfitting and enjoys both high computational efficiency and good performance. Experimental results on several HSI datasets verify the effectiveness of our design.
更多查看译文
关键词
Deep learning (DL),hyperspectral image (HSI) classification,transformer
AI 理解论文
溯源树
样例
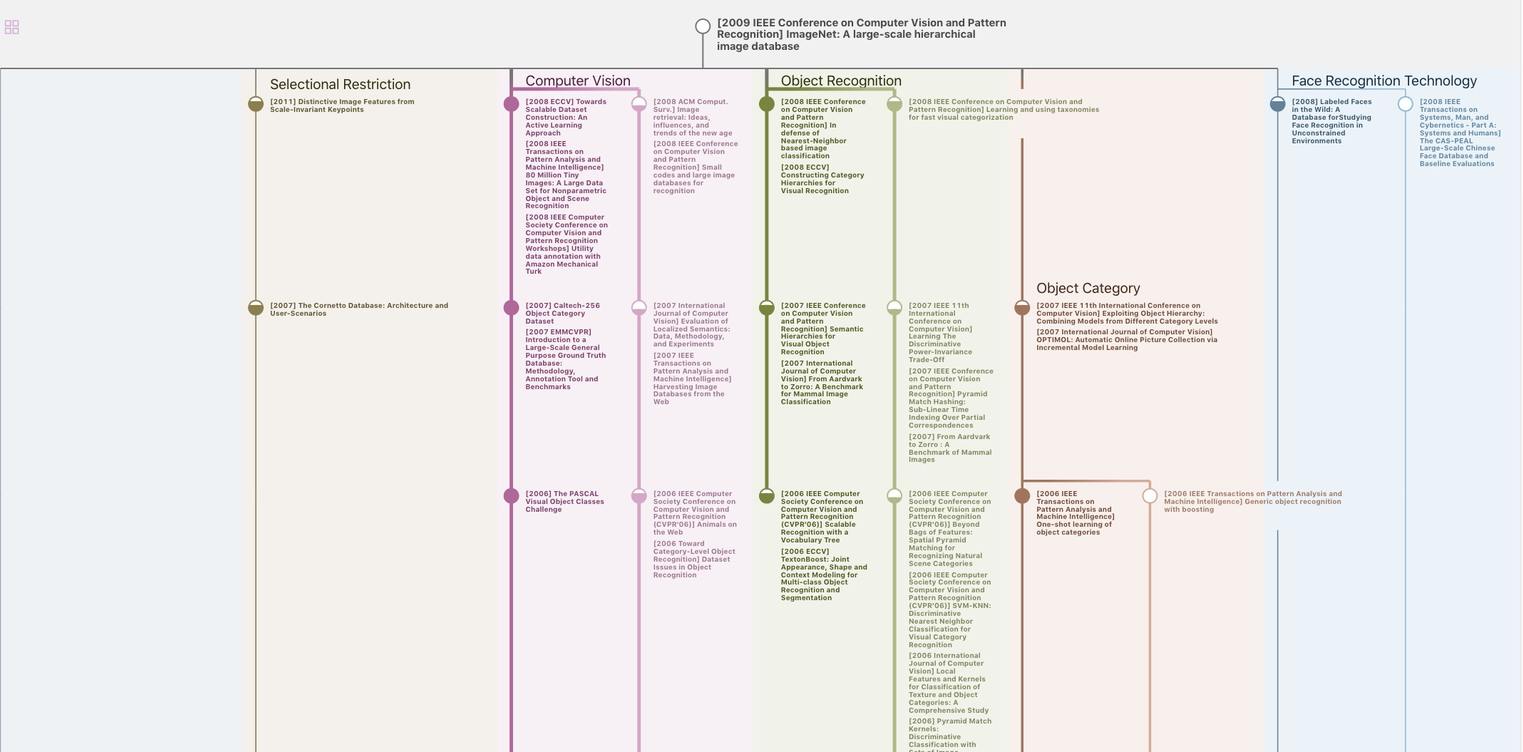
生成溯源树,研究论文发展脉络
Chat Paper
正在生成论文摘要