Bounding convolutional network for refining object locations
Neural Comput. Appl.(2023)
摘要
Object detection, an important task in computer vision, has achieved a conspicuous improvement. Among the methods for object detection, the one-stage detector is a simple, end-to-end, anchor-free, and straightforward deep learning pipeline. Many one-stage detectors locate the bounding box using regression, and the regression loss is the maximum among all errors. Hence, locating the bounding box accurately is one of the keys for improving the average precision (AP) for a detector. In this paper, we suggest a simple and precise locator named the bounding convolutional network (BoundConvNet) to draw “bounding features” from heatmaps to refine the object locations and apply a category-aware collaborative intersection over union (Co-IoU) loss function to optimize the bounding box regression for dealing with a problem of different class center point overlap. BoundConvNet is a head network for bounding box regression, which contains several depthwise separable dilated convolutional layers to decouple the classification task from the regression task. Extensive experiments demonstrate that BoundConvNet improves the AP of the one-stage detector CenterNet and helps the CenterNet mark the bounding box of objects more accurately. For small object detection, the AP of CenterNet is improved by 13.8% relative on MS COCO dataset with ResNet-18 as backbone.
更多查看译文
关键词
convolutional network,object
AI 理解论文
溯源树
样例
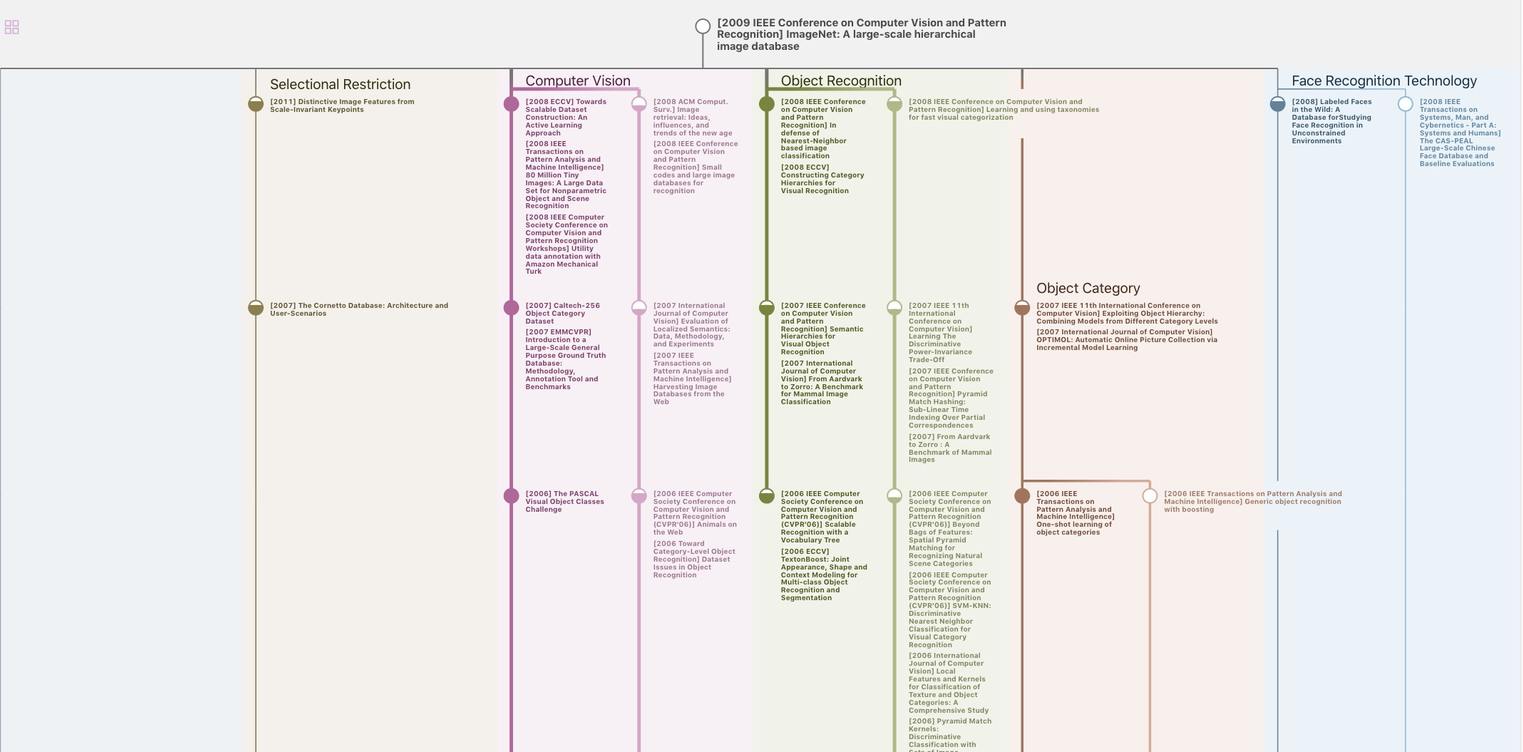
生成溯源树,研究论文发展脉络
Chat Paper
正在生成论文摘要