Pruning method for dendritic neuron model based on dendrite layer significance constraints.
CAAI Trans. Intell. Technol.(2023)
摘要
The dendritic neural model (DNM) mimics the non-linearity of synapses in the human brain to simulate the information processing mechanisms and procedures of neurons. This enhances the understanding of biological nervous systems and the applicability of the model in various fields. However, the existing DNM suffers from high complexity and limited generalisation capability. To address these issues, a DNM pruning method with dendrite layer significance constraints is proposed. This method not only evaluates the significance of dendrite layers but also allocates the significance of a few dendrite layers in the trained model to a few dendrite layers, allowing the removal of low-significance dendrite layers. The simulation experiments on six UCI datasets demonstrate that our method surpasses existing pruning methods in terms of network size and generalisation performance.
更多查看译文
关键词
dendritic neuron model,dendrite layer significance constraints
AI 理解论文
溯源树
样例
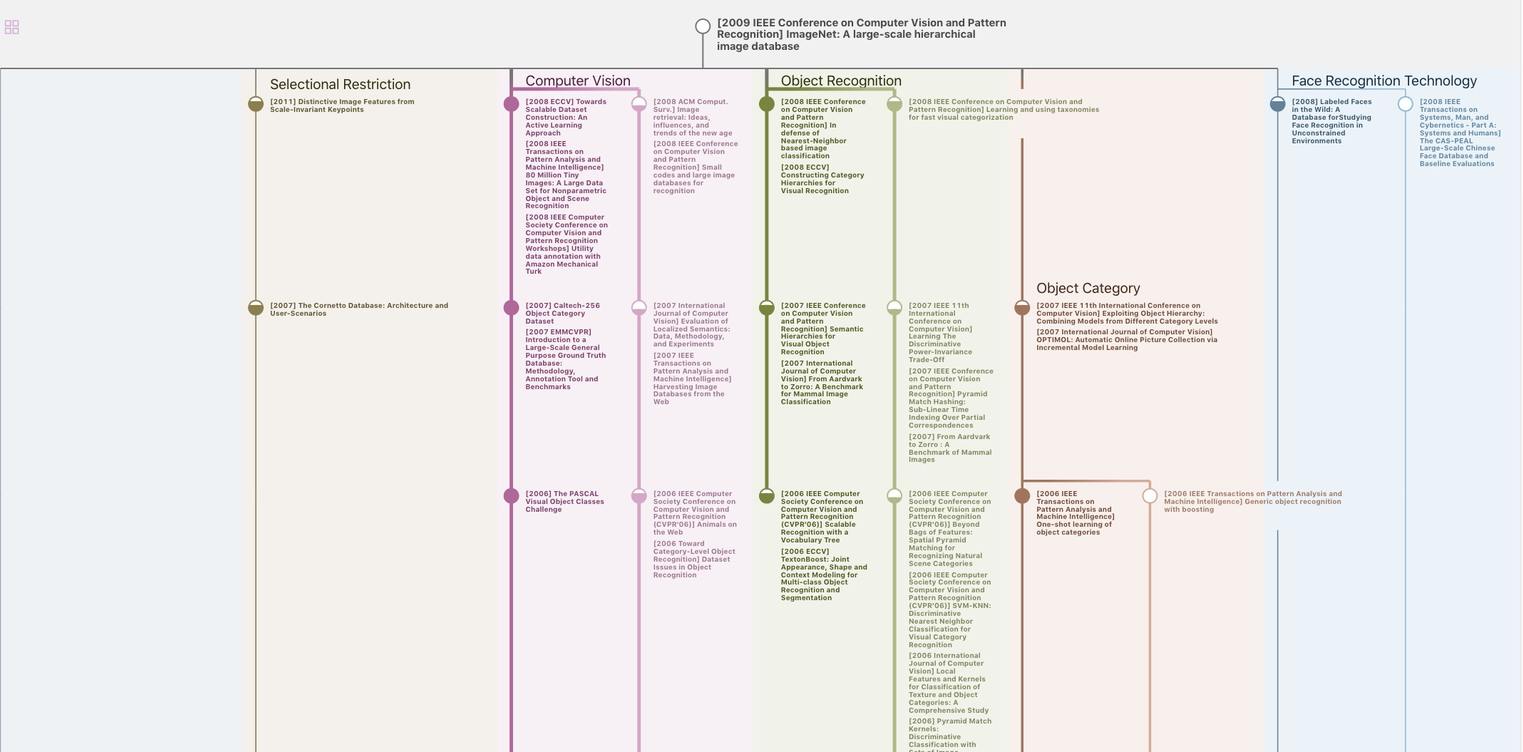
生成溯源树,研究论文发展脉络
Chat Paper
正在生成论文摘要