Overcoming the Long Horizon Barrier for Sample-Efficient Reinforcement Learning with Latent Low-Rank Structure.
SIGMETRICS Perform. Evaluation Rev.(2023)
摘要
Reinforcement learning (RL) methods have been increasingly popular in sequential decision making tasks due to its empirical success. However, large state and action spaces in real-world problems modeled as a Markov decision processes (MDPs) limit the use of RL algorithms. Given a finite-horizon MDP with state space S, action space A, and horizon H, one needs Ω |S|A|H3/ε2 samples given a generative model to learn an optimal policy [3], which can be impractical when S and A are large. The above tabular RL framework does not capture the fact that many realworld systems in fact have additional structure that if exploited should improve computational and statistical efficiency. Moreover, [1] empirically verifies that optimal and near-optimal action-value functions (both viewed as |S|-by- |A| matrices) of classical stochastic control tasks have low rank. Thus, the critical question is what are the minimal low rank structural assumptions that allow for computationally and statistically efficient learning.
更多查看译文
关键词
learning,long horizon barrier,sample-efficient,low-rank
AI 理解论文
溯源树
样例
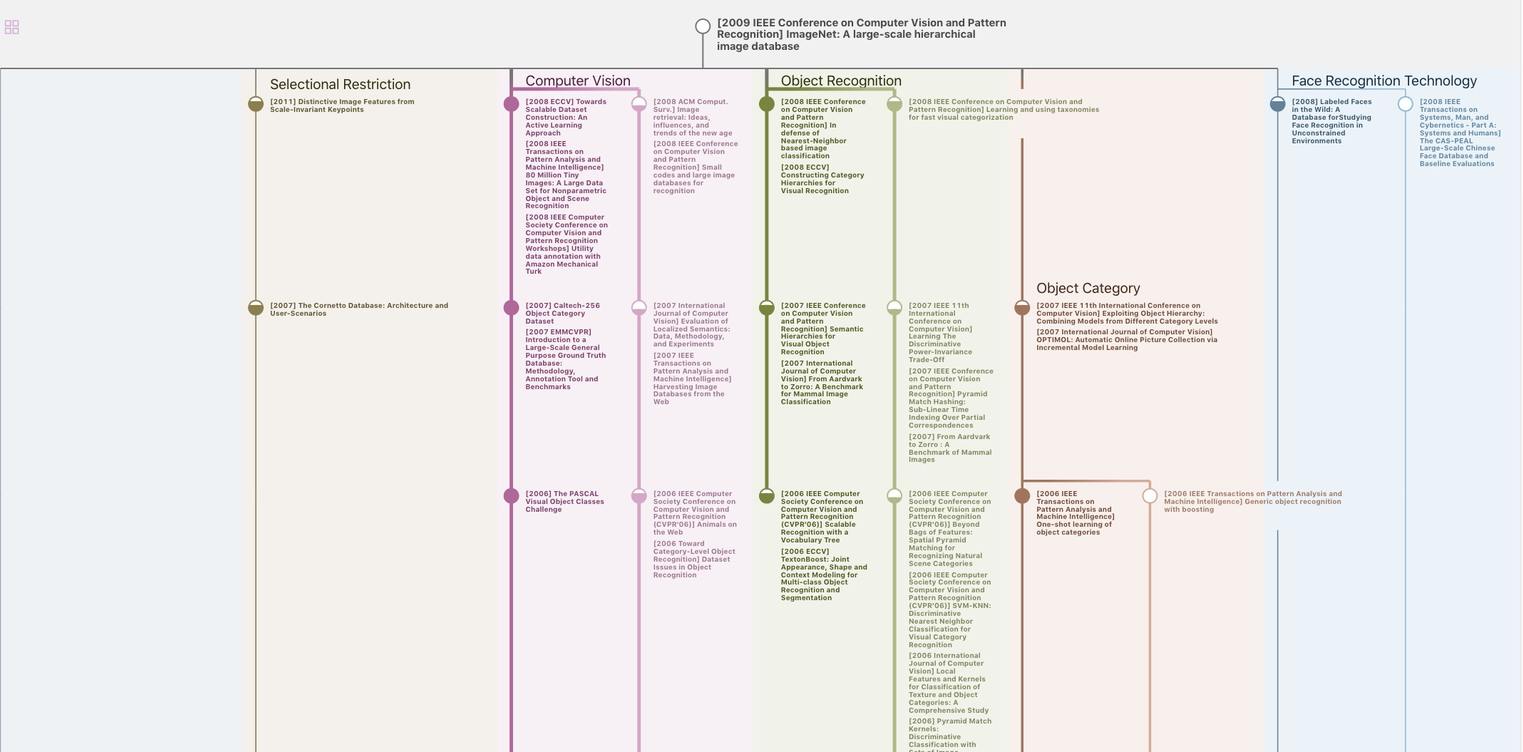
生成溯源树,研究论文发展脉络
Chat Paper
正在生成论文摘要