Robustness and Explainability of Image Classification Based on QCNN.
Quantum Eng.(2023)
摘要
In this paper, we propose a multiscale entanglement renormalization ansatz (MERA) feature extraction method based on a novel quantum convolutional neural network (QCNN) for binary scanning tunneling microscopy (STM) image classification. We design QCNN quantum circuits for state preparation, quantum convolution, and quantum pooling in the TensorFlow quantum framework and compare the performance of QCNN classifier and two hybrid quantum-classical QCNN models. Adversarial attacks are considered as a type of interpretable method to evaluate the robustness of QCNN models. The similarity between the pixels of image bitplane slicing and Ising phase transition opens up new ways for exploring classification performance enhancement by QCNN classifiers. Classification performance of different bitplanes of QCNN also shows that they can robustly resist adversarial attacks such as FGSM, CW, JSMA, and DEEPFOOL.
更多查看译文
关键词
image classification,qcnn,explainability,robustness
AI 理解论文
溯源树
样例
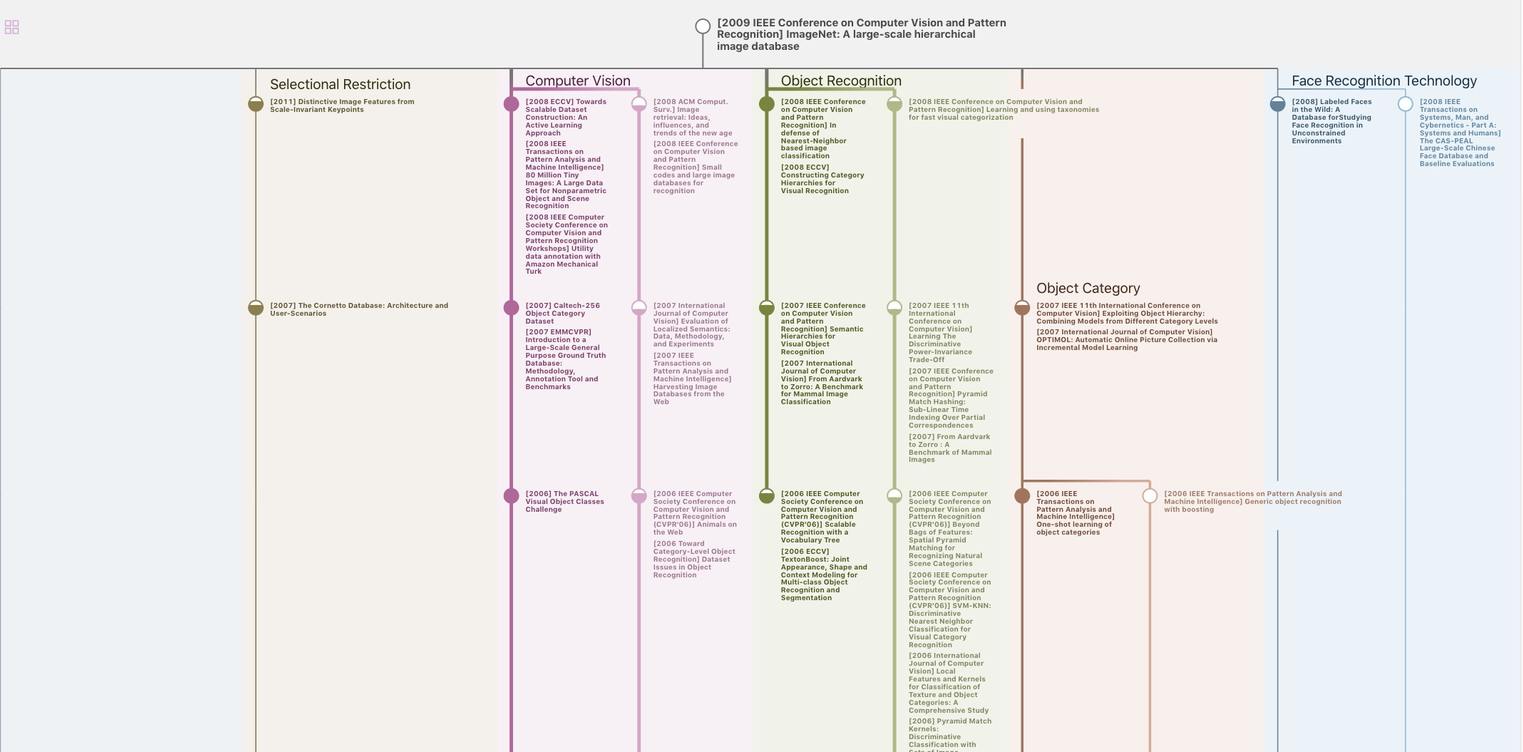
生成溯源树,研究论文发展脉络
Chat Paper
正在生成论文摘要