Learning to Remove Shadows from a Single Image
Int. J. Comput. Vis.(2023)
摘要
Recent learning-based shadow removal methods have achieved remarkable performance. However, they basically require massive paired shadow and shadow-free images for model training, which limits their generalization capability since these data are often cumbersome to obtain and lack of diversity. To address the problem, we present Self-ShadowGAN, a novel adversarial framework that is able to learn to remove shadows in an image by training solely on the image itself, using the shadow mask as the only supervision. Our approach is built upon the concept of histogram matching, by constraining the deshadowed regions produced by a shadow relighting network share similar histograms to the original shadow-free regions via a histogram-based discriminator. In order to speed up the single image training, we define the shadow relighting network to be lightweight multi-layer perceptions (MLPs) that estimate spatially-varying shadow relighting coefficients, where the parameters of the MLPs are predicted from a low-resolution input by a fast convolutional network and then upsampled back to the original full-resolution. Experimental results show that our method performs favorably against the state-of-the-art shadow removal methods, and is effective to process previously challenging shadow images.
更多查看译文
关键词
shadows,single image,learning
AI 理解论文
溯源树
样例
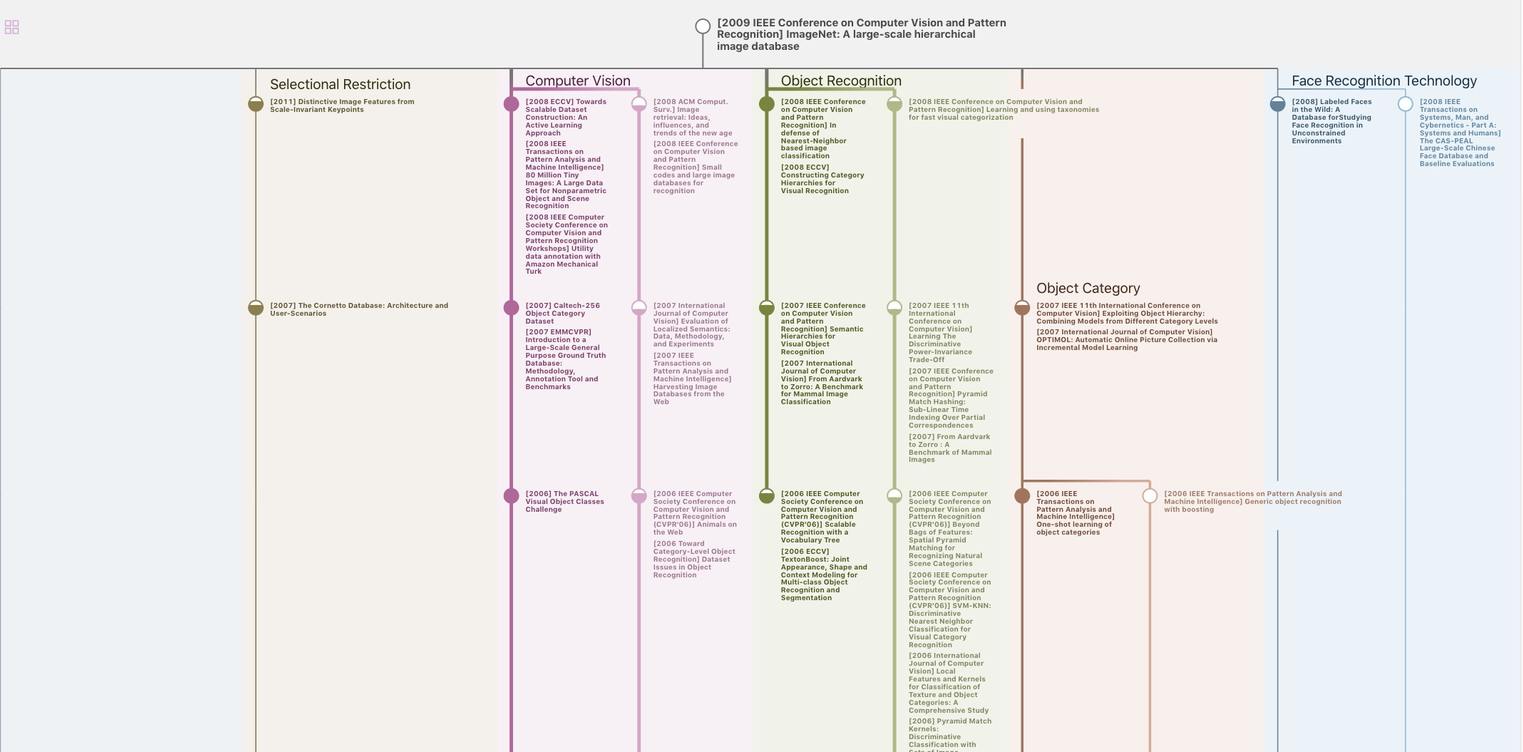
生成溯源树,研究论文发展脉络
Chat Paper
正在生成论文摘要