Feature evolvable learning with image streams.
Intell. Data Anal.(2023)
摘要
Feature Evolvable Stream Learning (FESL) has received extensive attentions during the past few years where old features could vanish and new features could appear when learning with streaming data. Existing FESL algorithms are mainly designed for simple datasets with low-dimension features, nevertheless they are ineffective to deal with complex streams such as image sequences. Such crux lies in two facts: (1) the shallow model, which is supported to be feasible for the low-dimension streams, fails to reveal the complex nonlinear patterns of images, and (2) the linear mapping used to recover the vanished features from the new ones is inadequate to reconstruct the old features of image streams. In response, this paper explores a new online learning paradigm: Feature Evolvable Learning with Image Streams (FELIS) which attempts to make the online learners less restrictive and more applicable. In particular, we present a novel ensemble residual network (ERN), in which the prediction is weighted combination of classifiers learnt by the feature representations from several residual blocks, such that the learning is able to start with a shallow network that enjoys fast convergence, and then gradually switch to a deeper model when more data has been received to learn more complex hypotheses. Moreover, we amend the first residual block of ERN as an autoencoder, and then proposed a latent representation mapping (LRM) approach to exploit the relationship between the previous and current feature space of the image streams via minimizing the discrepancy of the latent representations from the two different feature spaces. We carried out experiments on both virtual and real scenarios over large-scale images, and the experimental results demonstrate the effectiveness of the proposed method.
更多查看译文
关键词
evolvable learning,image streams
AI 理解论文
溯源树
样例
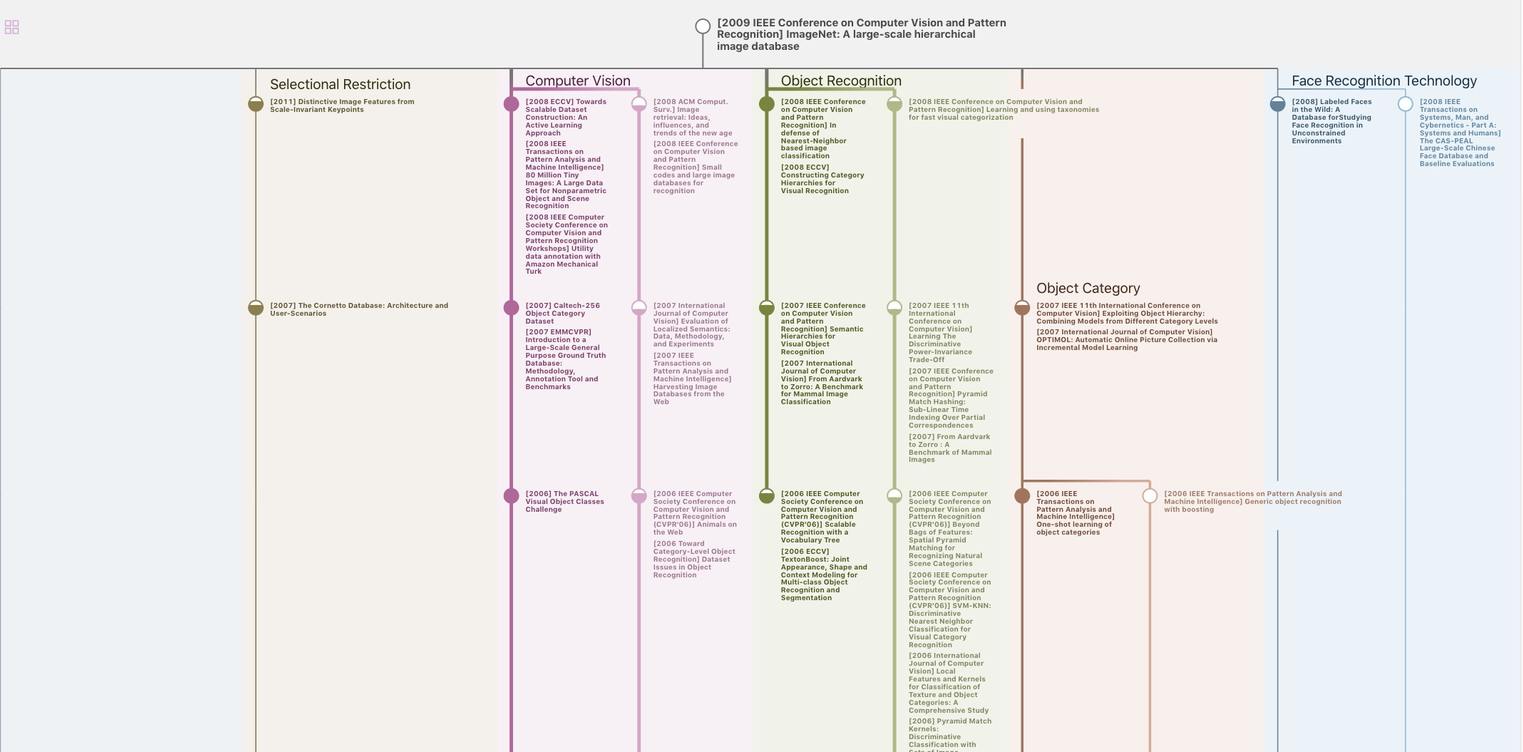
生成溯源树,研究论文发展脉络
Chat Paper
正在生成论文摘要