Data-driven predictive maintenance framework for railway systems.
Intell. Data Anal.(2023)
摘要
The emergence of the Industry 4.0 trend brings automation and data exchange to industrial manufacturing. Using computational systems and IoT devices allows businesses to collect and deal with vast volumes of sensorial and business process data. The growing and proliferation of big data and machine learning technologies enable strategic decisions based on the analyzed data. This study suggests a data-driven predictive maintenance framework for the air production unit (APU) system of a train of Metro do Porto. The proposed method assists in detecting failures and errors in machinery before they reach critical stages. We present an anomaly detection model following an unsupervised approach, combining the Half-Space-trees method with One Class K Nearest Neighbor, adapted to deal with data streams. We evaluate and compare our approach with the Half-Space-Trees method applied without the One Class K Nearest Neighbor combination. Our model produced few type-I errors, significantly increasing the value of precision when compared to the Half-Space-Trees model. Our proposal achieved high anomaly detection performance, predicting most of the catastrophic failures of the APU train system.
更多查看译文
关键词
predictive maintenance framework,railway systems,data-driven
AI 理解论文
溯源树
样例
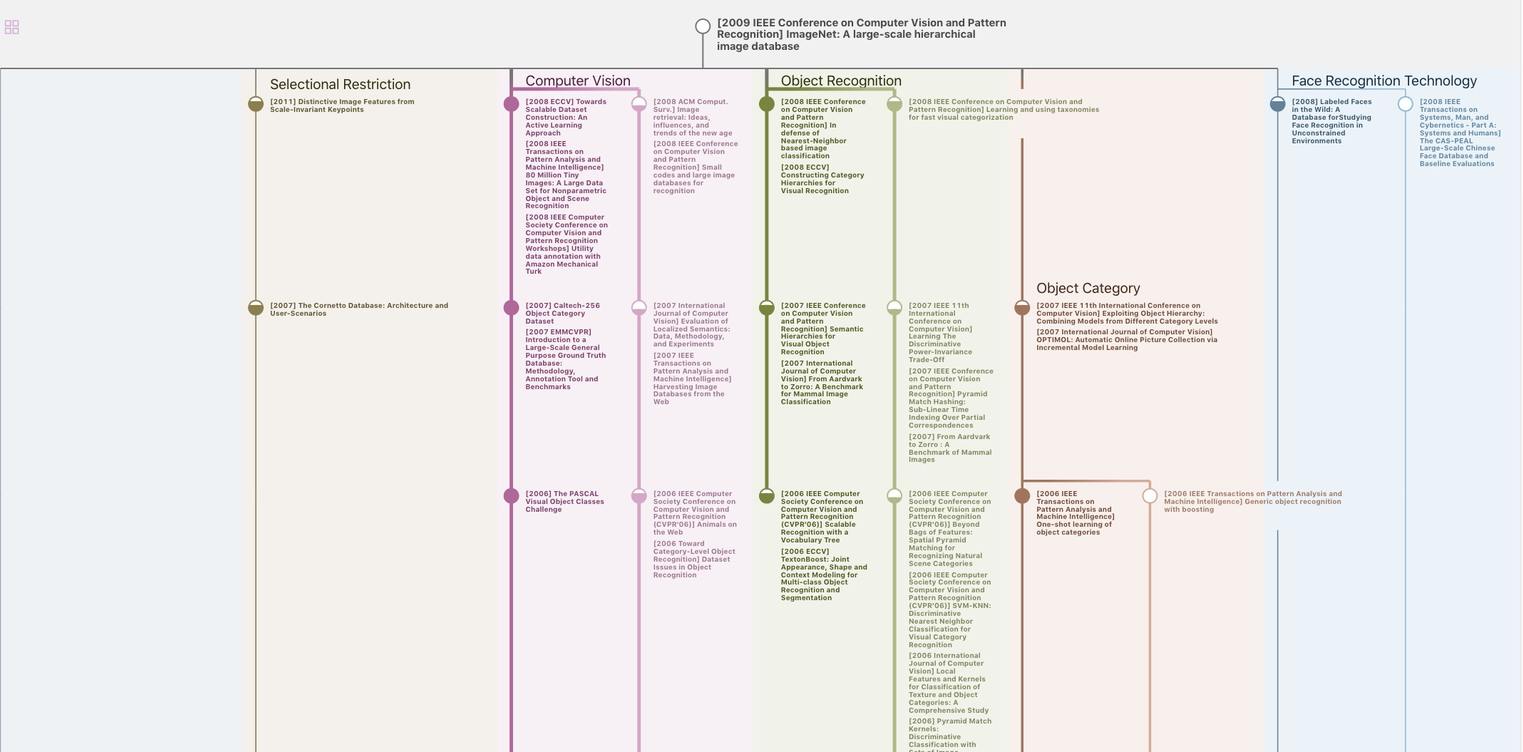
生成溯源树,研究论文发展脉络
Chat Paper
正在生成论文摘要