Dynamic mutual information-based feature selection for multi-label learning.
Intell. Data Anal.(2023)
摘要
In classification problems, feature selection is used to identify important input features to reduce the dimensionality of the input space while improving or maintaining classification performance. Traditional feature selection algorithms are designed to handle single-label learning, but classification problems have recently emerged in multi-label domain. In this study, we propose a novel feature selection algorithm for classifying multi-label data. This proposed method is based on dynamic mutual information, which can handle redundancy among features controlling the input space. We compare the proposed method with some existing problem transformation and algorithm adaptation methods applied to real multi-label datasets using the metrics of multi-label accuracy and hamming loss. The results show that the proposed method demonstrates more stable and better performance for nearly all multi-label datasets.
更多查看译文
关键词
Feature selection, multi-label learning, dynamic mutual information, filter ranking, algorithm adaptation
AI 理解论文
溯源树
样例
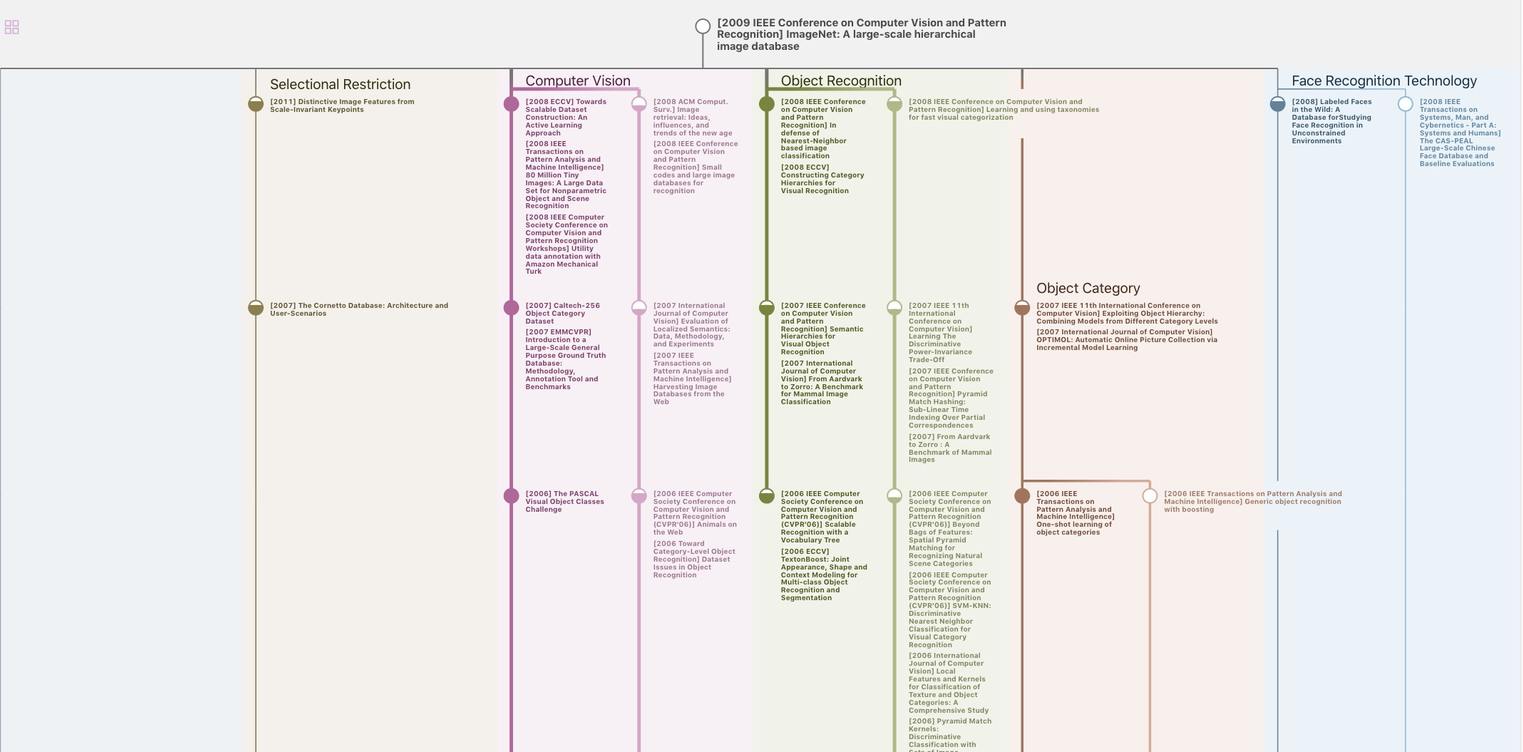
生成溯源树,研究论文发展脉络
Chat Paper
正在生成论文摘要