Unsupervised Deep Unfolded Representation Learning for Singing Voice Separation
IEEE ACM Trans. Audio Speech Lang. Process.(2023)
摘要
Learning effective vocal representations from a waveform mixture is a crucial but challenging task for deep neural network (DNN)-based singing voice separation (SVS). Successful representation learning (RL) depends heavily on well-designed neural architectures and effective general priors. However, DNNs for RL in SVS are mostly built on generic architectures without general priors being systematically considered. To address these issues, we introduce deep unfolding to RL and propose two RL-based models for SVS, deep unfolded representation learning (DURL) and optimal transport DURL (OT-DURL). In both models, we formulate RL as a sequence of optimization problems for signal reconstruction, where three general priors, synthesis, non-negative, and our novel analysis, are incorporated. In DURL and OT-DURL, we take different approaches in penalizing the analysis prior. DURL uses the Euclidean distance as its penalty, while OT-DURL uses a more sophisticated penalty known as the OT distance. We address the optimization problems in DURL and OT-DURL with the first-order operator splitting algorithm and unfold the obtained iterative algorithms to novel encoders, by mapping the synthesis/analysis/non-negative priors to different interpretable sublayers of the encoders. We evaluated these DURL and OT-DURL encoders in the unsupervised informed SVS and supervised Open-Unmix frameworks. Experimental results indicate that (1) the OT-DURL encoder is better than the DURL encoder and (2) both encoders can considerably improve the vocal-signal-separation performance compared with those of the baseline model.
更多查看译文
关键词
Deep unfolding,representation learning,analysis prior,singing voice separation
AI 理解论文
溯源树
样例
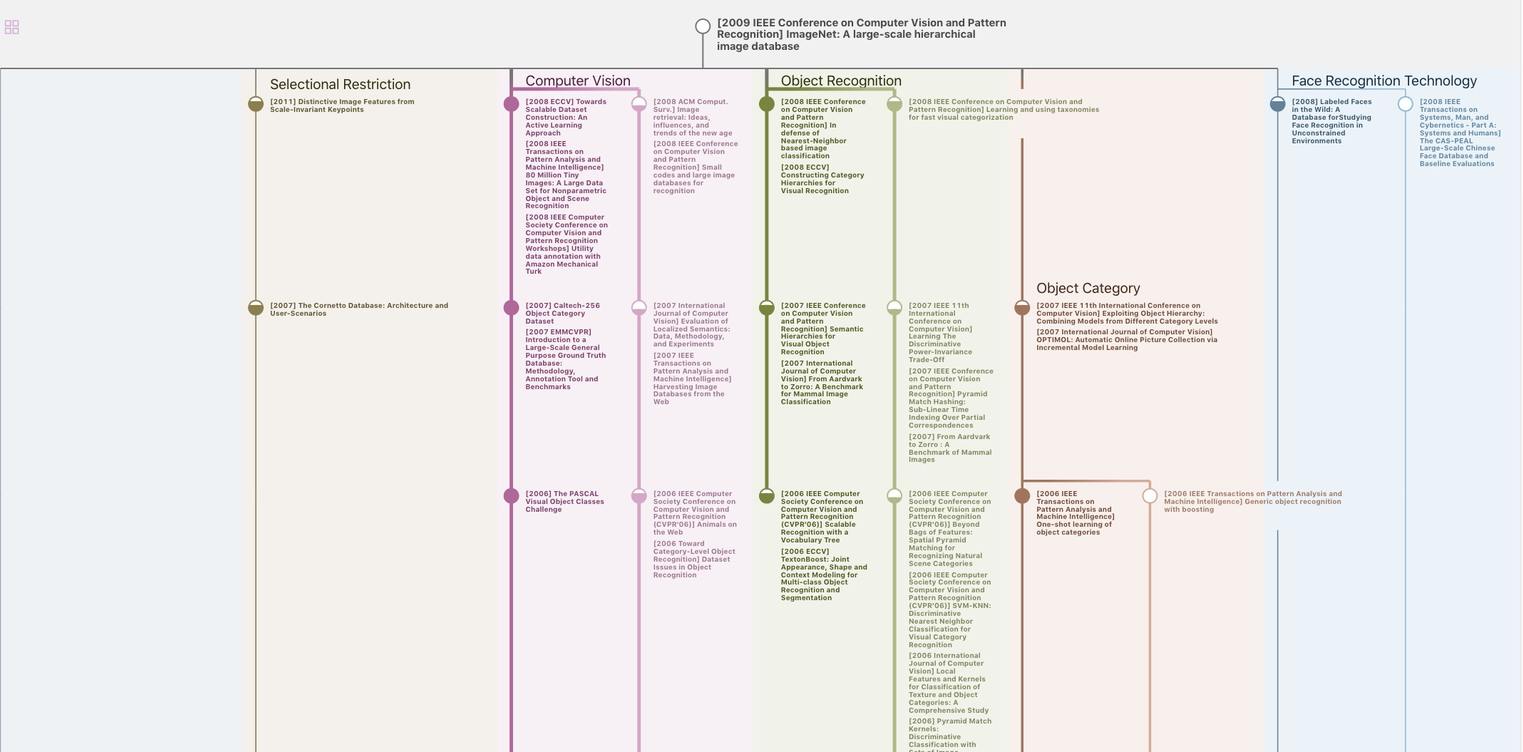
生成溯源树,研究论文发展脉络
Chat Paper
正在生成论文摘要