Reinforcement-Learning Based Method for Accelerating Functional Coverage Closure of Traffic Light Controller Dynamic Digital Design.
ICCTA(2022)
摘要
Functional verification of digital designs is an increasingly complex and time-consuming endeavor. One of the major challenges in functional verification is achieving functional coverage closure in a timely manner. Verification experts have moved from using directed stimulus, towards constrained random stimulus and finally, towards using machine learning to direct stimulus. In this work, reinforcement learning (RL) was used, incorporating three different kinds of RL agents to direct stimulus generation for a traffic light controller system. Two of the agents are based on Q-learning (DQN and SARSA), and one is based on Monte-Carlo method (CEM). The maximum reduction in simulation time needed to achieve a targeted percent of coverage was 97%. The average reduction of simulation time across all testcases and agents used was 70%. The solution is highly reusable, the architecture was integrated with different agents and testcases.
更多查看译文
关键词
Functional Verification,Functional Coverage Closure,Reinforcement Learning,Digital Design,RTL,FSM
AI 理解论文
溯源树
样例
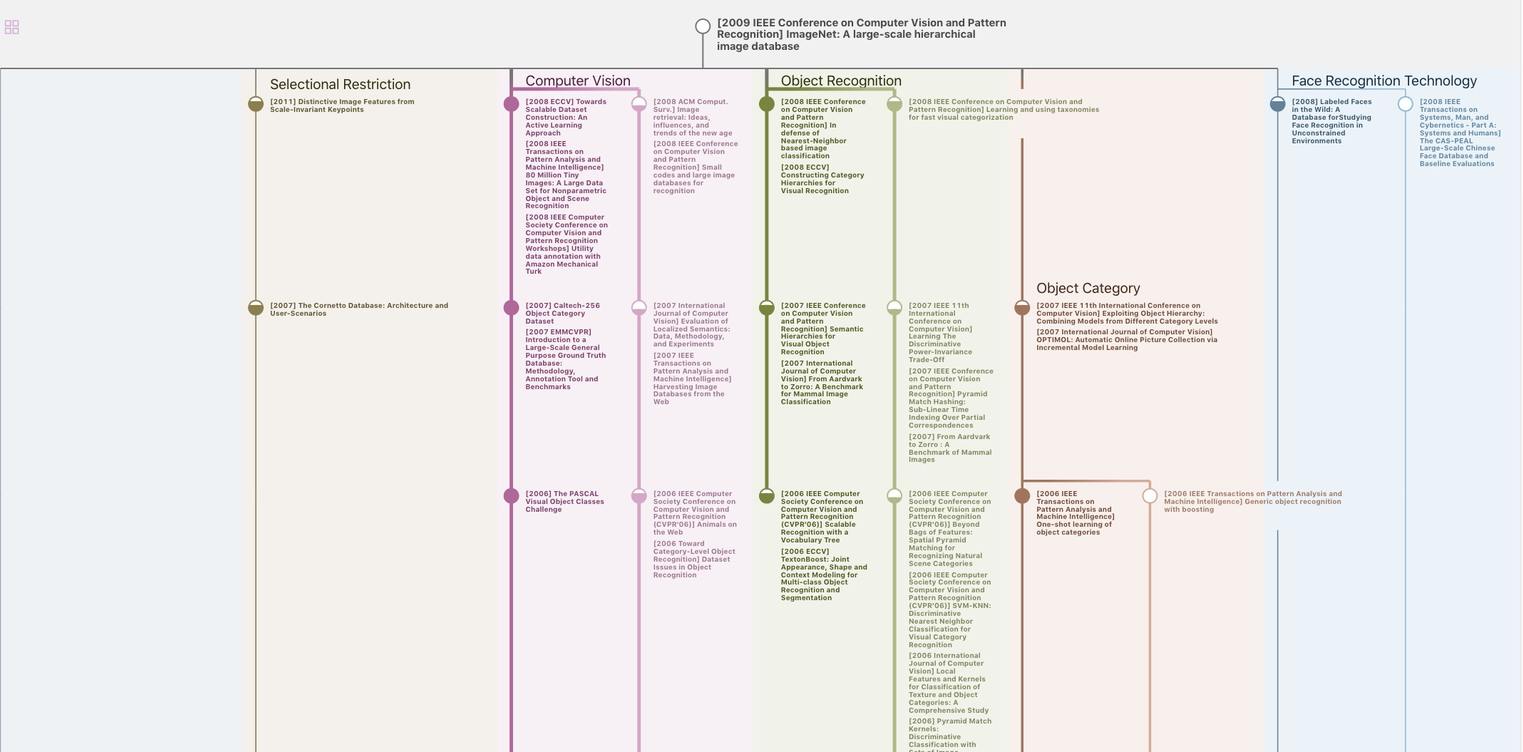
生成溯源树,研究论文发展脉络
Chat Paper
正在生成论文摘要