Susceptibility of Autonomous Driving Agents to Learning-Based Action-Space Attacks
DSN-W(2023)
摘要
Intelligent vehicles with increasing complexity face cybersecurity threats. This paper studies action-space attacks on autonomous driving agents that make decisions using either a traditional modular processing pipeline or the recently proposed end-to-end driving model obtained via deep reinforcement learning (DRL). Such attacks alter the actuation signal and pose direct risks to the vehicle's state. We formulate the attack construction as a DRL problem based on the input from either an extra camera or inertial measurement unit deployed. The attacks are designed to lurk until a safety-critical moment arises and cause a side collision upon activation. We analyze the behavioral differences between two driving agents when subjected to action-space attacks and demonstrate the superior resilience of the modular processing pipeline. We further investigate the performance and limitations of two enhancement methods, i.e., adversarial training through fine-tuning and progressive neural networks. The result offers valuable insights into vehicle safety from the viewpoints of both the assailant and the defender and informs the future design of autonomous driving systems.
更多查看译文
关键词
Action-space attack,Autonomous driving,Reinforcement learning,Cybersecurity
AI 理解论文
溯源树
样例
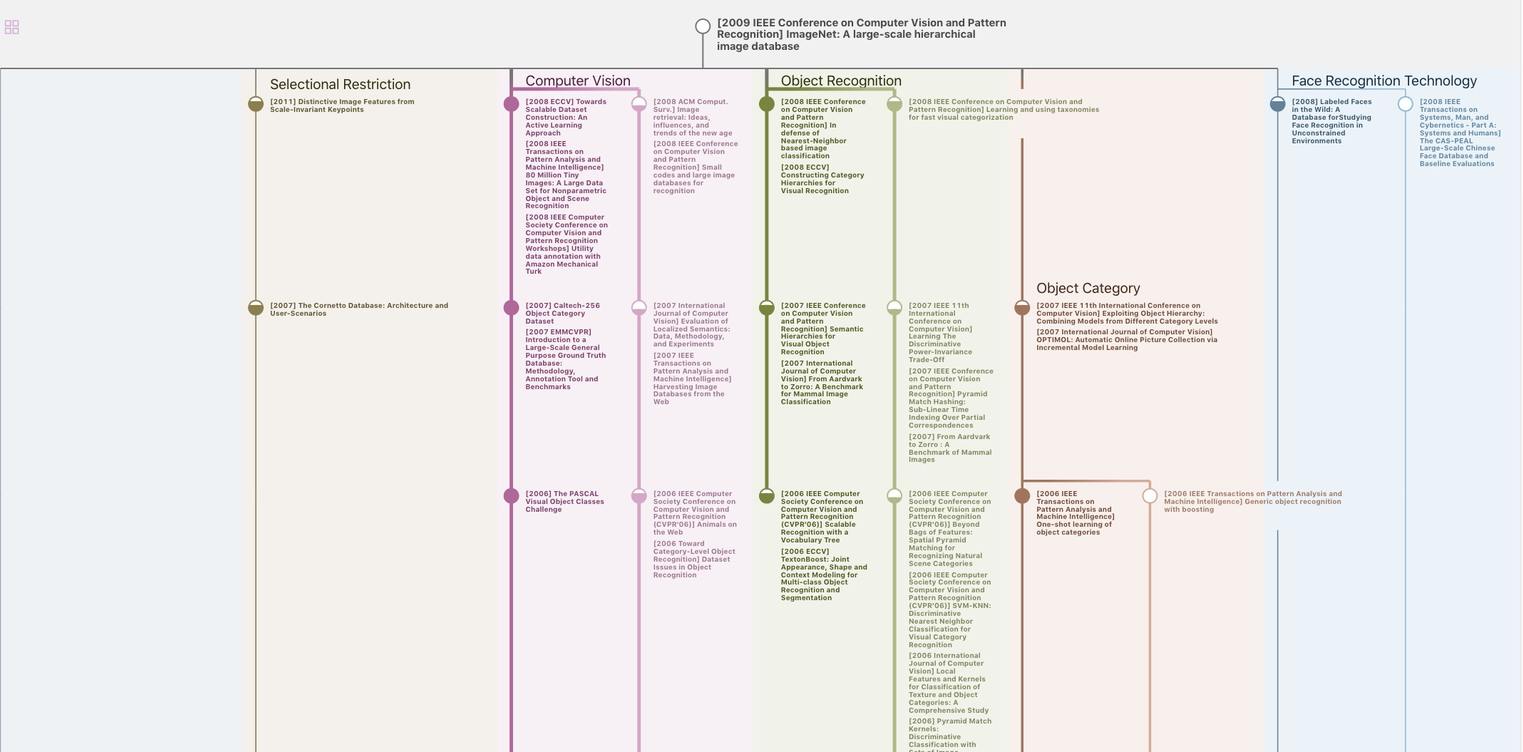
生成溯源树,研究论文发展脉络
Chat Paper
正在生成论文摘要