Continuous Kendall Shape Variational Autoencoders.
GSI (1)(2023)
摘要
We present an approach for unsupervised learning of geometrically meaningful representations via equivariant variational autoencoders (VAEs) with hyperspherical latent representations. The equivariant encoder/decoder ensures that these latents are geometrically meaningful and grounded in the input space. Mapping these geometry- grounded latents to hyperspheres allows us to interpret them as points in a Kendall shape space. This paper extends the recent Kendall-shape VAE paradigm by Vadgama et al. by providing a general definition of Kendall shapes in terms of group representations to allow for more flexible modeling of KS-VAEs. We show that learning with generalized Kendall shapes, instead of landmark-based shapes, improves representation capacity.
更多查看译文
AI 理解论文
溯源树
样例
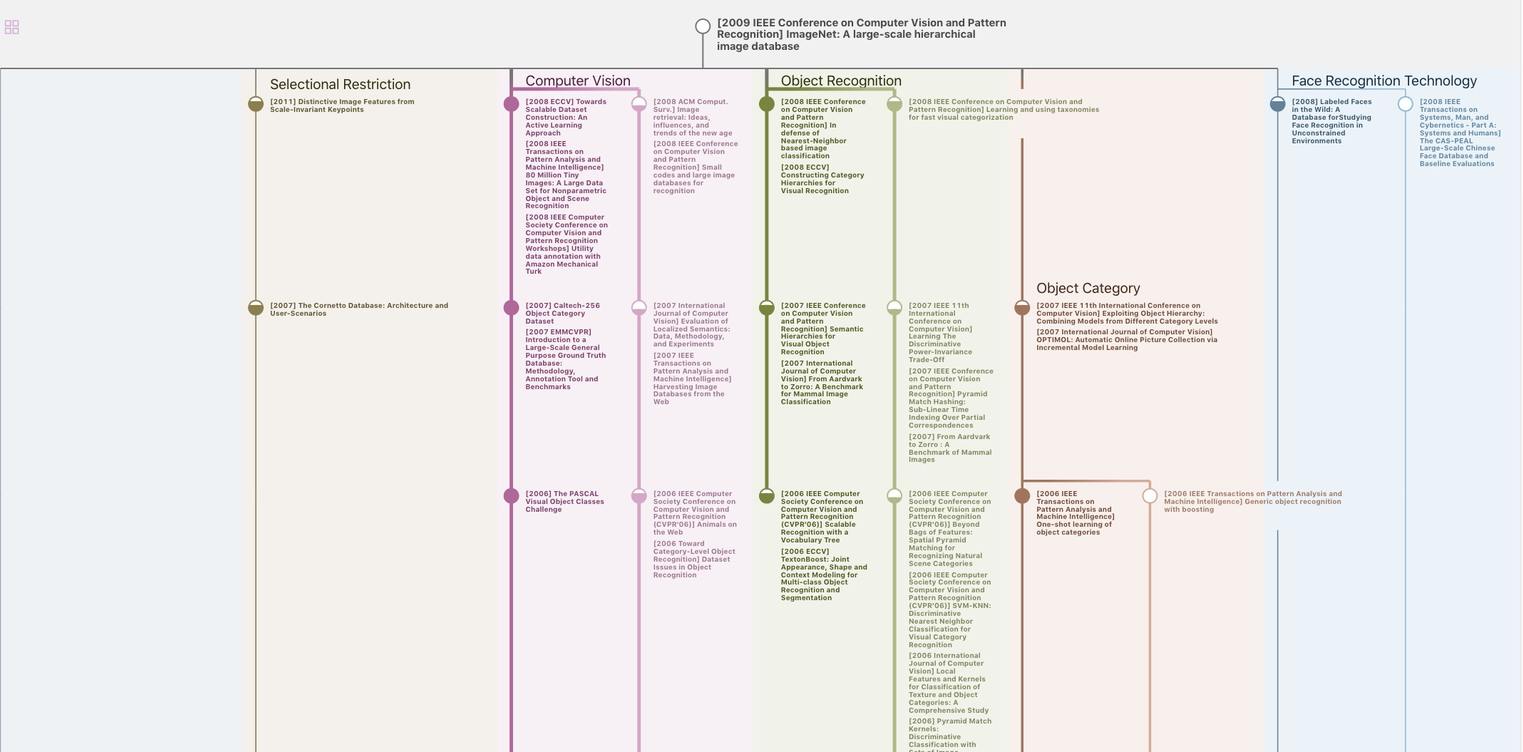
生成溯源树,研究论文发展脉络
Chat Paper
正在生成论文摘要