TAPE-Pero: Using Deep Representation Learning Model to Identify and Localize Peroxisomal Proteins.
ICIC (3)(2023)
摘要
Peroxisomes, organelles containing one or more oxidases within a single lipid bilayer, play a crucial role in various metabolic pathways. Incorrect localization of peroxisomal proteins can lead to severe diseases. In this study, we introduced the TAPE-Pero model to improve the accuracy of peroxisomal protein identification and localization. This model incorporates two deep representation learning models, ProSE and BERT-based TAPE, trained on large protein databases, to extract peroxisomal protein features. The data set is balanced using SMOTE, and the optimal feature vector is selected using analysis of variance (ANOVA). Subsequently, nine machine learning classifiers are utilized to identify and locate peroxisomal proteins. Our model outperforms existing state-of-the-art methods with an overall prediction accuracy of 98.97% and 92.57% from tenfold cross-validation and double cross-validation tests on the data set, respectively. The proposed model provides a novel approach for the identification and localization of peroxisomal proteins.
更多查看译文
关键词
localize peroxisomal proteins,deep representation learning model,tape-pero
AI 理解论文
溯源树
样例
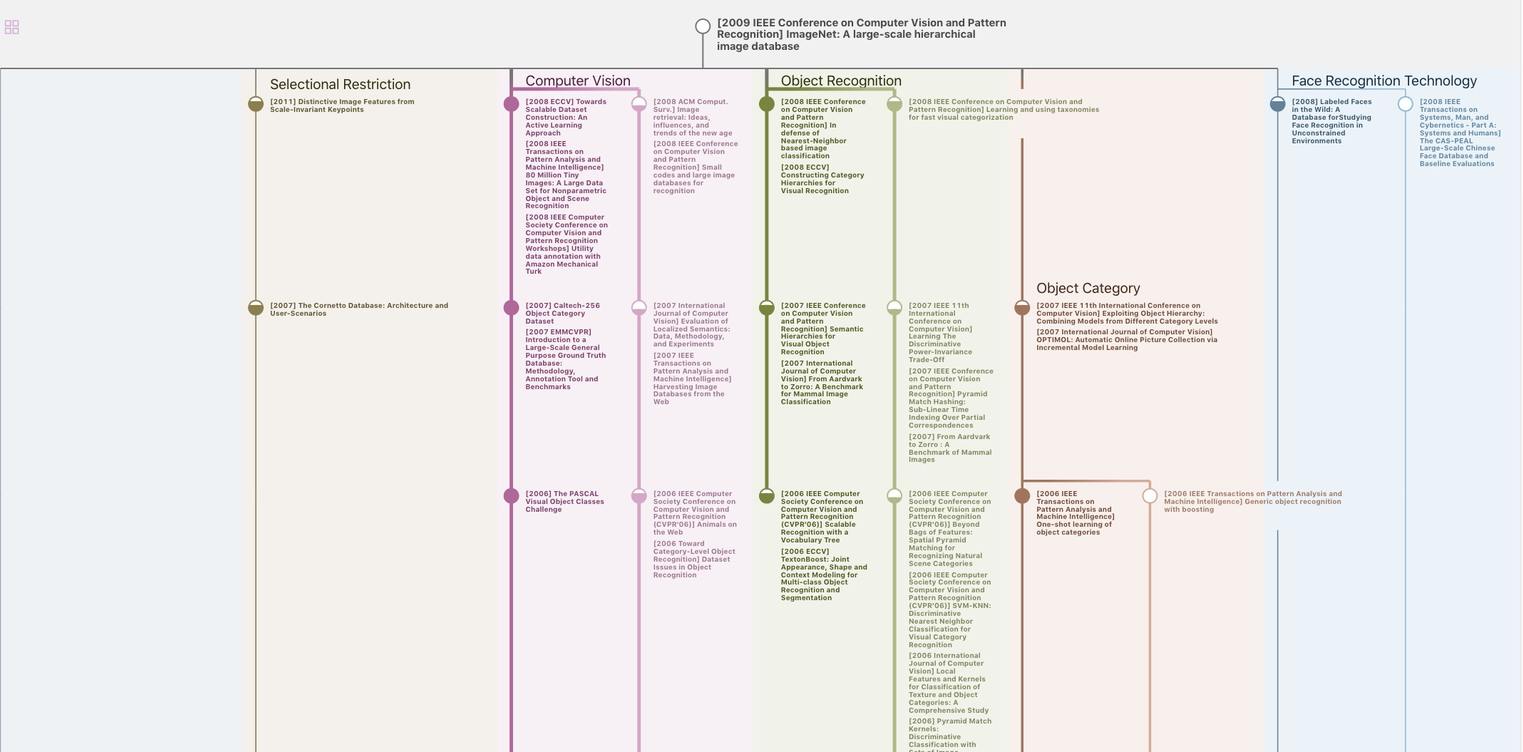
生成溯源树,研究论文发展脉络
Chat Paper
正在生成论文摘要