Hessian Non-negative Hypergraph.
ICIC (5)(2023)
摘要
Hypergraph is a vital relationship modeling tool applied to many learning tasks. Compared with the common graph, the hypergraph can model the high-order relationship among more than two data samples. Thus, hypergraph provides more flexibility and the capability of modeling complex data correlations. However, real-world image data inevitably lie on or close to a thin layer of a manifold while containing the noise component. The practical image data influence the hypergraph learning procedure, subsequently resulting in a notable performance deterioration. To this end, we propose a novel Hessian non-negative hypergraph model. Specifically, the Hessian energy regularized non-negative constrained data reconstruction is used to generate manifold-respecting and noise resistant hyperedges. The extensive experiments on the benchmark image datasets demonstrate that the proposed hypergraph model can significantly enhance the learning performance of the hypergraph-based image clustering and classification.
更多查看译文
关键词
non-negative
AI 理解论文
溯源树
样例
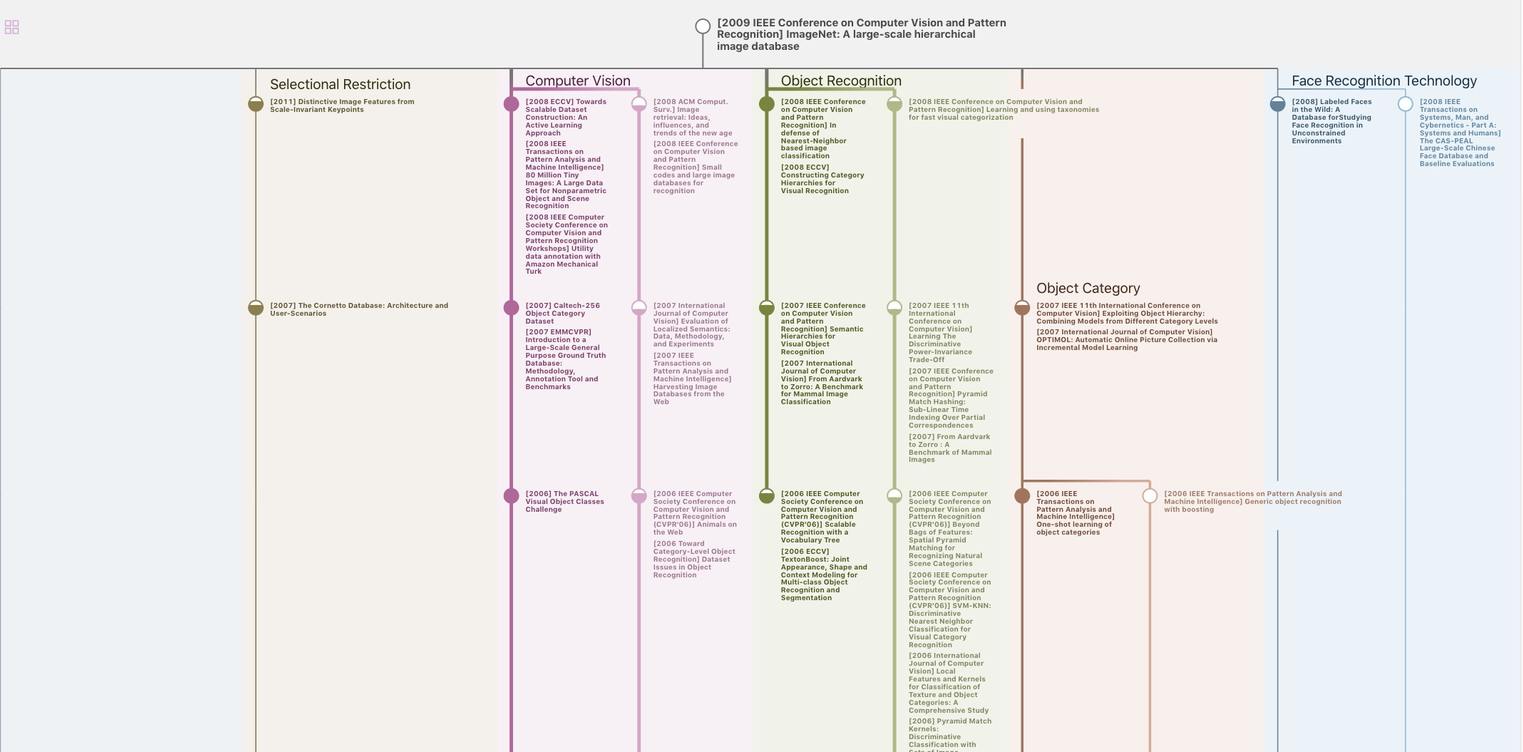
生成溯源树,研究论文发展脉络
Chat Paper
正在生成论文摘要