Graph-Based Short Text Clustering via Contrastive Learning with Graph Embedding.
ICIC (1)(2023)
摘要
Clustering is an unsupervised learning technique that helps us quickly classify short texts. It works by effectively capturing the semantic themes of texts and assigning the similar texts into the same cluster. Due to the excellent ability of contrastive learning to learn representations, using contrastive learning to extract semantic features for clustering tasks has become a new trend for short text clustering. However, the existing short text clustering methods pay more attention to the global information, and lead to wrong classification for samples with ambiguous clusters. Therefore, we propose graph-based short text clustering via contrastive learning with graph embedding (GCCL) - a novel framework that leverages the affinity between samples and neighbors to impose constraints on the low-dimensional representation space. To verify the effectiveness of our method, we evaluate GCCL on short text benchmark datasets. The experimental results show that GCCL outperforms the baseline method in terms of accuracy (ACC) and normalized mutual information (NMI). In addition, our approach achieves impressive results in terms of convergence speed, demonstrating the guidance of graph embeddings for short text clustering tasks.
更多查看译文
关键词
short text clustering,contrastive learning,graph-based
AI 理解论文
溯源树
样例
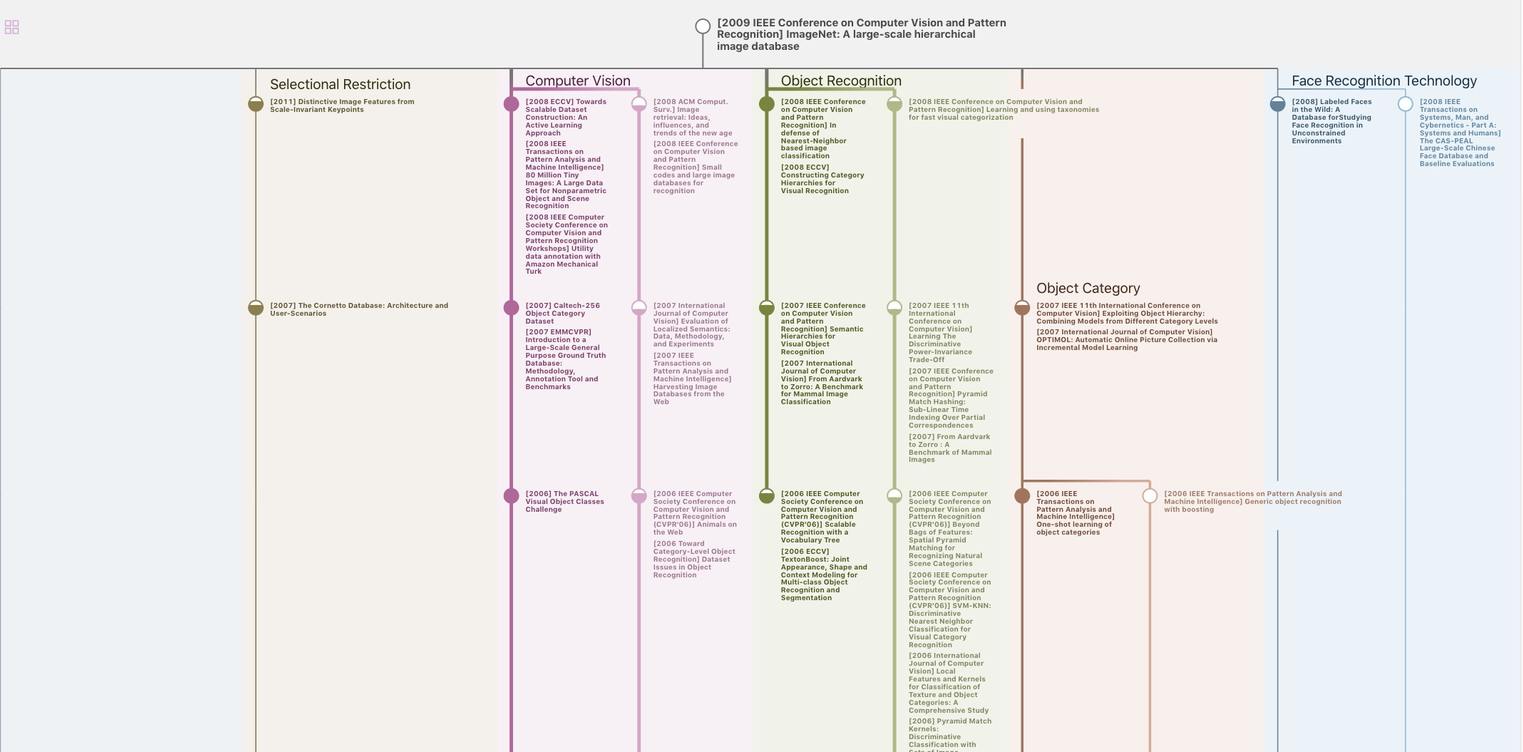
生成溯源树,研究论文发展脉络
Chat Paper
正在生成论文摘要