Leveraging Inter-class Differences and Label Semantics for Few-Shot Text Classification.
ICIC (4)(2023)
摘要
In some few-shot text classification tasks with strong data privacy or difficult labeling, the performance of pipeline methods, which directly encode text features and perform linear classification, is limited by the feature extraction ability of models. An increasing number of studies have recognized the significance of combining text features with label semantics and achieved good results. However, these existing methods cannot be well generalized to classification tasks where the class names have weak correlations with the instance texts. In this work, we address this problem by means of an effective fusion of text-label similarity and a redesign of contrastive loss. Firstly, the semantic similarity modules of text-text and text-label are adopted for further merging to improve the feature extraction ability. Then, we introduce DLSC, an inter-class differences and label semantics contrastive loss that facilitates instance embeddings to approximate correct label semantics in vector space. Experimental results show that our approach has greatly improved F1 scores on English and Chinese datasets from six classification tasks, even in tasks where label names are not strongly correlated with texts.
更多查看译文
关键词
text classification,label semantics,inter-class,few-shot
AI 理解论文
溯源树
样例
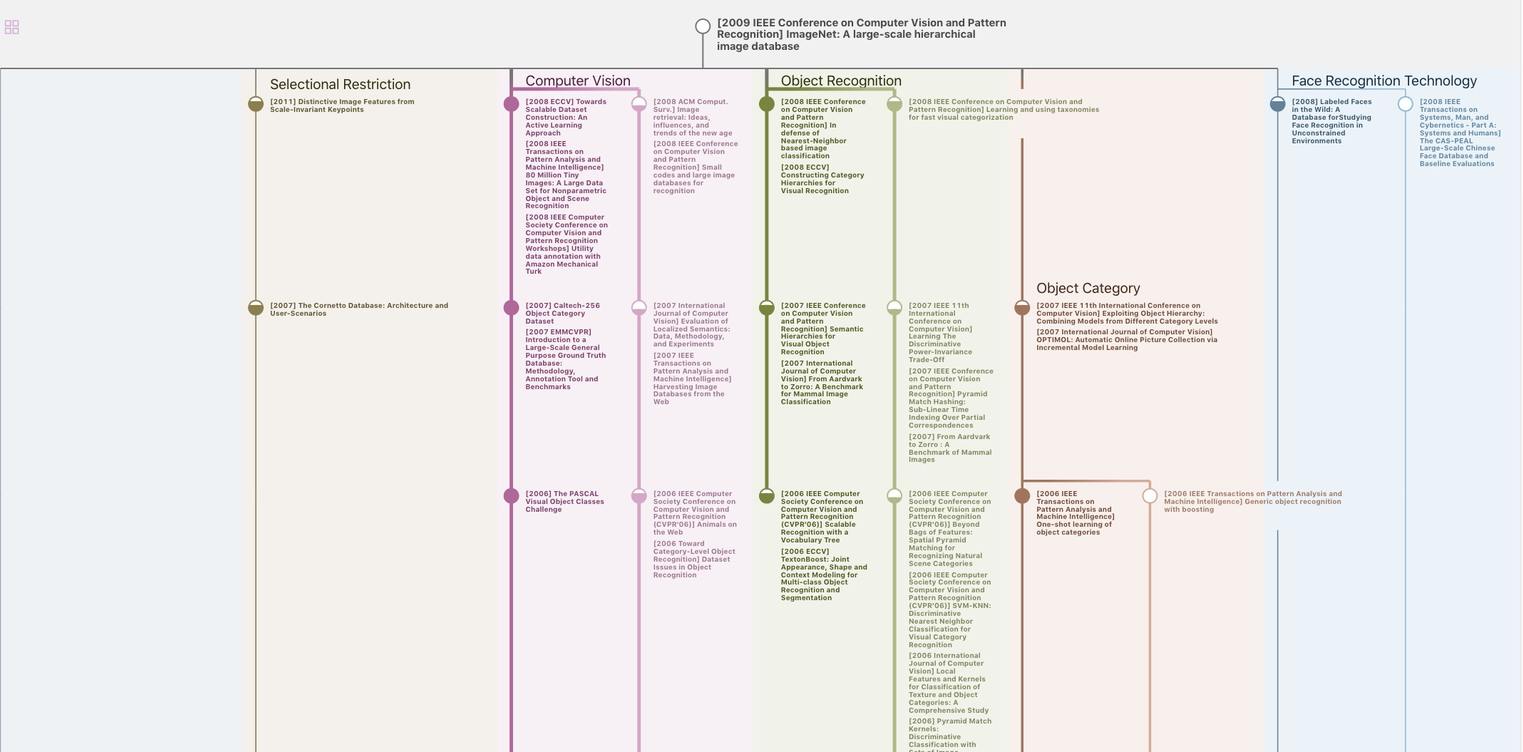
生成溯源树,研究论文发展脉络
Chat Paper
正在生成论文摘要