Learning Variable Neighborhood Search Algorithm for Solving the Energy-Efficient Flexible Job-Shop Scheduling Problem.
ICIC (1)(2023)
摘要
With the increasingly challenging environment, green manufacturing attracts widespread attention. Moreover, facing fierce market competition and frequently variable product demand, flexible manufacturing is more closely to real-life applications. In this article, we propose a probability matrix-based learning variable neighborhood local search (LVNS_PM) algorithm for solving the energy-efficient flexible job-shop scheduling problem (EE_FJSP). The optimization objective is to minimize the makespan and total energy consumption (TEC). Concretely speaking, on the one hand, a problem-dependent three-dimensional probability matrix is proposed as an information accumulation matrix to learn and retain characteristic messages of elite solutions that guide the algorithm’s global exploration. On the other hand, we design multiple problem-specific neighborhood operators and present a variable neighborhood search algorithm, which balances the algorithm’s global exploration and local exploitation. Finally, simulation studies and computational comparisons demonstrate the practicality and robustness of LVNS_PM.
更多查看译文
关键词
job-shop job-shop scheduling problem,variable neighborhood search algorithm,energy-efficient
AI 理解论文
溯源树
样例
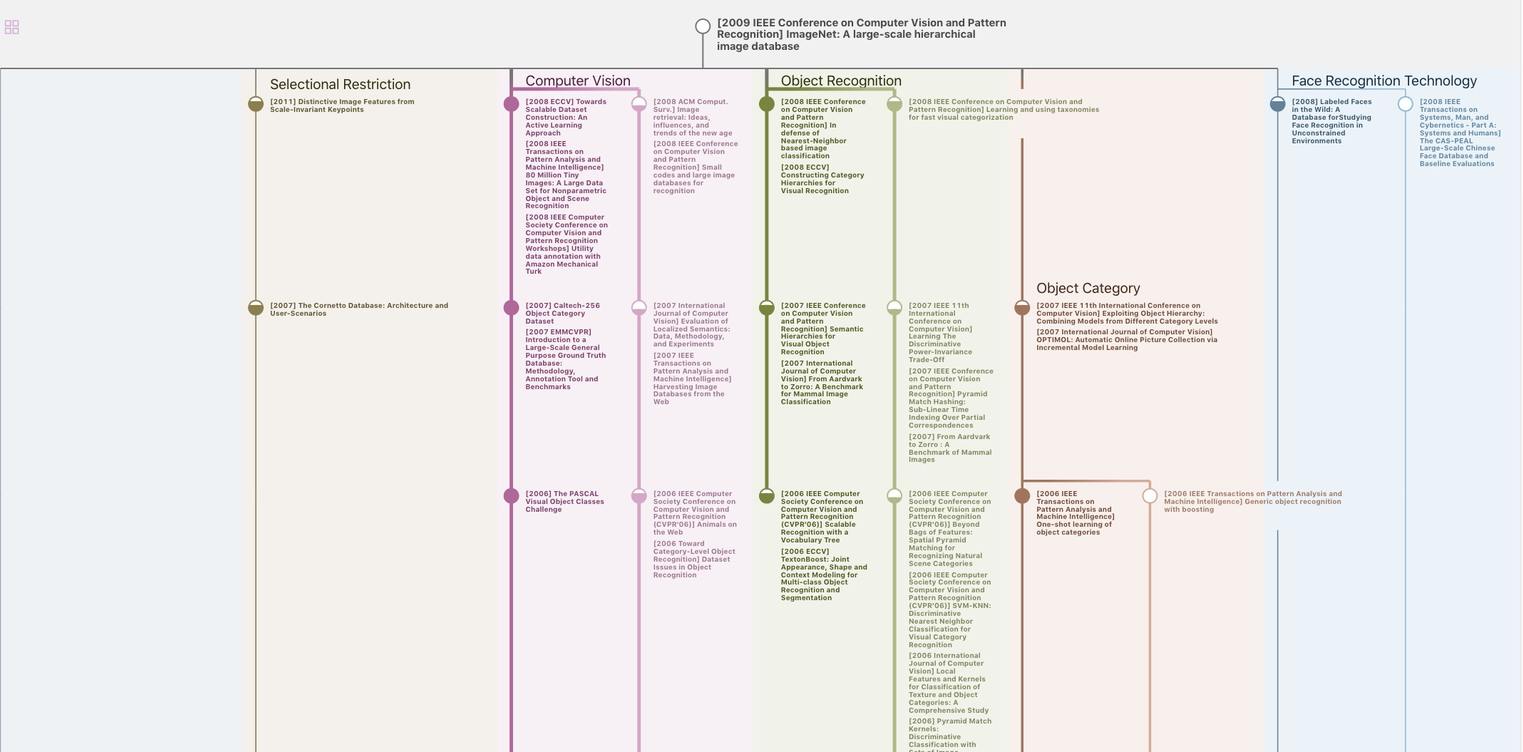
生成溯源树,研究论文发展脉络
Chat Paper
正在生成论文摘要