On Distributed Training of Foundation Models: Challenges and Observations.
IPDPS Workshops(2023)
摘要
We are currently at an inflection point in the ongoing AI revolution. The emergence of highly parallelizable transformer-based neural architectures, along with self-supervised learning, has made it possible to use widely available unlabeled datasets to train large foundation models. These models have shown remarkable performance across various benchmarks and continue to exhibit new and improved emergent properties as we scale across parameter and data sizes. Over a year ago, our team at IBM Research embarked on a mission to perform distributed training of these large-scale foundation models. We aimed to do so on cost-effective commodity hardware that is cloud native. In this presentation, we will discuss the challenges we faced - from selecting model architectures and hyper-parameters to parallelization choices for distributed training at scale - and the valuable lessons we learned.
更多查看译文
关键词
cost effective commodity hardware,data sizes,distributed training,highly parallelizable transformer-based neural architectures,IBM Research,improved emergent properties,inflection point,large scale foundation models,model architectures,ongoing AI revolution,self-supervised learning,widely available unlabeled datasets
AI 理解论文
溯源树
样例
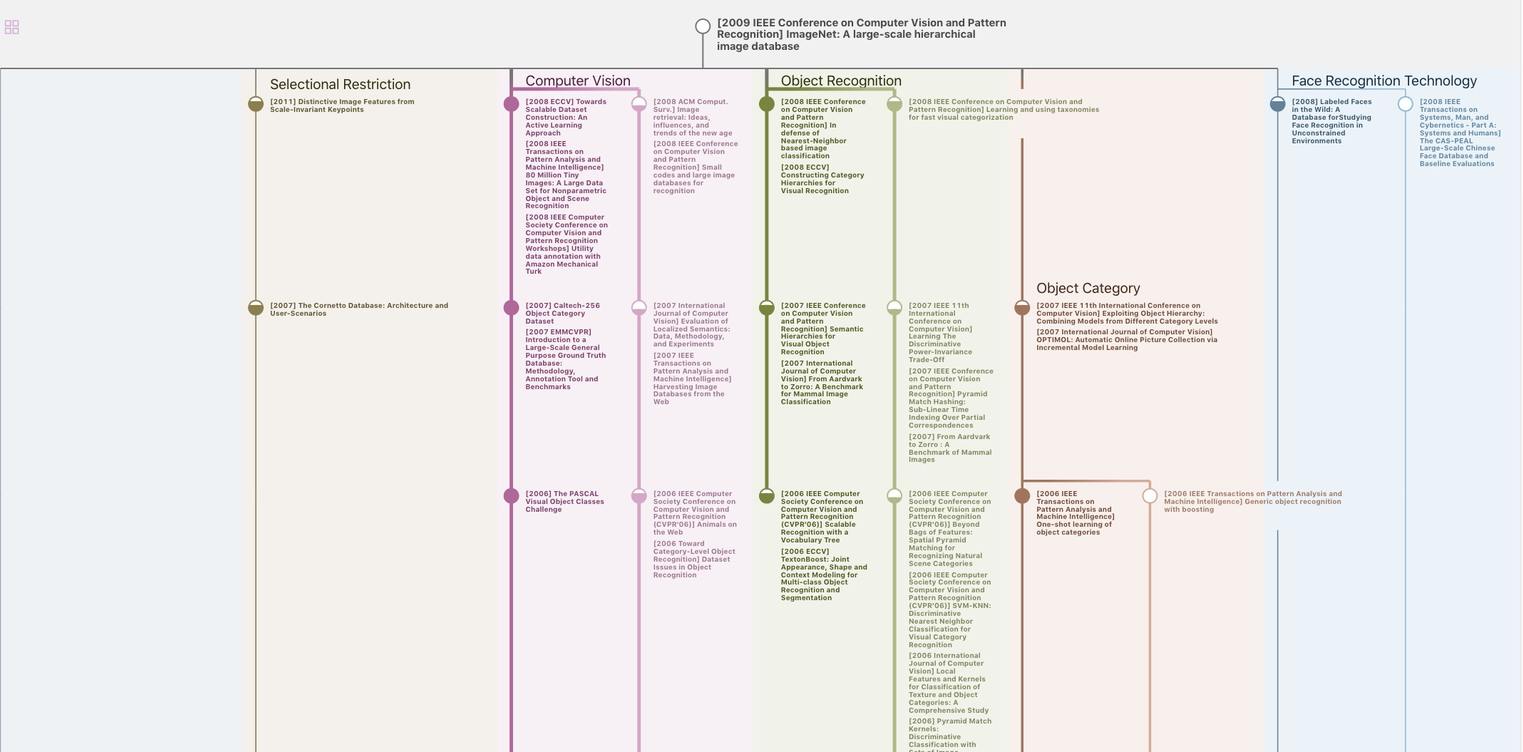
生成溯源树,研究论文发展脉络
Chat Paper
正在生成论文摘要