Comparing and Improving Active Learning Uncertainty Measures for Transformer Models.
ADBIS(2023)
摘要
Despite achieving state-of-the-art results in nearly all Natural Language Processing applications, fine-tuning Transformer-encoder based language models still requires a significant amount of labeled data to achieve satisfying work. A well known technique to reduce the amount of human effort in acquiring a labeled dataset is Active Learning (AL): an iterative process in which only the minimal amount of samples is labeled. AL strategies require access to a quantified confidence measure of the model predictions. A common choice is the softmax activation function for the final Neural Network layer. In this paper we compare eight alternatives on seven datasets and show that the softmax function provides misleading probabilities. Our finding is that most of the methods primarily identify hard-to-learn-from samples (outliers), resulting in worse than random performance, instead of samples, which reduce the uncertainty of the learned language model. As a solution this paper proposes a heuristic to systematically exclude samples, which results in improvements of various methods compared to the softmax function.
更多查看译文
关键词
active learning uncertainty measures,transformer models
AI 理解论文
溯源树
样例
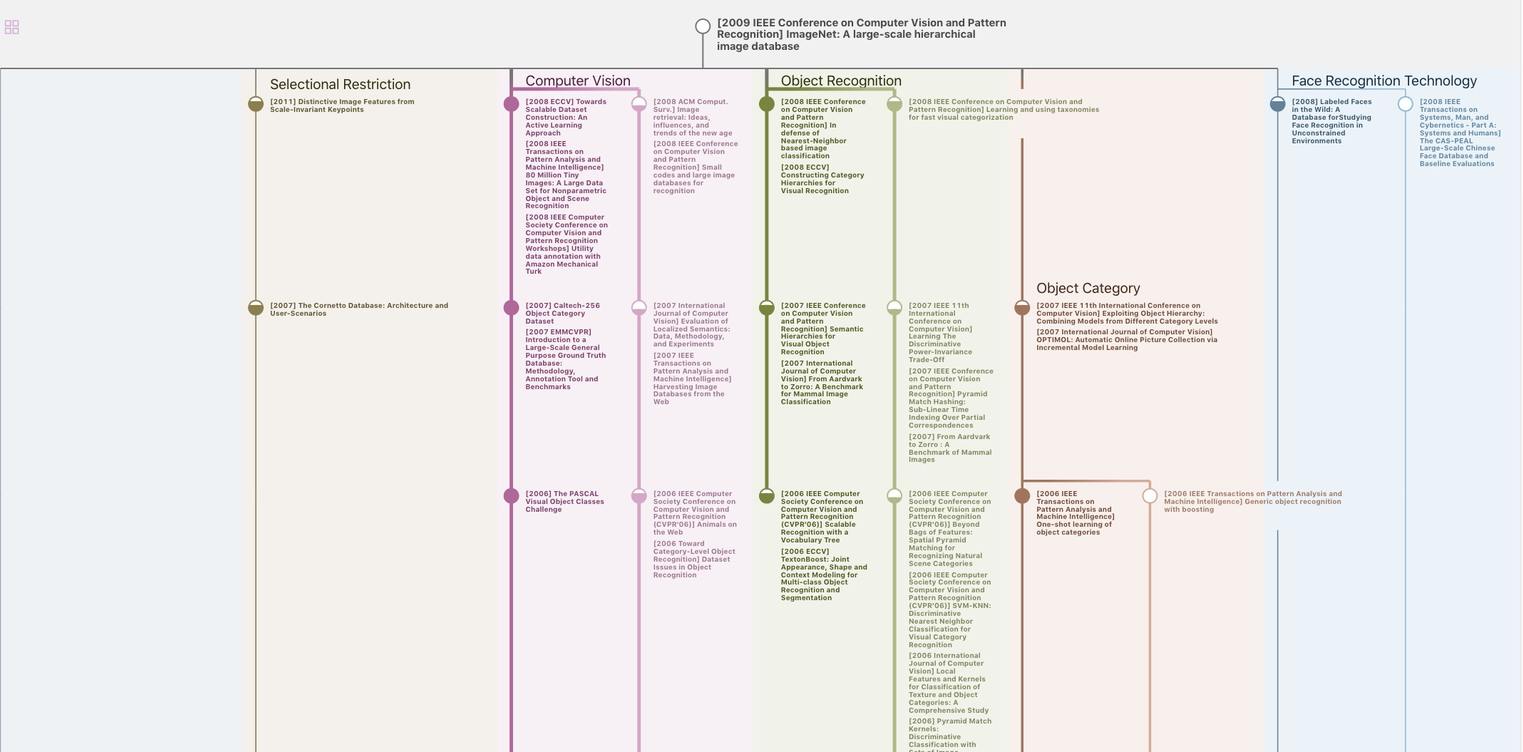
生成溯源树,研究论文发展脉络
Chat Paper
正在生成论文摘要