High Density Crowd Scene Detection in Untrimmed Streaming Videos for Surveillance Purpose.
ECAI(2023)
摘要
This paper aims to develop a fast method that can estimate and extract the scenes with crowds from untrimmed streaming videos for surveillance purpose. To this end, we have proposed a simple but effective methodology using regression-based crowd counting algorithms and a custom scene change detector starting from PySceneDetect library. The proposed approach allows to extract crowd scenes from a large amount of data which are useful for crowd statistics, crowd dynamic analysis and crowd scenarios variations detection. The resulted data collection, named UrbanEvent, aims to enhance the performance of semantic segmentation algorithms on crowd scenes. In particular we conduct extensive experiments on crowd counting methods to use as a primary filter on the candidate scenes. The second filter comprises in the determination of a content value threshold, considering the frame-to-frame variety of the candidate changed scene for crowd surveillance purpose. In addition, the experiments show that the K-means clustering method based on feature embedding from ResNet-152 has good results in high and low crowded scenes, being insensitive on context variety, when the proposed method is sensitive to crowd density variations and also to extrinsic camera parameters changes, minimizing the true-negative collected samples, like the car crowded scenes and cars position changes.
更多查看译文
关键词
statistical approaches,crowd,surveillance,segmentation,aerial images
AI 理解论文
溯源树
样例
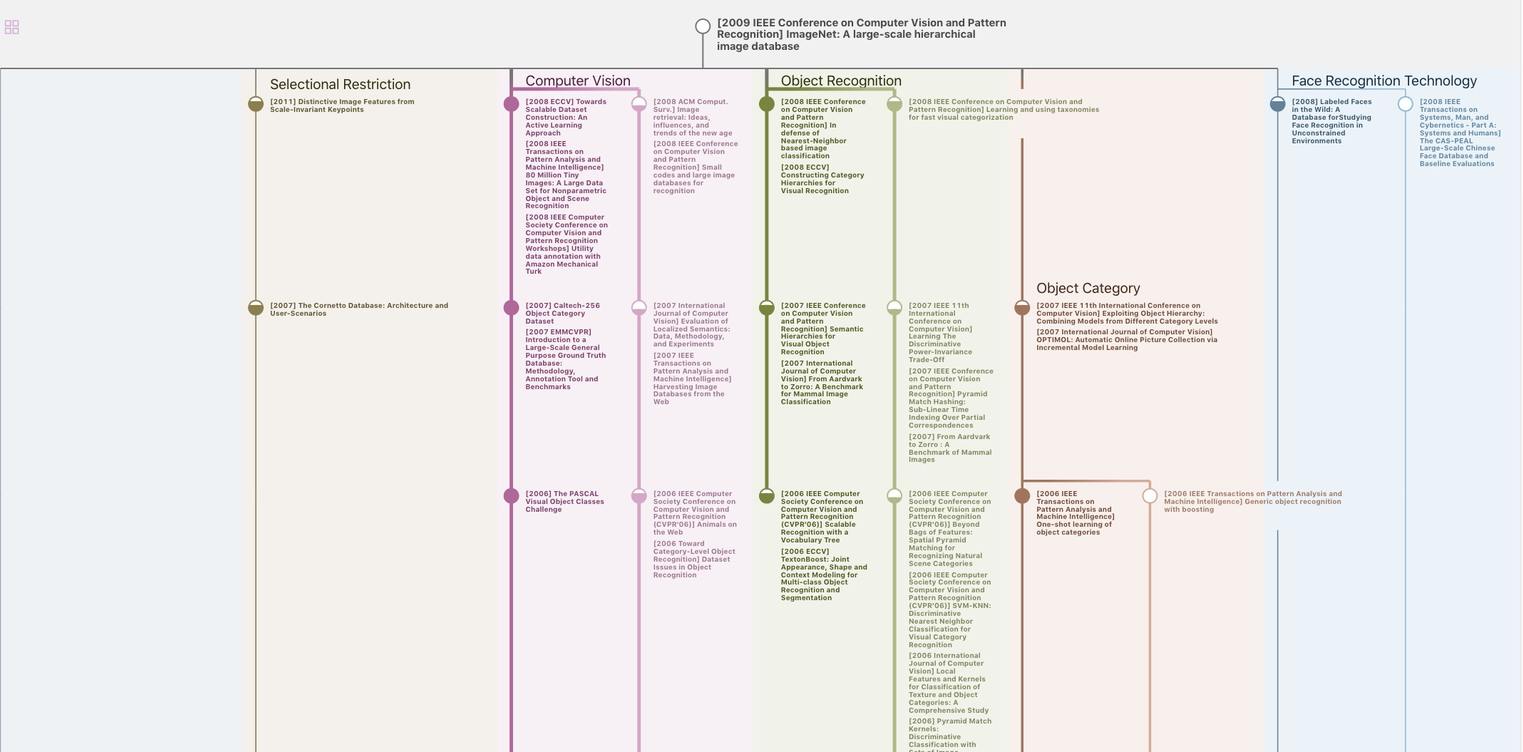
生成溯源树,研究论文发展脉络
Chat Paper
正在生成论文摘要