Memorization of Named Entities in Fine-Tuned BERT Models.
CD-MAKE(2023)
摘要
Privacy preserving deep learning is an emerging field in machine learning that aims to mitigate the privacy risks in the use of deep neural networks. One such risk is training data extraction from language models that have been trained on datasets, which contain personal and privacy sensitive information. In our study, we investigate the extent of named entity memorization in fine-tuned BERT models. We use single-label text classification as representative downstream task and employ three different fine-tuning setups in our experiments, including one with Differentially Privacy (DP). We create a large number of text samples from the fine-tuned BERT models utilizing a custom sequential sampling strategy with two prompting strategies. We search in these samples for named entities and check if they are also present in the fine-tuning datasets. We experiment with two benchmark datasets in the domains of emails and blogs. We show that the application of DP has a detrimental effect on the text generation capabilities of BERT. Furthermore, we show that a fine-tuned BERT does not generate more named entities specific to the fine-tuning dataset than a BERT model that is pre-trained only. This suggests that BERT is unlikely to emit personal or privacy sensitive named entities. Overall, our results are important to understand to what extent BERT-based services are prone to training data extraction attacks (Source code and datasets are available at: https://github.com/drndr/bert_ent_attack . An extended version of this paper can be also found on arXiv [ 12 ]).
更多查看译文
关键词
memorization,named entities,models,fine-tuned
AI 理解论文
溯源树
样例
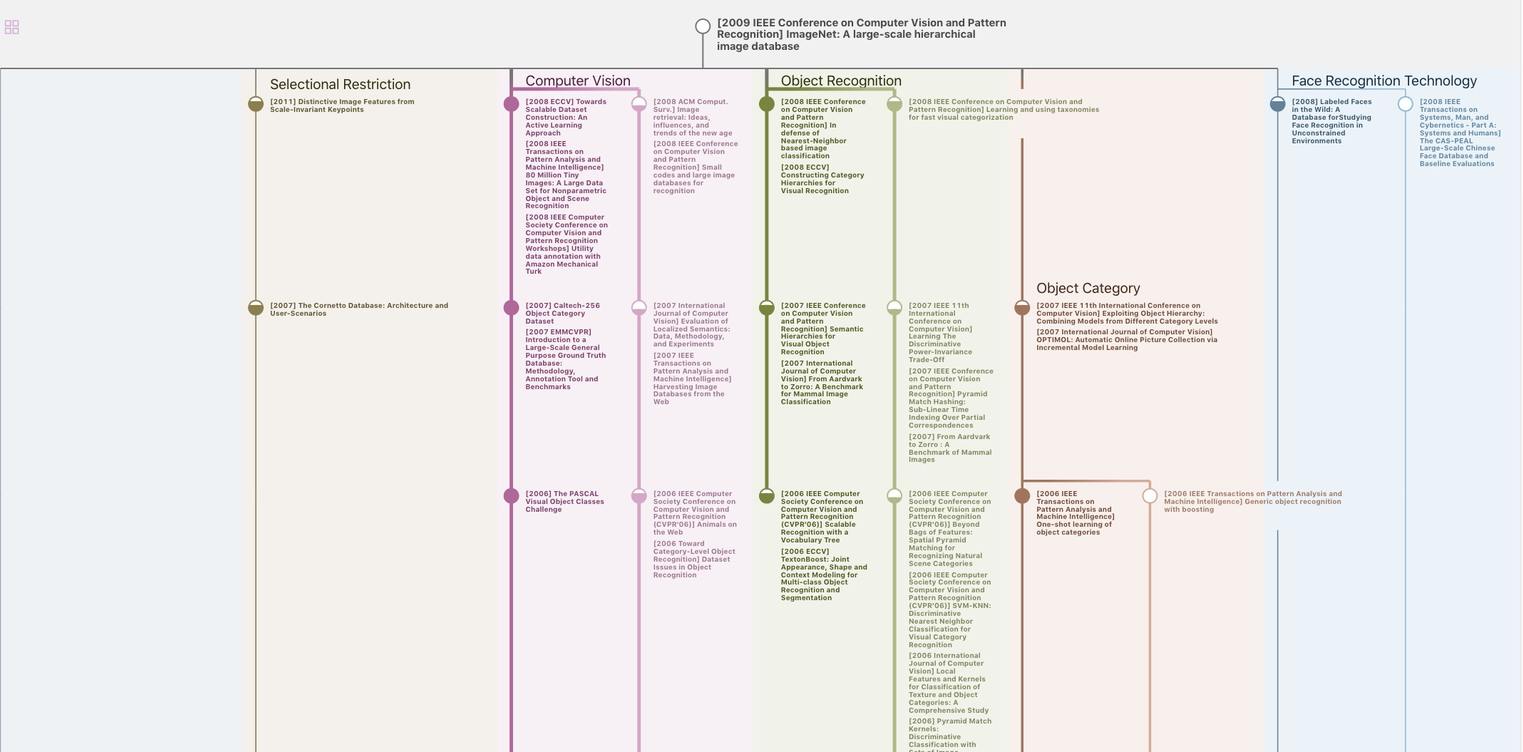
生成溯源树,研究论文发展脉络
Chat Paper
正在生成论文摘要