Deformable Fractional Filters.
AIM(2023)
摘要
This paper introduces Deformable Fractional Filters (DFFs) for Convolutional Neural Networks (CNNs). DFFs enhance the efficiency of conventional Deformable Convolutional Filters by introducing a compression mechanism rooted in techniques from fractional calculus. Concretely, our method reduces the parameter overhead requirement of convolutional filters by replacing the kernel with a fractional approximation, which can be trained using only three parameters - regardless of the kernel size. DFFs present a compelling use case for the compression of networks that require large kernel sizes. To demonstrate the benefits of DFFs, we report experimental results across a diverse set of computer vision problem domains, including classification, semantic segmentation, and medical imaging. Our experiments illustrate the favorable performance and regularization properties presented by DFFs in comparison with other baseline CNNs.
更多查看译文
关键词
compression mechanism,conventional Deformable Convolutional Filters,Convolutional Neural Networks,Deformable Fractional Filters,DFFs,fractional approximation,fractional calculus,kernel size,parameter overhead requirement
AI 理解论文
溯源树
样例
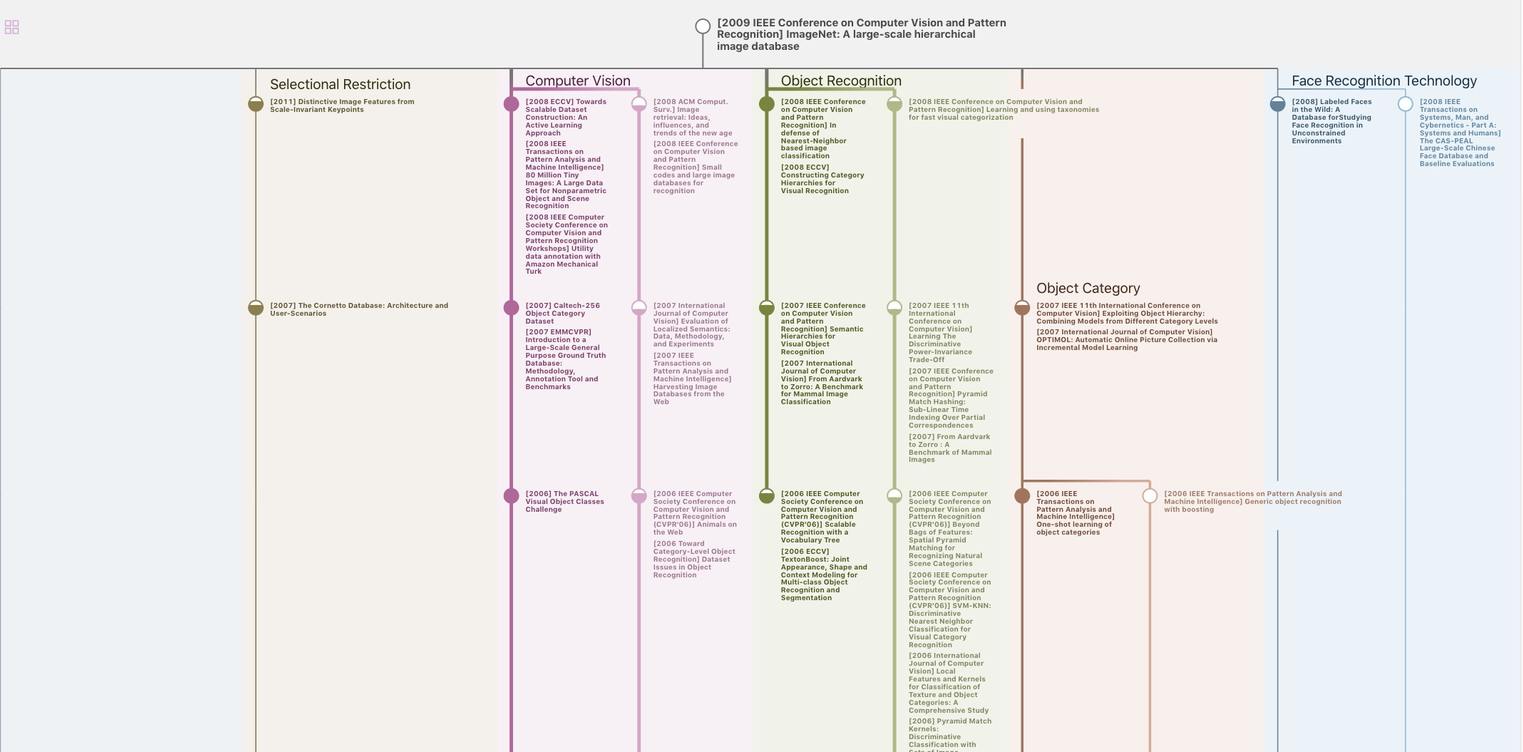
生成溯源树,研究论文发展脉络
Chat Paper
正在生成论文摘要