Zero-Shot Action Recognition with Transformer-based Video Semantic Embedding.
CVPR Workshops(2023)
摘要
While video action recognition has been an active area of research for several years, zero-shot action recognition has only recently started gaining traction. In this work, we propose a novel end-to-end trained transformer model which is capable of capturing long range spatiotemporal dependencies efficiently, contrary to existing approaches which use 3D-CNNs. Moreover, to address a common ambiguity in the existing works about classes that can be considered as previously unseen, we propose a new experimentation setup that satisfies the zero-shot learning premise for action recognition by avoiding overlap between the training and testing classes. The proposed approach significantly outperforms the state of the arts in zero-shot action recognition in terms of the the top-1 accuracy on UCF-101, HMDB-51 and ActivityNet datasets.
更多查看译文
关键词
end-to-end trained transformer model,transformer-based video semantic,video action recognition,zero-shot action recognition,zero-shot learning premise
AI 理解论文
溯源树
样例
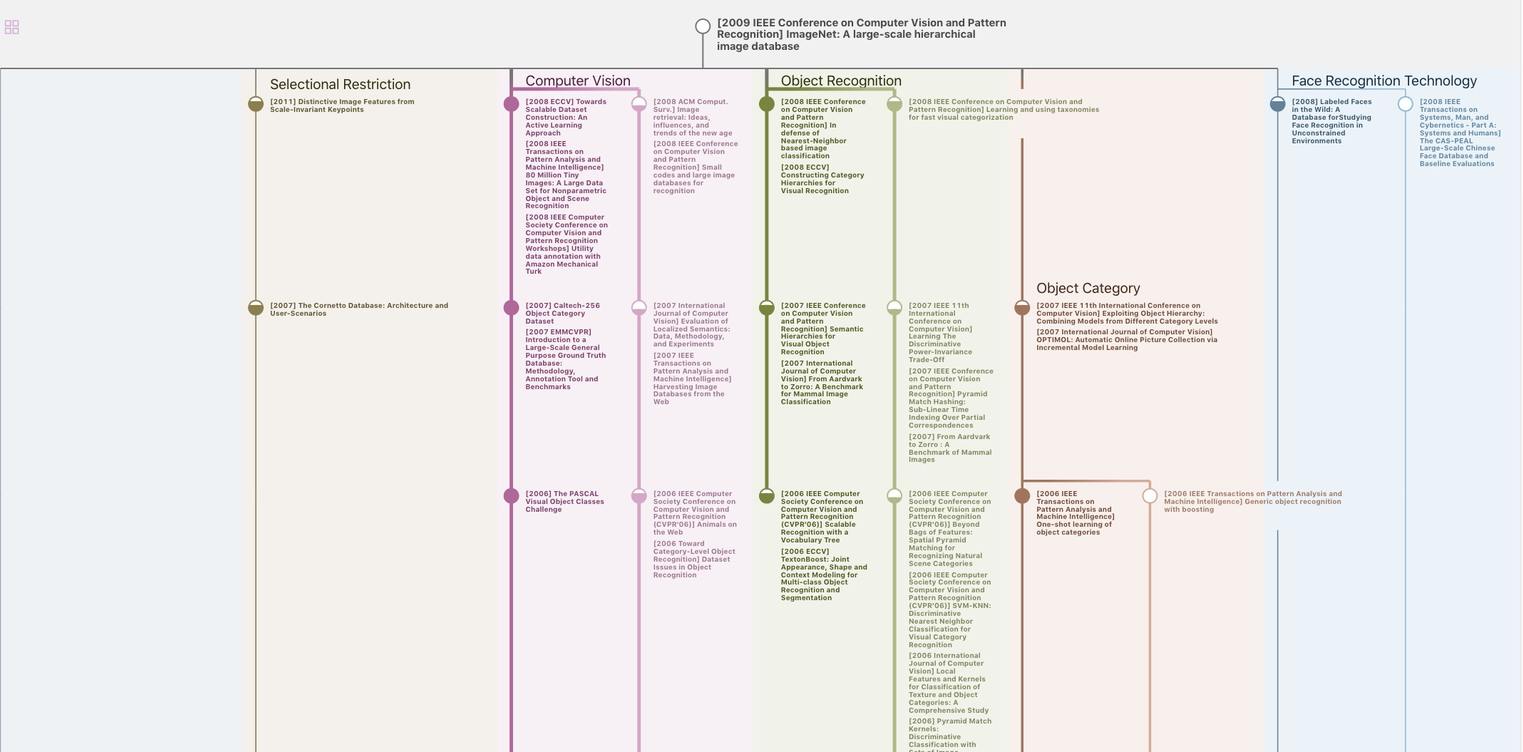
生成溯源树,研究论文发展脉络
Chat Paper
正在生成论文摘要