Tensor Chain Decomposition and Function Interpolation.
SSP(2023)
摘要
Tensor Chain (TC) decomposition represents a given tensor as a chain (circle) of order-3 tensors (wagons) connected through tensor contractions. In this paper, we show the link between the TC decomposition and a structured Tucker decompositions, and propose a variant of the Krylov-Levenberg-Marquardt optimization, tailored for this problem. Many extensions can be considered, here we only mention decomposition of tensor with missing entries, which enables the tensor completion. Performance of the proposed algorithm is demonstrated on tensor decomposition of the sampled Rosenbrock function. It can be better modeled both as TC and canonical polyadic (CP) decomposition, but with TC, the reconstruction is possible with a lower number of function values.
更多查看译文
关键词
Multilinear models,canonical polyadic decomposition,tensor train
AI 理解论文
溯源树
样例
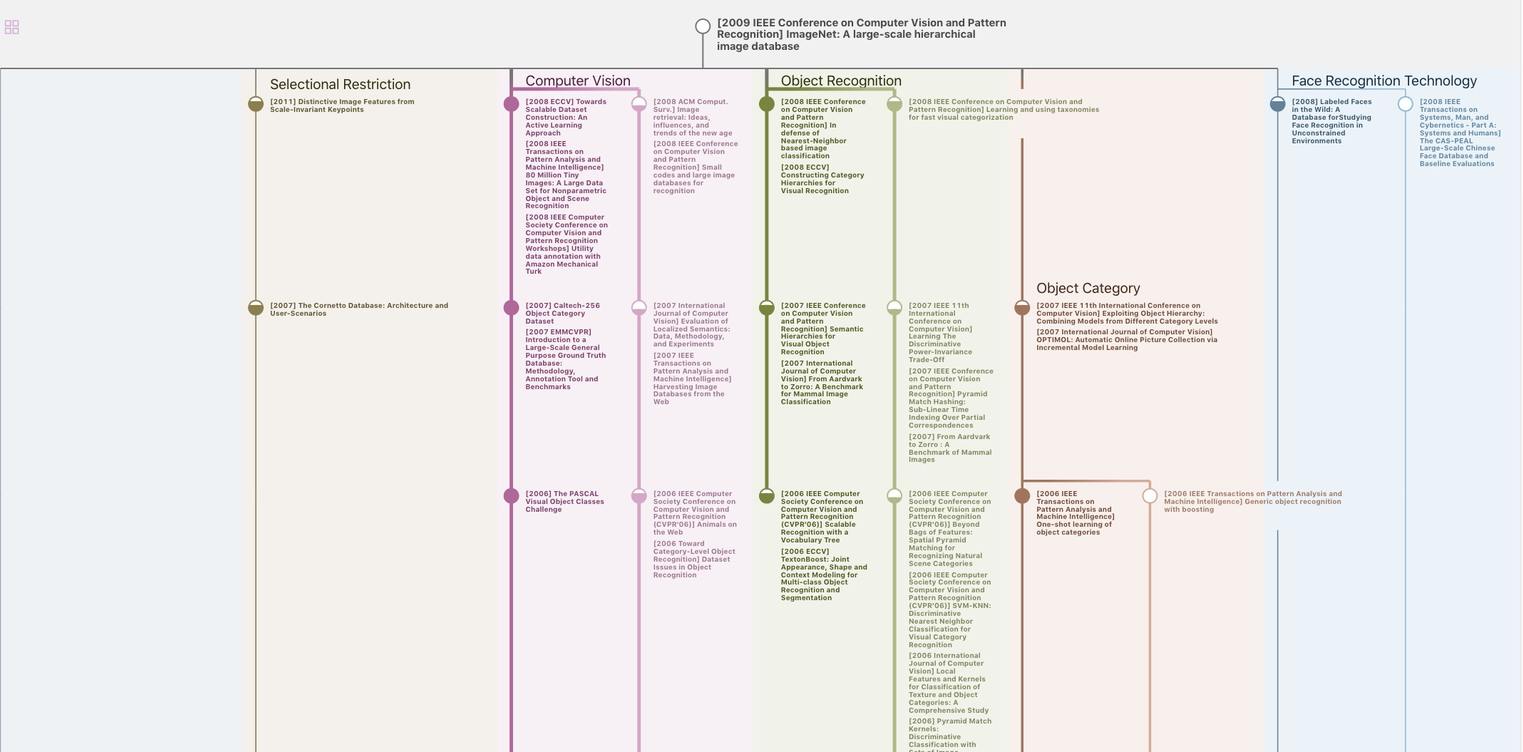
生成溯源树,研究论文发展脉络
Chat Paper
正在生成论文摘要