Dual-Input Type Convolutional Neural Networks Employing Color Normalized and Nuclei Segmented Data for Histopathology Image Classification.
SSP(2023)
摘要
Improvements in Convolutional Neural Network (CNN) have been widely successful for histopathology image classification. However, color normalization for data preprocessing and nuclei segmentation for feature extraction should also be considered for further performance boost, data redundancy elimination, and provision of distinguishing information. These techniques are known to improve generalizability. However, there is a need to find ways to use the data obtained from color normalized and segmented data for training. In this work, dual-input CNN (DiCNN), concatenated-input CNN (CiCNN), and ensemble CNN (ECNN) are trained and tested with color normalized and nuclei segmented data. The normalization technique is chosen based on correlation and structural similarity. The segmentation method is chosen based on the best-performing normalization technique for consistency and generalizability. The results show that normalized and segmented inputs results in better binary classification with CiCNN outperforming other methods. However, for multiclass
更多查看译文
关键词
Histopathology, Color Normalization, Nuclei Segmentation, Dual Input CNN, Image Classification
AI 理解论文
溯源树
样例
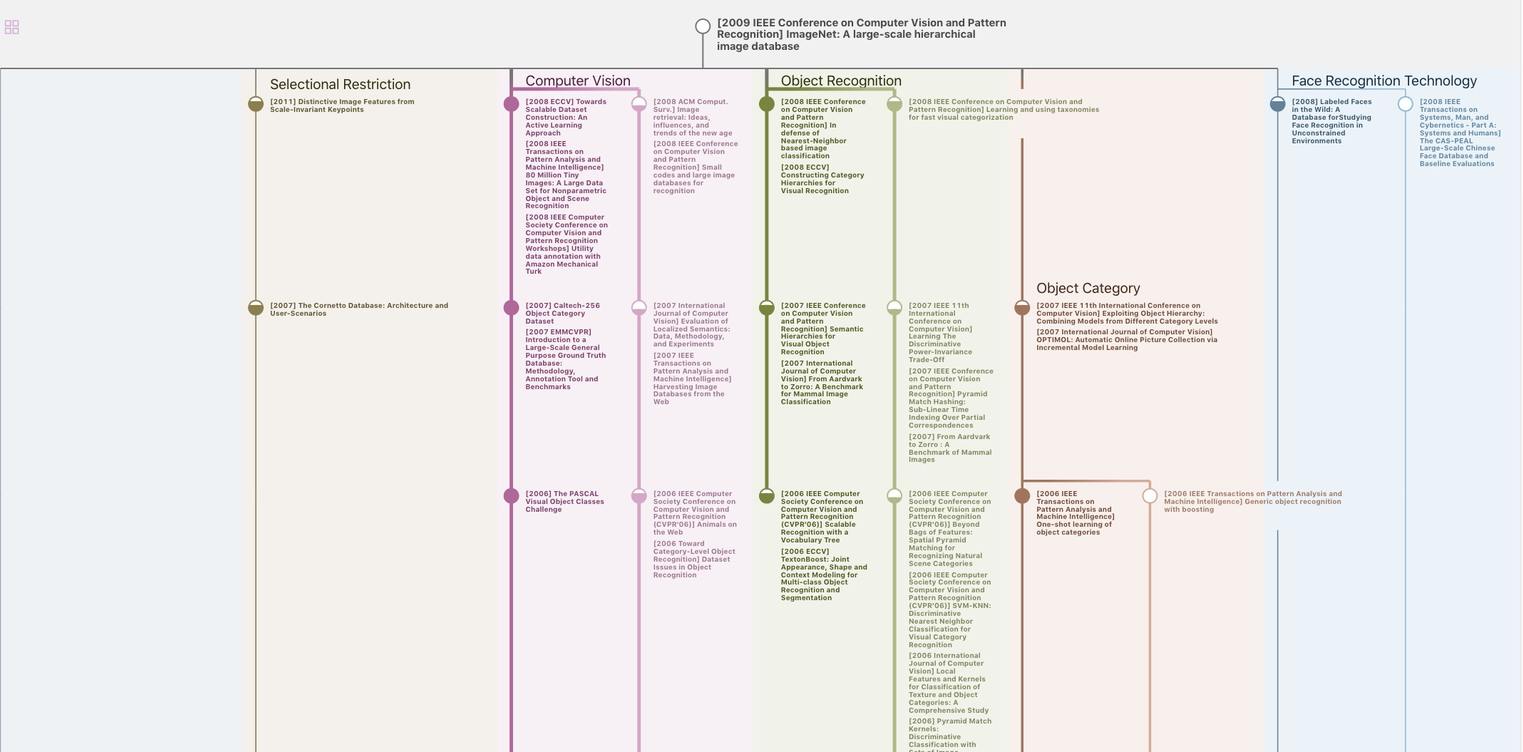
生成溯源树,研究论文发展脉络
Chat Paper
正在生成论文摘要