Improving Deep Learning-based Automatic Checkout System Using Image Enhancement Techniques.
CVPR Workshops(2023)
摘要
The retail sector has experienced significant growth in artificial intelligence and computer vision applications, particularly with the emergence of automatic checkout (ACO) systems in stores and supermarkets. ACO systems encounter challenges such as object occlusion, motion blur, and similarity between scanned items while acquiring accurate training images for realistic checkout scenarios is difficult due to constant product updates. This paper improves existing deep learning-based ACO solutions by incorporating several image enhancement techniques in the data pre-processing step. The proposed ACO system employs a detect-and-track strategy, which involves: (1) detecting objects in areas of interest; (2) tracking objects in consecutive frames; and (3) counting objects using a track management pipeline. Several data generation techniques—including copy-and-paste, random placement, and augmentation—are employed to create diverse training data. Additionally, the proposed solution is designed as an open-ended framework that can be easily expanded to accommodate multiple tasks. The system has been evaluated on the AI City Challenge 2023 Track 4 dataset, showcasing outstanding performance by achieving a top-1 ranking on test-set A with an F1 score of 0.9792.
更多查看译文
关键词
ACO system,AI City Challenge 2023 Track 4 dataset,artificial intelligence,augmentation techniques,automatic checkout systems,computer vision applications,constant product updates,copy-and-paste techniques,data generation techniques,deep learning-based ACO solutions,detect-and-track strategy,diverse training data,image enhancement techniques,motion blur,object occlusion,random placement techniques,retail sector,supermarkets,track management pipeline
AI 理解论文
溯源树
样例
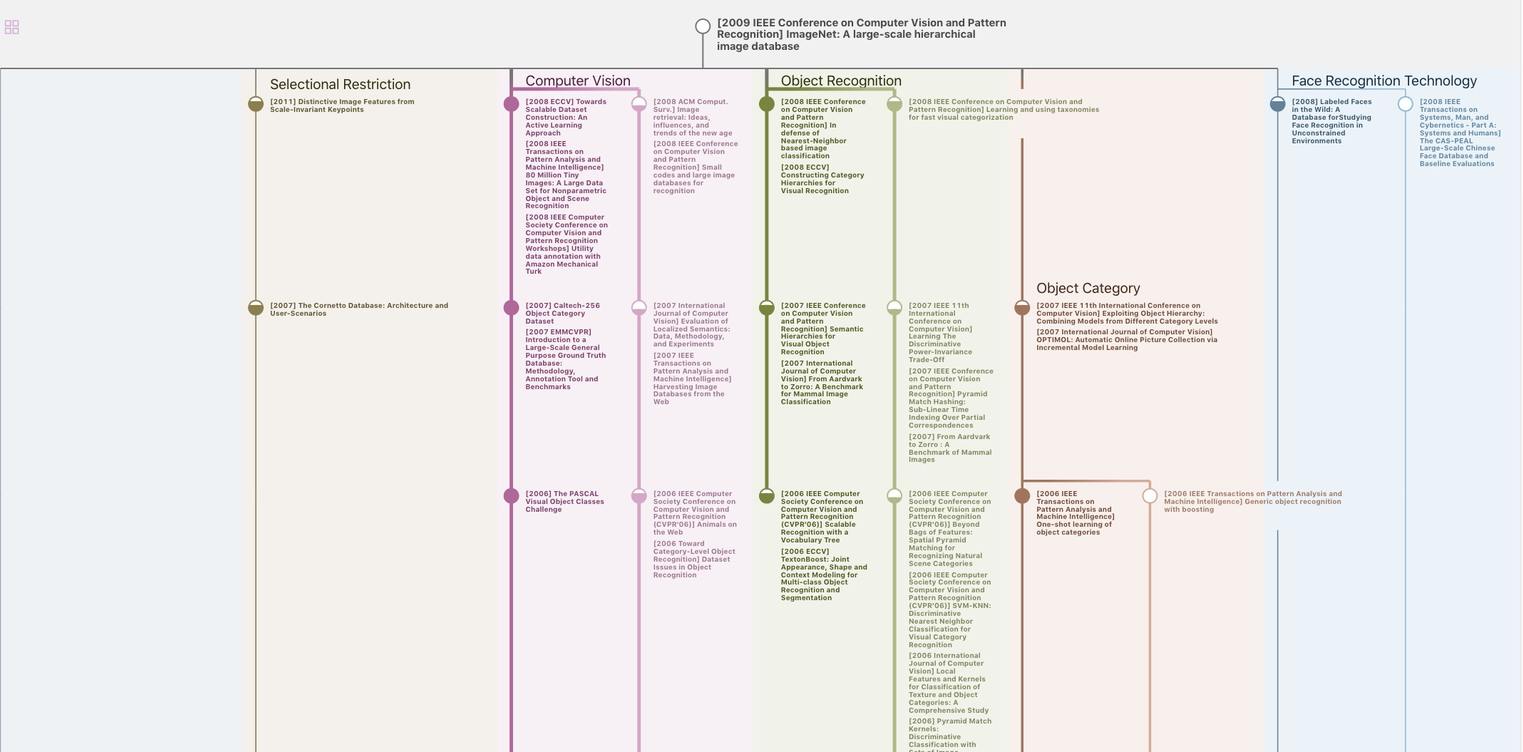
生成溯源树,研究论文发展脉络
Chat Paper
正在生成论文摘要