CRCS: Learning Synergistic Cascade Correlation for Microscopic Cascade Prediction.
SmartWorld/UIC/ScalCom/DigitalTwin/PriComp/Meta(2022)
摘要
Information diffusion prediction is the basis of many fundamental tasks, such as social recommendation and community detection. Currently, most researchers infer user correlation based on cascade records to predict future infected users. However, they only consider whether the infected users at each location are correctly predicted, ignoring the semantic differences between users who participate in the retweets of similar content and other users. This naturally leads to learning biased user features. In this paper, we compute semantic relevance between two different cascades (e.g., c
m
and c
n
) based on user coincidence and develop the cascade correlation graph (CCG) to learn user features. Afterward, we devise a position-independent sequence similarity loss (SSL) function combined with an auxiliary predictor to optimize the user encoder. The above two methods make users participating in the relevant cascades more relevant than others. Experiments show that the two methods are effective, and the combination of them can improve performance more significantly.
更多查看译文
关键词
social network analysis,information diffusion prediction,heterogeneous graph,loss function
AI 理解论文
溯源树
样例
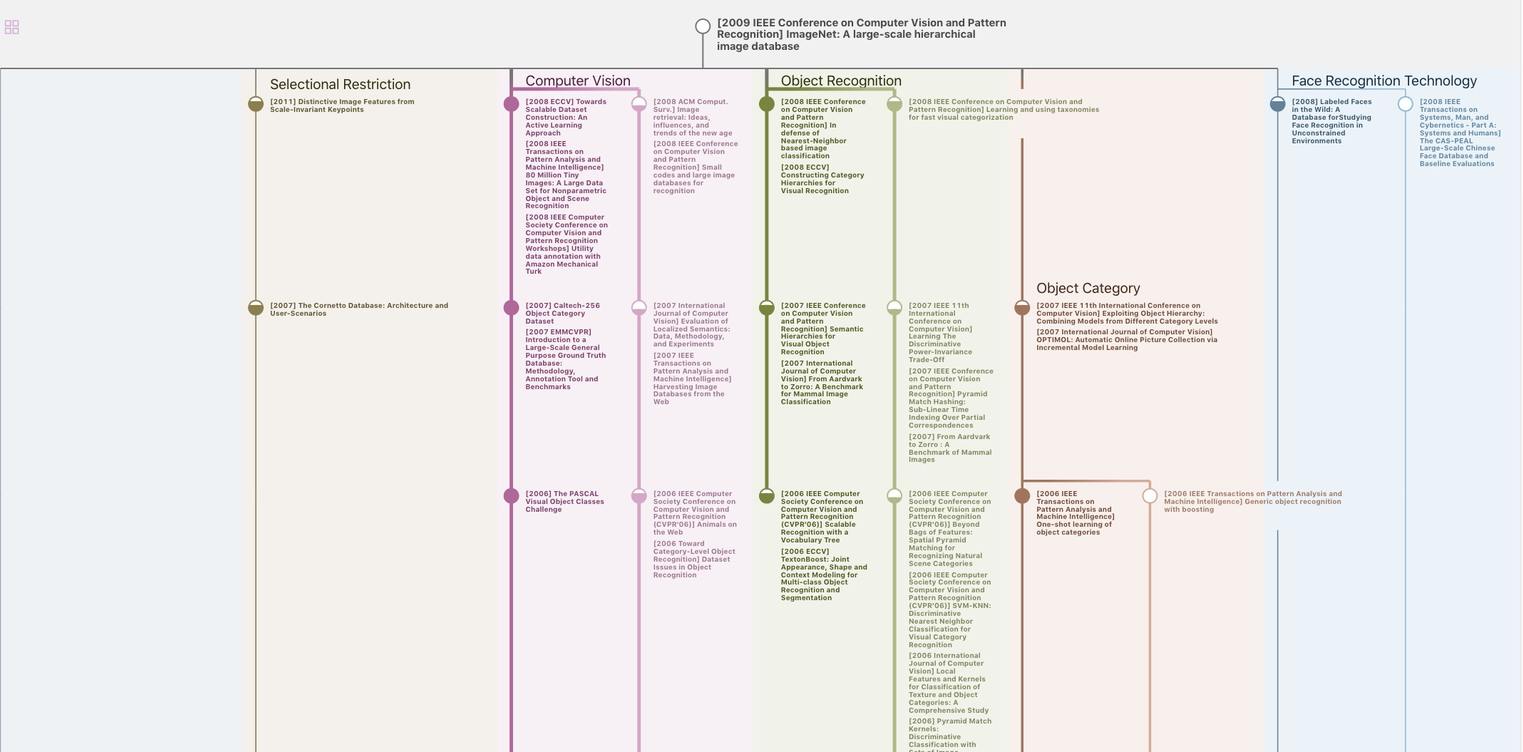
生成溯源树,研究论文发展脉络
Chat Paper
正在生成论文摘要