Vehicle Check-In Data-driven POI Recommendation Based on Improved SVD and Graph Convolutional Network.
SmartWorld/UIC/ScalCom/DigitalTwin/PriComp/Meta(2022)
摘要
The application of automobile tool software is becoming increasingly widespread, and its functions are becoming increasingly diverse. Using vehicle traffic data to give users with recommendations for Points-of-interest (POI) is becoming an intriguing research challenge. However, the current POI recommendation is either a sequence-based method, ignoring the interaction information between users and POIs. Or it needs a lot of model training time to use graph correlation method to mine the interaction between users or vehicles and POIs. In order to address these issues, we propose a method for vehicle check-in data-driven POI recommendation based on improved Singular Value Decomposition (SVD) and Graph Convolutional Network (GCN). First, we apply SVD to replace the neighborhood aggregation update method in traditional GCN, update the node embedding with fewer parameters and faster speed. Second, we propose an information enhancement strategy to make up for the information loss caused by the SVD. Experiments on large vehicle check-in datasets demonstrate that our method is both faster and more effective when making POI recommendations.
更多查看译文
关键词
Graph Convolutional Network,low-rank,singular value decomposition,vehicle systems
AI 理解论文
溯源树
样例
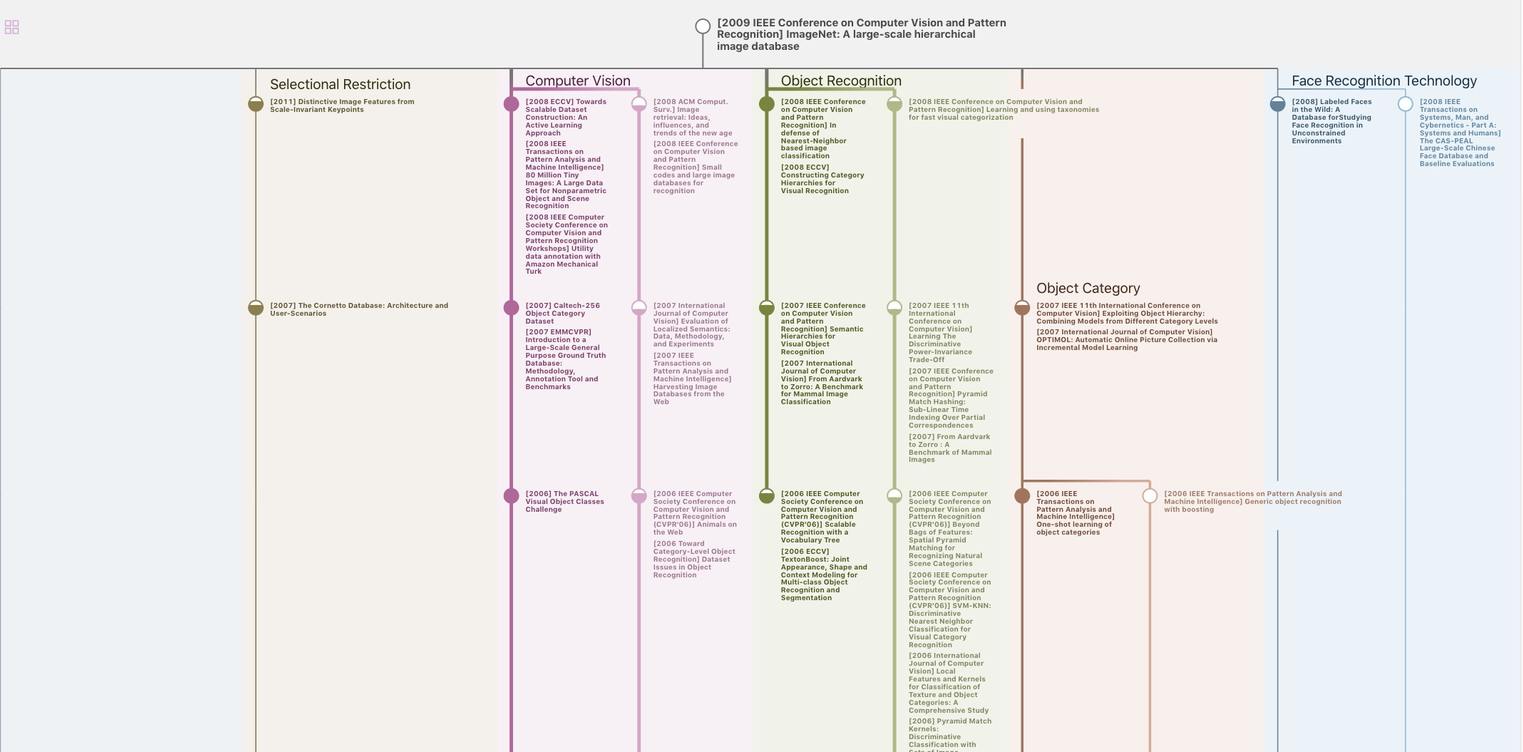
生成溯源树,研究论文发展脉络
Chat Paper
正在生成论文摘要