Phase-field Models for Lightweight Graph Convolutional Networks.
CVPR Workshops(2023)
摘要
In this paper, we design lightweight graph convolutional networks (GCNs) using a particular class of regularizers, dubbed as phase-field models (PFMs). PFMs exhibit a bi-phase behavior using a particular ultra-local term that allows training both the topology and the weight parameters of GCNs as a part of a single "end-to-end" optimization problem. Our proposed solution also relies on a reparametrization that pushes the mask of the topology towards binary values leading to effective topology selection and high generalization while implementing any targeted pruning rate. Both masks and weights share the same set of latent variables and this further enhances the generalization power of the resulting lightweight GCNs. Extensive experiments conducted on the challenging task of skeleton-based recognition show the outperformance of PFMs against other staple regularizers as well as related lightweight design methods.
更多查看译文
关键词
bi-phase behavior,end-to-end optimization problem,lightweight GCNs,lightweight graph convolutional networks,PFMs,phase-field models,pruning rate,regularizers,related lightweight design methods,reparametrization,skeleton-based recognition,topology selection
AI 理解论文
溯源树
样例
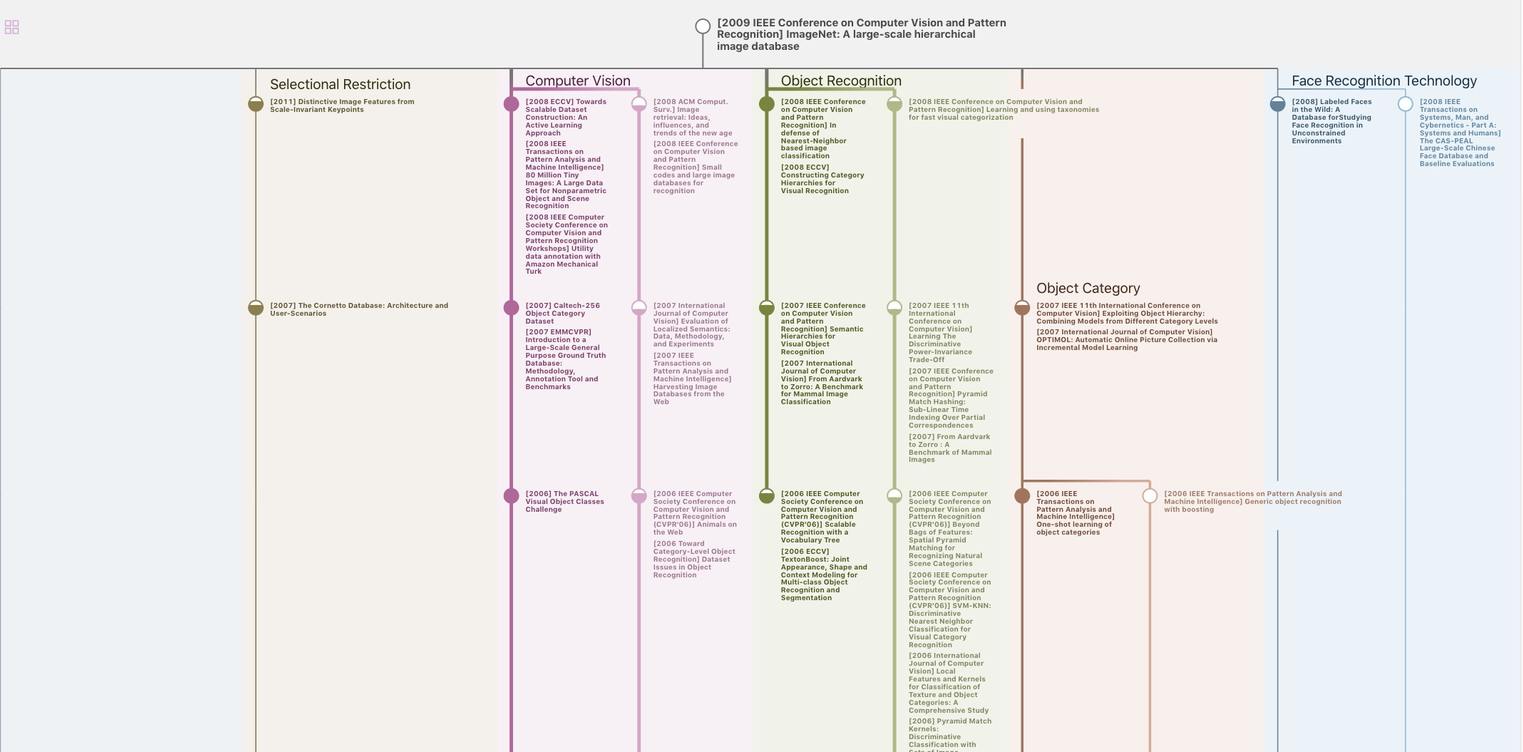
生成溯源树,研究论文发展脉络
Chat Paper
正在生成论文摘要