The Effectiveness of Quantum Random Walk Model in Recommender Systems
PROCEEDINGS OF THE 2023 ACM SIGIR INTERNATIONAL CONFERENCE ON THE THEORY OF INFORMATION RETRIEVAL, ICTIR 2023(2023)
摘要
Graph Convolutional Networks (GCNs) are effective in providing more relevant items at higher rankings in recommender systems. However, in real-world scenarios, it is important to provide recommended items with diversity and novelty as well as relevance to each user's preference. Additionally, users often desire a wide range of recommendations not just based on their past search behaviors and histories. To enhance each user's satisfaction, it is important to develop a recommender system that provides much more relevant and diverse items. LightGCN can achieve this, which is a GCN-based recommender system that learns latent vectors of users and items using multiple layers of aggregation functions and an adjacency matrix. However, LightGCN often provides recommendations without diversity when the number of layers is insufficient. On the other hand, when the number is excessive, the accuracy declines, which is known as the over-smoothing problem. To overcome this, we propose a novel approach using a continuous-time quantum walk model derived from a quantum algorithm to reconstruct the user-item adjacency matrix of LightGCN, improving the relevance and diversity of recommendations. We release our codes.(1)
更多查看译文
关键词
Recommender Systems,Graph Convolutional Network,Quantum Walk
AI 理解论文
溯源树
样例
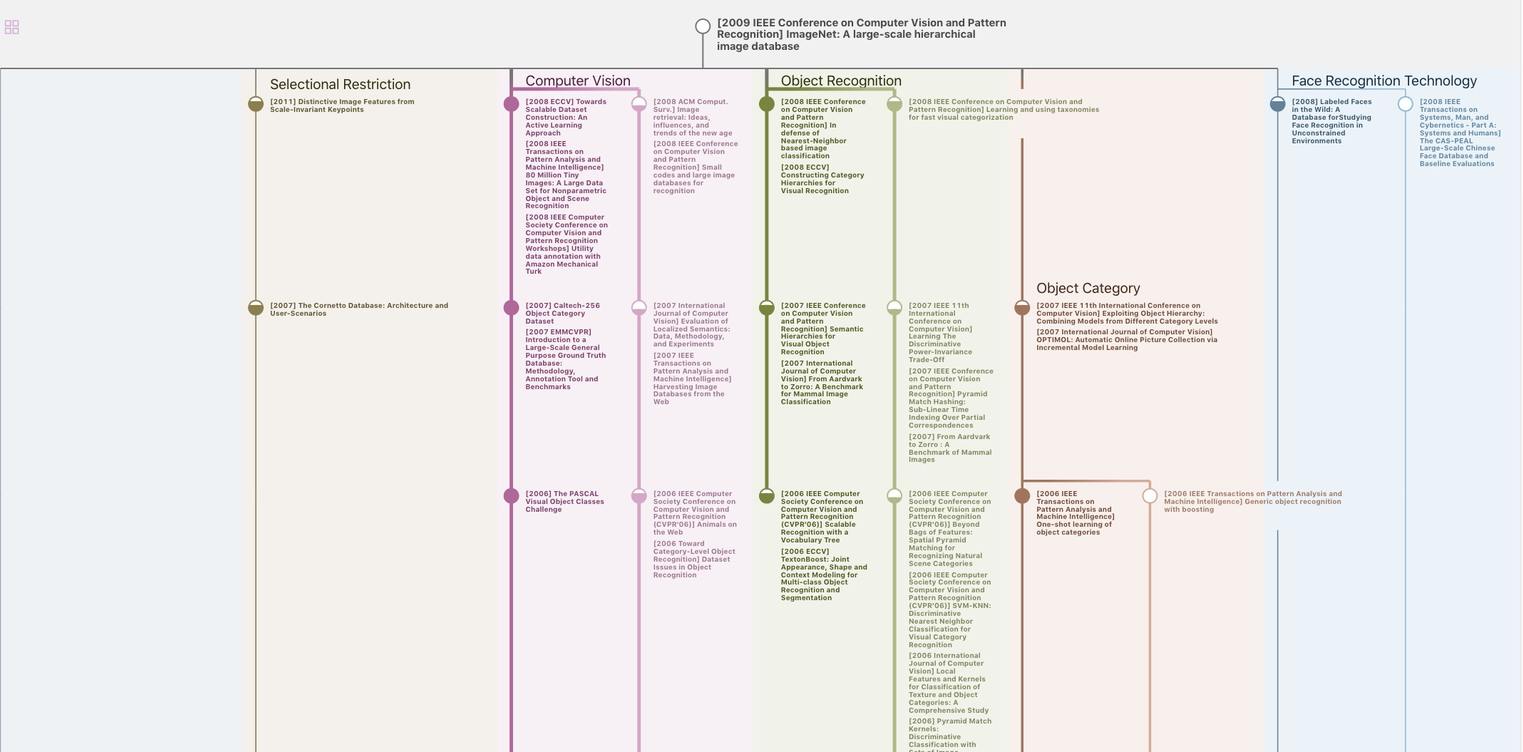
生成溯源树,研究论文发展脉络
Chat Paper
正在生成论文摘要