Assessment of the Quality of Topic Models for Information Retrieval Applications
PROCEEDINGS OF THE 2023 ACM SIGIR INTERNATIONAL CONFERENCE ON THE THEORY OF INFORMATION RETRIEVAL, ICTIR 2023(2023)
摘要
Topic modelling is an approach to generation of descriptions of document collections as a set of topics where each has a distinct theme and documents are a blend of topics. It has been applied to retrieval in a range of ways, but there has been little prior work on measurement of whether the topics are descriptive in this context. Moreover, existing methods for assessment of topic quality do not consider how well individual documents are described. To address this issue we propose a new measure of topic quality, which we call specificity; the basis of this measure is the extent to which individual documents are described by a limited number of topics. We also propose a new experimental protocol for validating topic-quality measures, a 'noise dial' that quantifies the extent to which the measure's scores are altered as the topics are degraded by addition of noise. The principle of the mechanism is that a meaningful measure should produce low scores if the 'topics' are essentially random. We show that specificity is at least as effective as existing measures of topic quality and does not require external resources. While other measures relate only to topics, not to documents, we further show that specificity correlates to the extent to which topic models are informative in the retrieval process.
更多查看译文
关键词
topic modelling,topic coherence,collection representation
AI 理解论文
溯源树
样例
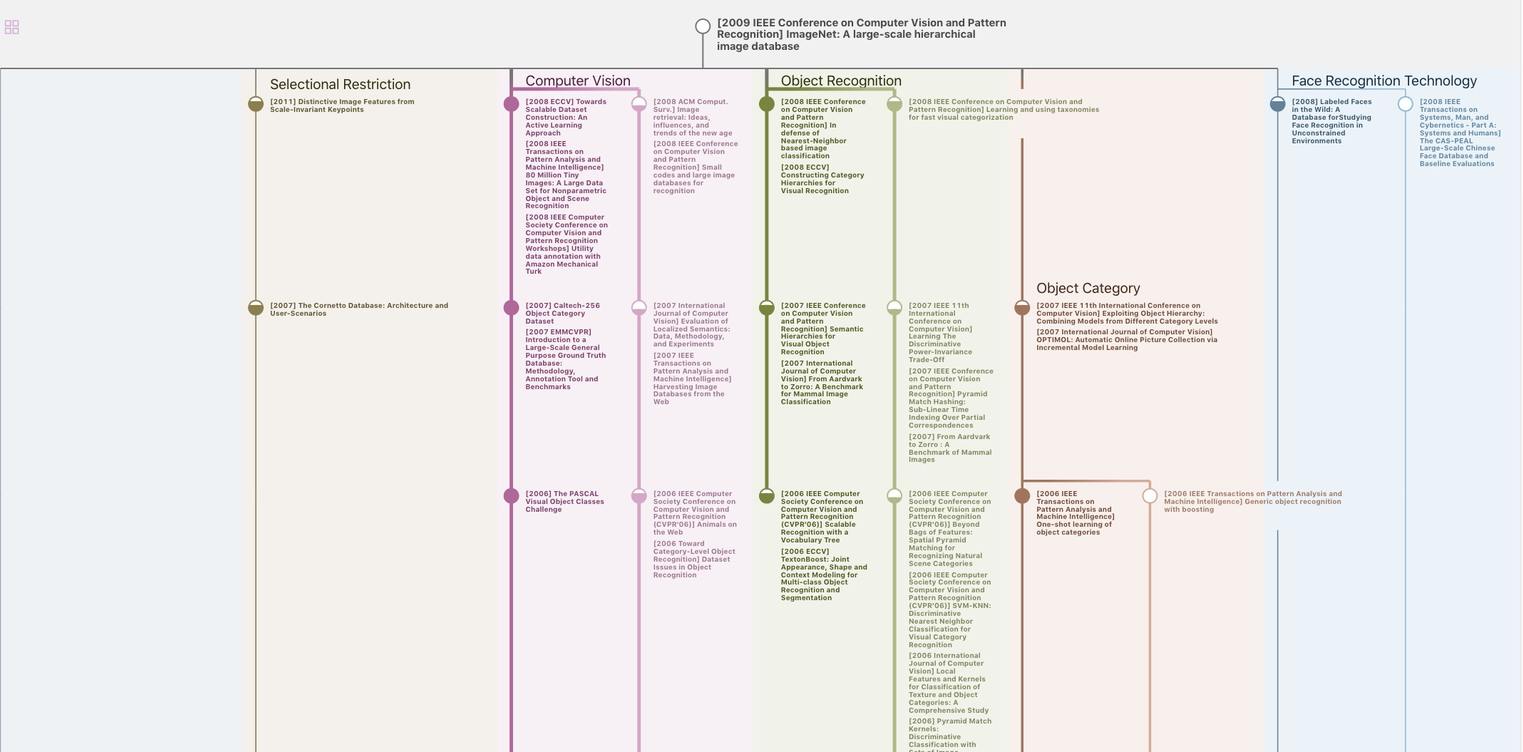
生成溯源树,研究论文发展脉络
Chat Paper
正在生成论文摘要