Balanced Knowledge Distillation with Contrastive Learning for Document Re-ranking
PROCEEDINGS OF THE 2023 ACM SIGIR INTERNATIONAL CONFERENCE ON THE THEORY OF INFORMATION RETRIEVAL, ICTIR 2023(2023)
摘要
Knowledge distillation is commonly used in training a neural document ranking model by employing a teacher to guide model refinement. As a teacher may not be correct in all cases, over-calibration between the student and teacher models can make training less effective. This paper focuses on the KL divergence loss used for knowledge distillation in document re-ranking, and re-visits balancing of knowledge distillation with explicit contrastive learning. The proposed loss function takes a conservative approach in imitating teacher's behavior, and allows student to deviate from a teacher's model sometimes through training. This paper presents analytic results with an evaluation on MS MARCO passages to validate the usefulness of the proposed loss for the transformer-based ColBERT re-ranking.
更多查看译文
关键词
Neural document ranking,knowledge distillation,KL divergence
AI 理解论文
溯源树
样例
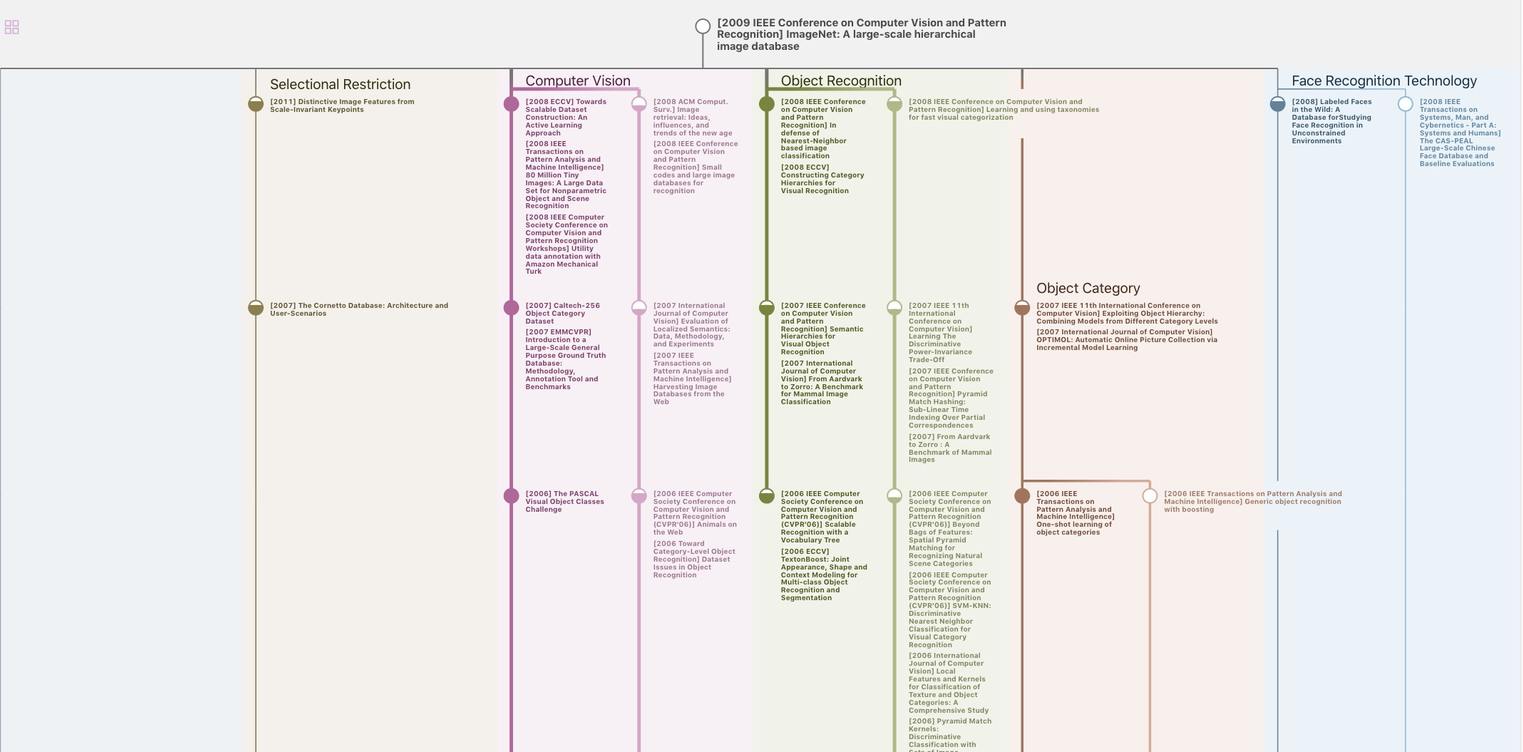
生成溯源树,研究论文发展脉络
Chat Paper
正在生成论文摘要