International Workshop on Federated Learning for Distributed Data Mining
PROCEEDINGS OF THE 29TH ACM SIGKDD CONFERENCE ON KNOWLEDGE DISCOVERY AND DATA MINING, KDD 2023(2023)
摘要
The past decade has witnessed wide applications of machine learning to various domains for decision-making, including crime detection, urban planning, drug discovery, and health monitoring, which benefited from surging data resources. As data collection in real-world applications is often done in different locations, being able to mine and discover knowledge from distributed data sources is an essential requirement for building powerful predictive models. However, directly uploading all data sources to an untrustworthy centralized data server for learning will lead to risks of privacy leakage. Federated Learning (FL) emerges as a decentralized learning framework that aggregates knowledge from distributed data without centralizing them, hence mitigating privacy risks. By hosting this workshop, we aim to attract a broad spectrum of audiences, including researchers and practitioners from academia and industry interested in the latest advances in FL. As an effort to advance the fundamental development of FL in data mining, this workshop will encourage ideas exchange on the trustworthiness, scalability, robustness, and broad applications of FL.
更多查看译文
关键词
federated learning,distributed data mining,trustworthiness,applications
AI 理解论文
溯源树
样例
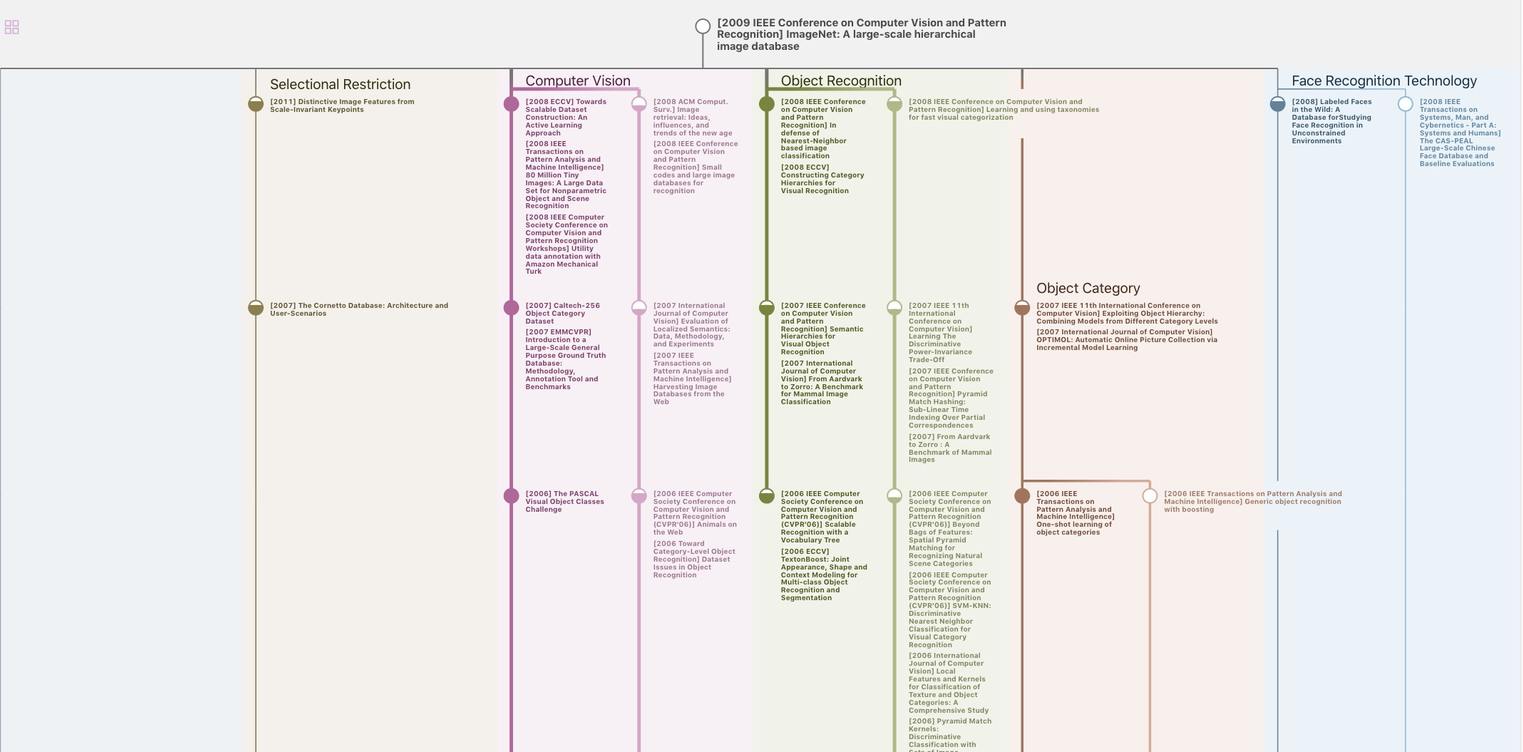
生成溯源树,研究论文发展脉络
Chat Paper
正在生成论文摘要