Hands-on Tutorial: "Explanations in AI: Methods, Stakeholders and Pitfalls".
KDD(2023)
摘要
While using vast amounts of training data and sophisticated models has enhanced the predictive performance of Machine Learning (ML) and Artificial Intelligence (AI) solutions, it has also led to an increased difficulty in comprehending their predictions. The ability to explain predictions is often one of the primary desiderata for adopting AI and ML solutions [6, 13]. The desire for explainability has led to a rapidly growing body of literature on explainable AI (XAI) and has also resulted in the development of hundreds of XAI methods targeting different domains (e.g., finance, healthcare), applications (e.g., model debugging, actionable recourse), data modalities (e.g., tabular data, images), models (e.g., transformers, convolutional neural networks) and stakeholders (e.g., end-users, regulatory authorities, data scientists). The goal of this tutorial is to present a comprehensive overview of the XAI field to the participants. As a hands-on tutorial, we will showcase state-of-the-art methods that can be used for different data modalities and contexts to extract the right abstractions for interpretation. We will also cover common pitfalls when using explanations, e.g., misrepresentation, and lack of robustness of explanations.
更多查看译文
AI 理解论文
溯源树
样例
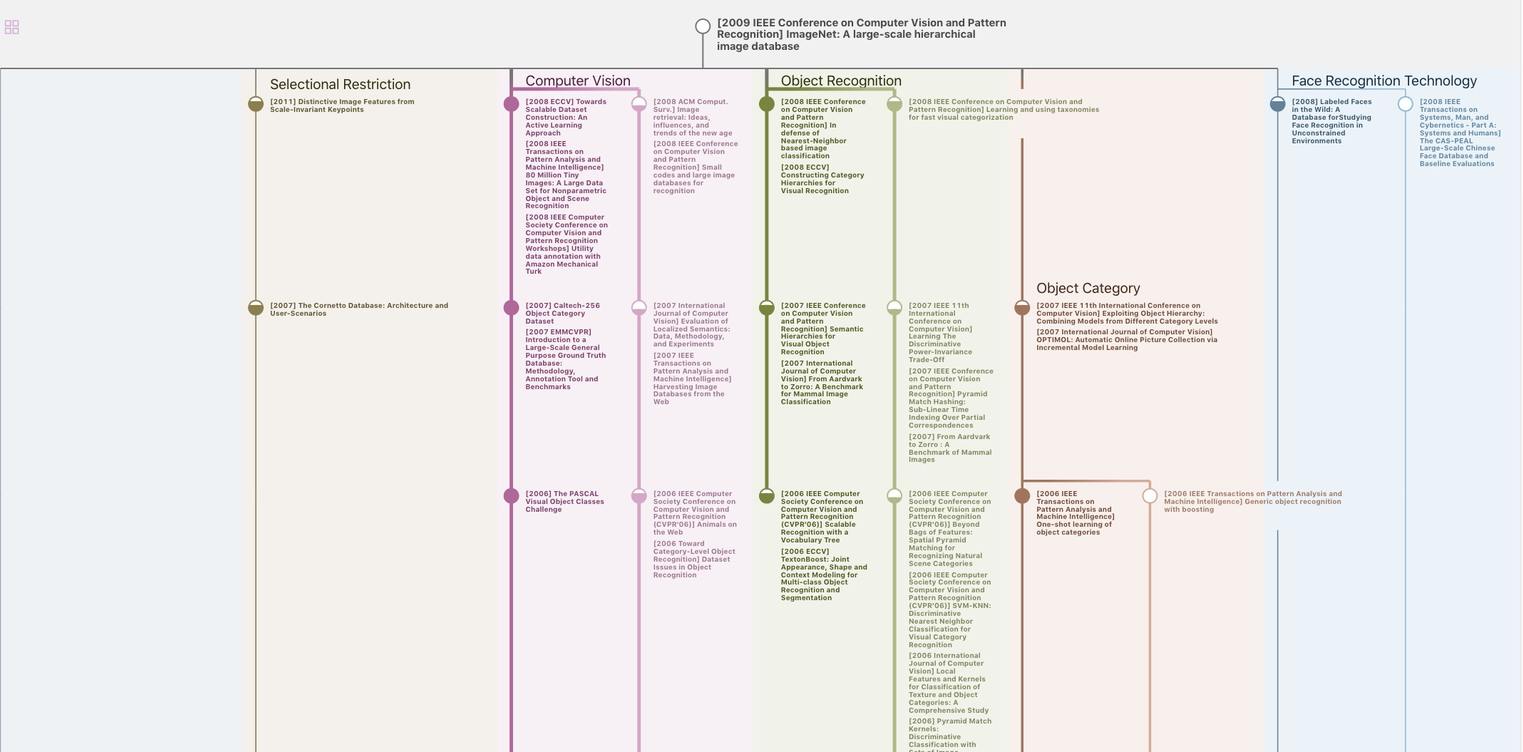
生成溯源树,研究论文发展脉络
Chat Paper
正在生成论文摘要