Knowledge-augmented Graph Machine Learning for Drug Discovery: From Precision to Interpretability
PROCEEDINGS OF THE 29TH ACM SIGKDD CONFERENCE ON KNOWLEDGE DISCOVERY AND DATA MINING, KDD 2023(2023)
摘要
Graph Machine Learning (GML) has gained considerable attention for its exceptional ability to model graph-structured biomedical data and investigate their properties and functional relationships. Despite extensive efforts, GML methods still suffer from several deficiencies, such as the limited ability to handle supervision sparsity and provide interpretability in learning and inference processes and their ineffectiveness in utilising relevant domain knowledge. In response, recent studies have proposed integrating external biomedical knowledge into the GML pipeline to realise more precise and interpretable drug discovery with limited training instances. This tutorial presents a comprehensive overviewof long-standing drug discovery principles, provides the foundational concepts and cutting-edge techniques for graph-structured data and knowledge databases, and formally summarises Knowledge-augmented Graph Machine Learning (KaGML) for drug discovery. We have recently completed a survey of KaGML works that organises the outstanding approaches into four categories following a novel-defined taxonomy. This tutorial will present the result of this scholarly work. To encourage audience participation and facilitate research in this promptly emerging field, we also share valuable practical resources for intelligent drug discovery and provide an in-depth discussion of the potential avenues for future advancements.
更多查看译文
关键词
Artificial intelligence,machine learning,deep learning,knowledge-augmented methods,graph machine learning,drug discovery
AI 理解论文
溯源树
样例
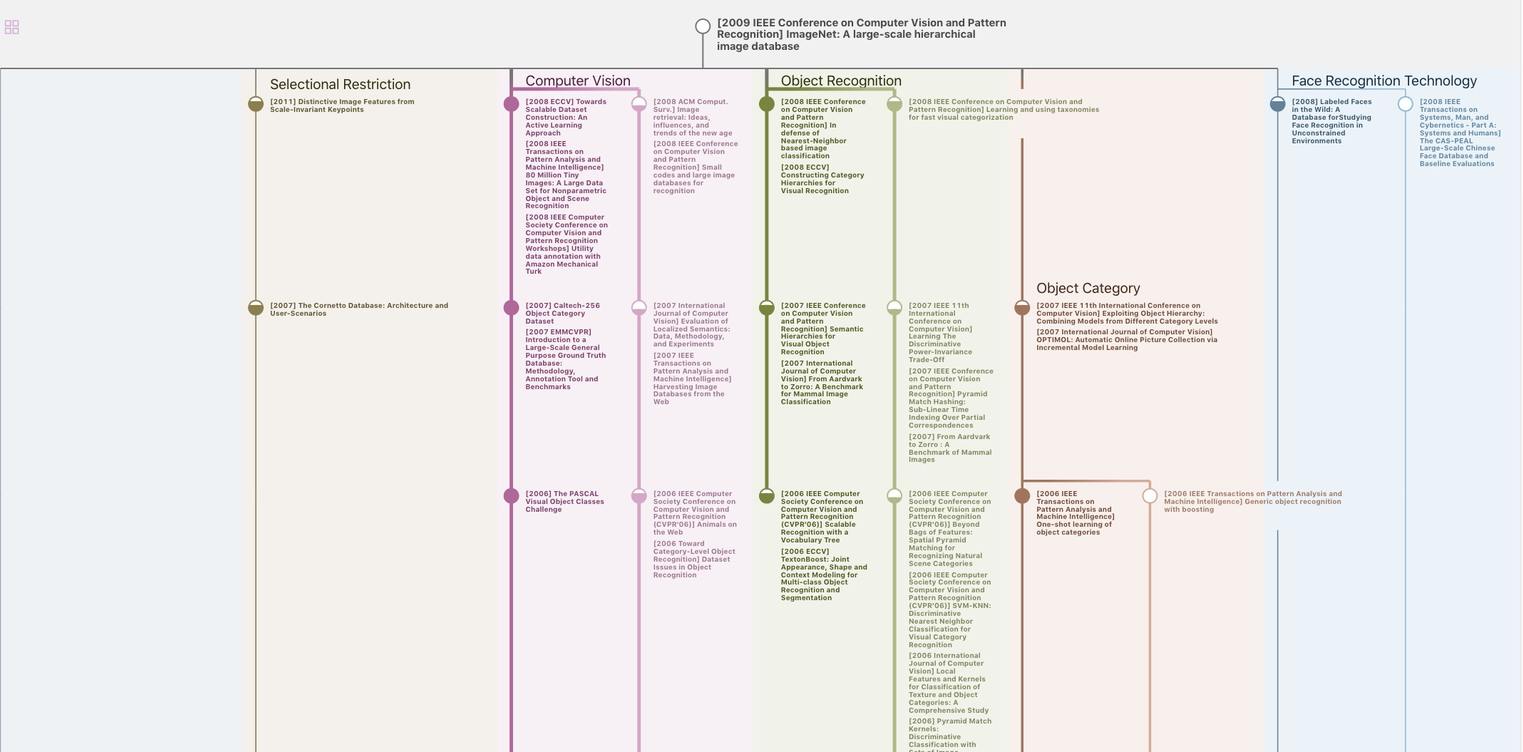
生成溯源树,研究论文发展脉络
Chat Paper
正在生成论文摘要