Graph and Geometry Generative Modeling for Drug Discovery
PROCEEDINGS OF THE 29TH ACM SIGKDD CONFERENCE ON KNOWLEDGE DISCOVERY AND DATA MINING, KDD 2023(2023)
摘要
With the recent progress in geometric deep learning, generative modeling, and the availability of large-scale biological datasets, molecular graph and geometry generative modeling have emerged as a highly promising direction for scientific discovery such as drug design. These generative methods enable efficient chemical space exploration and potential drug candidate generation. However, by representing molecules as 2D graphs or 3D geometries, there exist many both fundamental and challenging problems for modeling the distribution of these irregular and complex relational data. In this tutorial, we will introduce participants to the latest key developments in this field, covering important topics including 2D molecular graph generation, 3D molecular geometry generation, 2D graph to 3D geometry generation, and conditional 3D molecular geometry generation. We further include antibody generation, where we particularly consider large-size antibody molecules. For each topic, we will outline the underlying problem characteristics, summarize key challenges, present unified views of the representative approaches, and highlight future research direction and potential impacts. We anticipate this lecture-style tutorial would attract a broad audience of researchers and practitioners.
更多查看译文
AI 理解论文
溯源树
样例
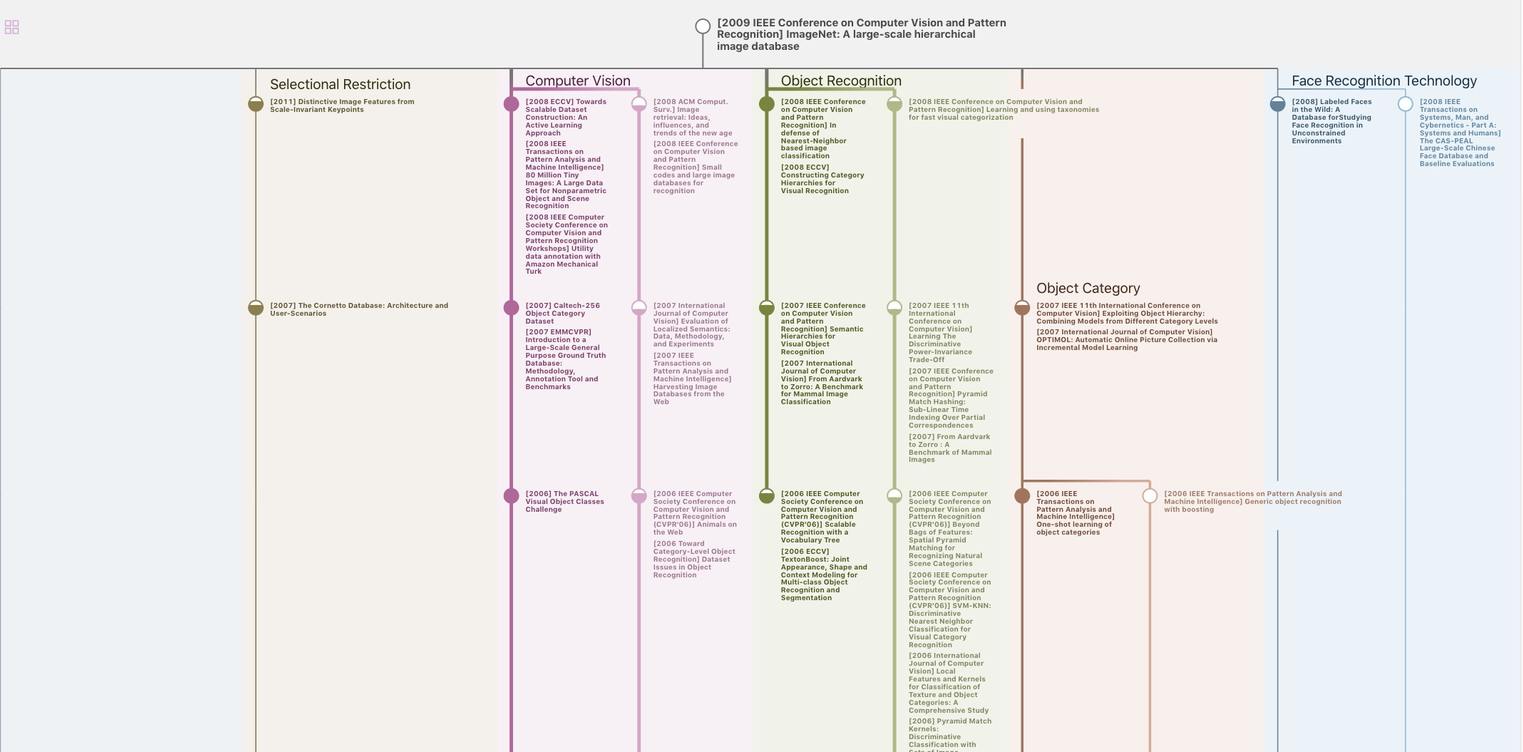
生成溯源树,研究论文发展脉络
Chat Paper
正在生成论文摘要