2D-Pose Based Human Body Segmentation for Weakly-Supervised Concealed Object Detection in Backscatter Millimeter-Wave Images.
ICPR Workshops (1)(2022)
摘要
The detection and localization of anomalies in backscatter images of a person is a standard procedure in airport security screening. Detecting a concealed item on a person and localizing the item to a specific body part requires the ability to recognize and segment distinct body parts. This can be challenging for backscatter images compared with RGB images due to lacking chromaticity cues and the limited availability of annotated backscatter images. To address this problem, we propose a weakly-supervised method for anomaly detection on human body parts which is based on an unsupervised body segmentation procedure that uses keypoints from a pretrained pose estimator to segment backscatter images without significant performance degradation. The paper presents a method for adapting a pretrained RGB pose estimator to segment human body parts in millimeter-wave images. We then train a body part-aware anomaly detection classifier to detect foreign objects on the body part. Our work is applied to TSA’s passenger screening dataset containing backscatter millimeter-wave scan images of airport travelers with binary labels that indicate whether a concealed item is attached to a body part. Our proposed approach significantly improves detection accuracy on 2D images from the baseline approach with a state-of-the-art performance of 97% F1-score and 0.0559 log-loss on TSA-PSD test set.
更多查看译文
关键词
concealed object detection,d-pose,weakly-supervised,millimeter-wave
AI 理解论文
溯源树
样例
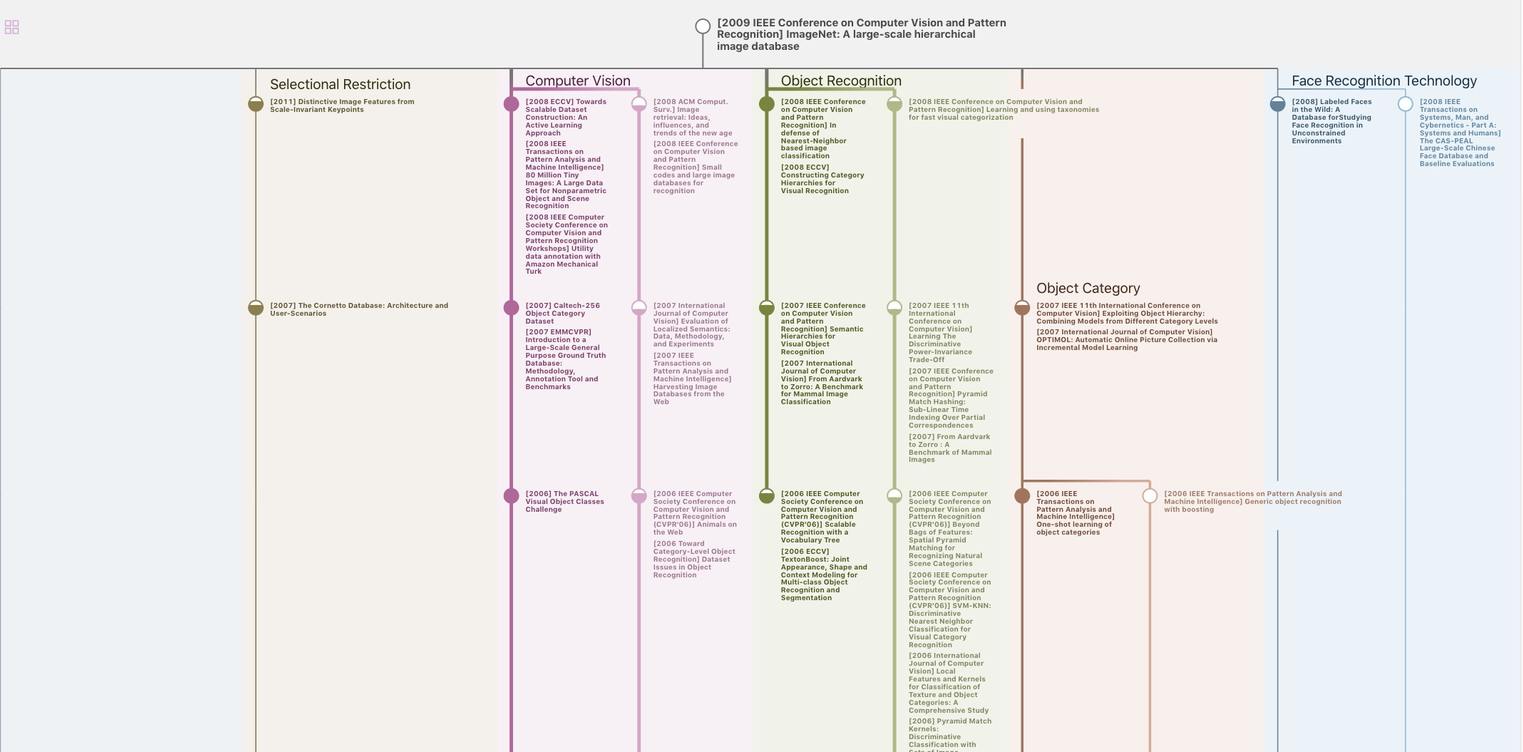
生成溯源树,研究论文发展脉络
Chat Paper
正在生成论文摘要