Visualization and Analysis of Whole Slide Histological Images.
ICPR Workshops (2)(2022)
摘要
The use of modern approaches based on convolutional neural networks (CNNs) for segmentation of whole slide images (WSIs) helps pathologists obtain more stable and quantitative analysis results and improve diagnosis objectivity. But working with WSIs is extremely difficult due to their resolution, size and the presence of a large number of incompatible image storage formats from equipment manufacturers. In addition, the use of modern CNN-based image analysis methods is complicated by the need to use a set of tools with a low level of internal integration. In order to facilitate the interaction of histologists with whole slide images and modern image analysis methods we implemented PathScribe – a new universal cross-platform cloud-based tool for comfortable viewing and manipulating large collections of WSIs on almost any device, including tablets and smartphones. We also consider the important problem of automatic tissue type recognition on WSIs and propose a new CNN-based method of automatic tissue type recognition on WSIs with a 2 subsets of PATH-DT-MSU dataset which contain high-quality whole slide images of digestive tract tumors with tissue type area annotations. The proposed method achieved 0.929 accuracy on CRC-VAL-HE-7K dataset (9 classes) and 0.97 accuracy on PATH-DT-MSU-WSS1, WSS2 datasets (5 classes). The developed method allows to classify the areas corresponding to the gastric own mucous glands in the lamina propria and distinguish the tubular structures of a highly differentiated gastric adenocarcinoma with normal glands.
更多查看译文
关键词
whole slide histological images,visualization
AI 理解论文
溯源树
样例
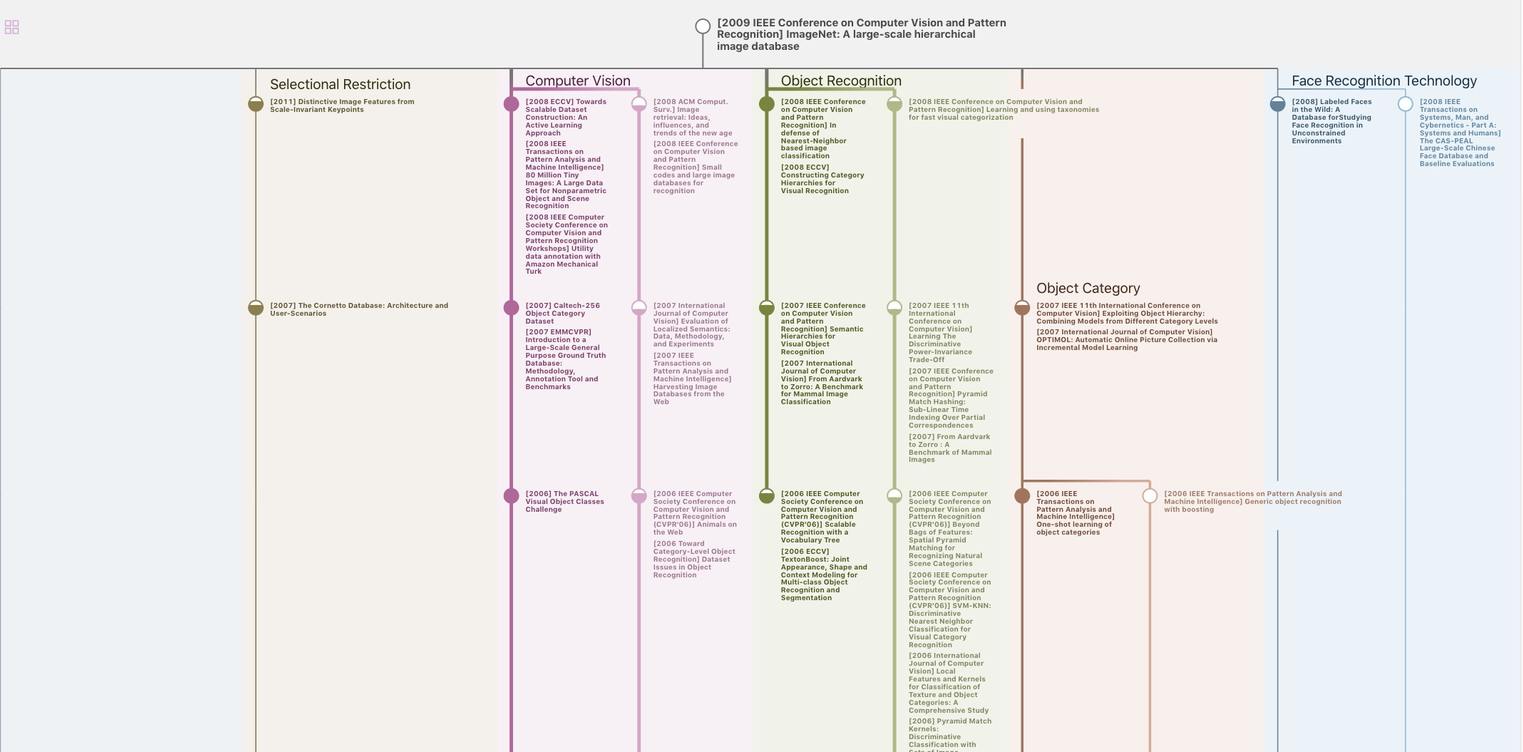
生成溯源树,研究论文发展脉络
Chat Paper
正在生成论文摘要