A PDF Malware Detection Method Using Extremely Small Training Sample Size
PROCEEDINGS OF THE 2023 ACM SYMPOSIUM ON DOCUMENT ENGINEERING, DOCENG 2023(2023)
摘要
Machine learning-based methods for PDF malware detection have grown in popularity because of their high levels of accuracy. However, many well-known ML-based detectors require a large number of specimen features to be collected before making a decision, which can be time-consuming. In this study, we present a novel, distance-based method for detecting PDF malware. Notably, our approach needs significantly less training data compared to traditional machine learning or neural network models. We evaluated our method using the Contagio dataset and reported that it can detect 90.50% of malware samples with only 20 benign PDF files used for model training. To show the statistical significance, we reported results with a 95% confidence interval (CI). We evaluated our model's performance across multiple metrics including Accuracy, F1 score, Precision, and Recall, alongside False Positive Rate, False Negative Rates, True Positive Rate and True Negative Rates. This paper highlights the feasibility of using distance-based methods for PDF malware detection, even with limited training data, thereby offering a promising direction for future research.
更多查看译文
AI 理解论文
溯源树
样例
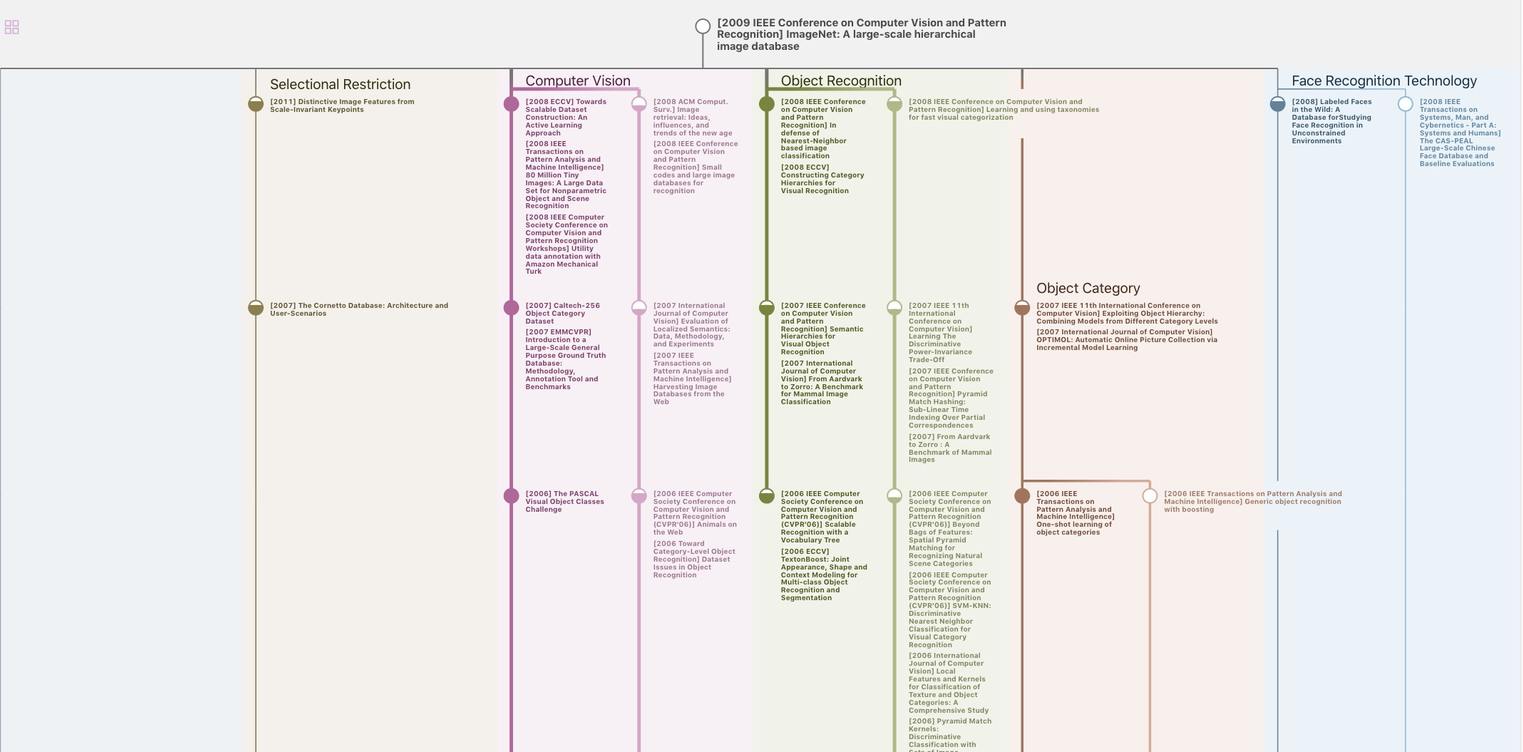
生成溯源树,研究论文发展脉络
Chat Paper
正在生成论文摘要