Exploiting Label Dependencies for Multi-Label Document Classification Using Transformers
PROCEEDINGS OF THE 2023 ACM SYMPOSIUM ON DOCUMENT ENGINEERING, DOCENG 2023(2023)
摘要
We introduce in this paper a new approach to improve deep learning-based architectures for multi-label document classification. Dependencies between labels are an essential factor in the multi-label context. Our proposed strategy takes advantage of the knowledge extracted from label co-occurrences. The proposed method consists in adding a regularization term to the loss function used for training the model, in a way that incorporates the label similarities given by the label co-occurrences to encourage the model to jointly predict labels that are likely to co-occur, and and not consider labels that are rarely present with each other. This allows the neural model to better capture label dependencies. Our approach was evaluated on three datasets: the standard AAPD dataset, a corpus of scientific abstracts and Reuters-21578, a collection of news articles, and a newly proposed multi-label dataset called arXiv-ACM. Our method demonstrates improved performance, setting a new state-of-the-art on all three datasets.
更多查看译文
关键词
Multi-label Classification,Document Classification,BERT,Transformers,Label Dependencies
AI 理解论文
溯源树
样例
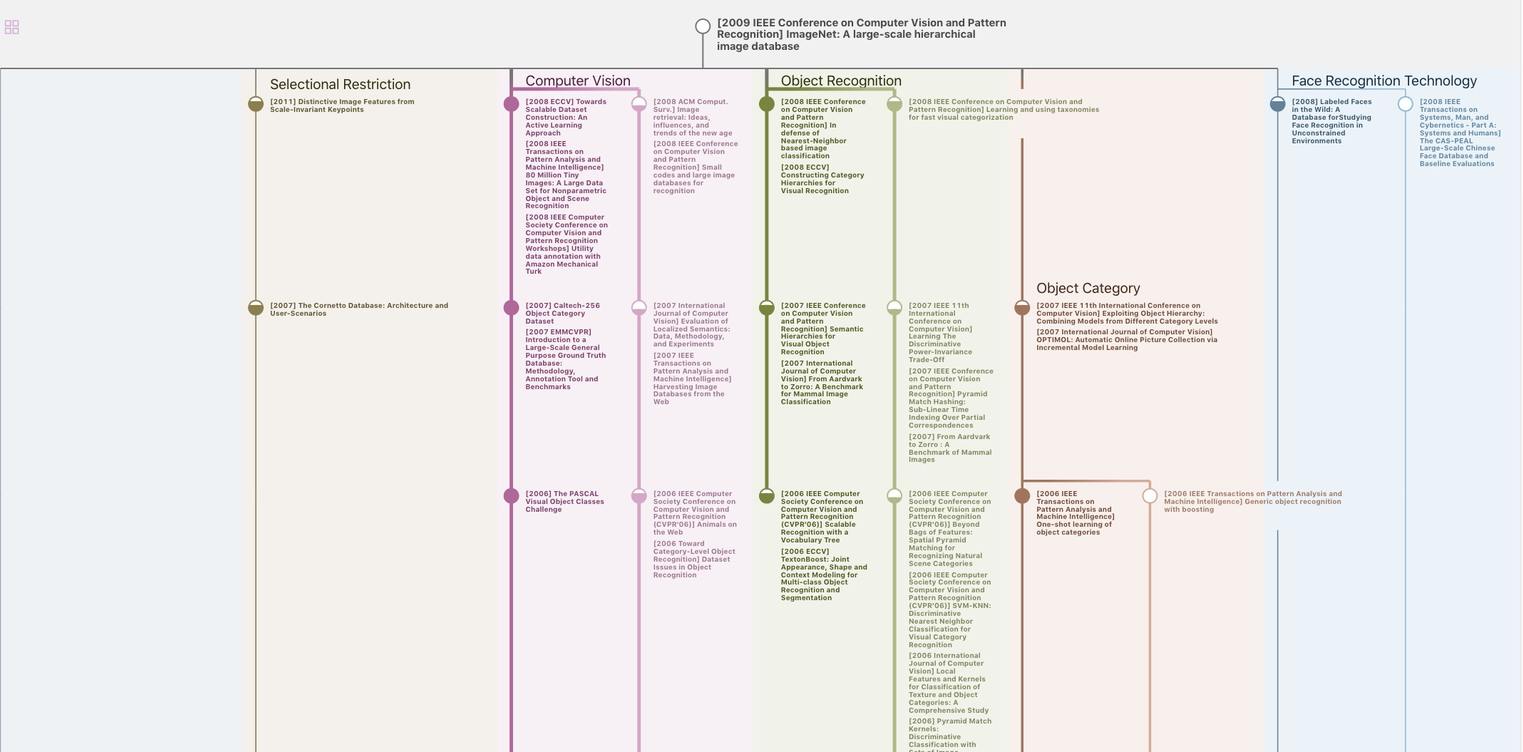
生成溯源树,研究论文发展脉络
Chat Paper
正在生成论文摘要