AIFORE: Smart Fuzzing Based on Automatic Input Format Reverse Engineering
PROCEEDINGS OF THE 32ND USENIX SECURITY SYMPOSIUM(2023)
摘要
Knowledge of a program's input format is essential for effective input generation in fuzzing. Automated input format reverse engineering represents an attractive but challenging approach to learning the format. In this paper, we address several challenges of automated input format reverse engineering, and present a smart fuzzing solution AIFORE which makes full use of the reversed format and benefits from it. The structures and semantics of input fields are determined by the basic blocks (BBs) that process them rather than the input specification. Therefore, we first utilize byte-level taint analysis to recognize the input bytes processed by each BB, then identify indivisible input fields that are always processed together with a minimum cluster algorithm, and learn their types with a neural network model that characterizes the behavior of BBs. Lastly, we design a new power scheduling algorithm based on the inferred format knowledge to guide smart fuzzing. We implement a prototype of AIFORE and evaluate both the accuracy of format inference and the performance of fuzzing against state-of-the-art (SOTA) format reversing solutions and fuzzers. AIFORE significantly outperforms SOTA baselines on the accuracy of field boundary and type recognition. With AIFORE, we uncovered 20 bugs in 15 programs that were missed by other fuzzers.
更多查看译文
AI 理解论文
溯源树
样例
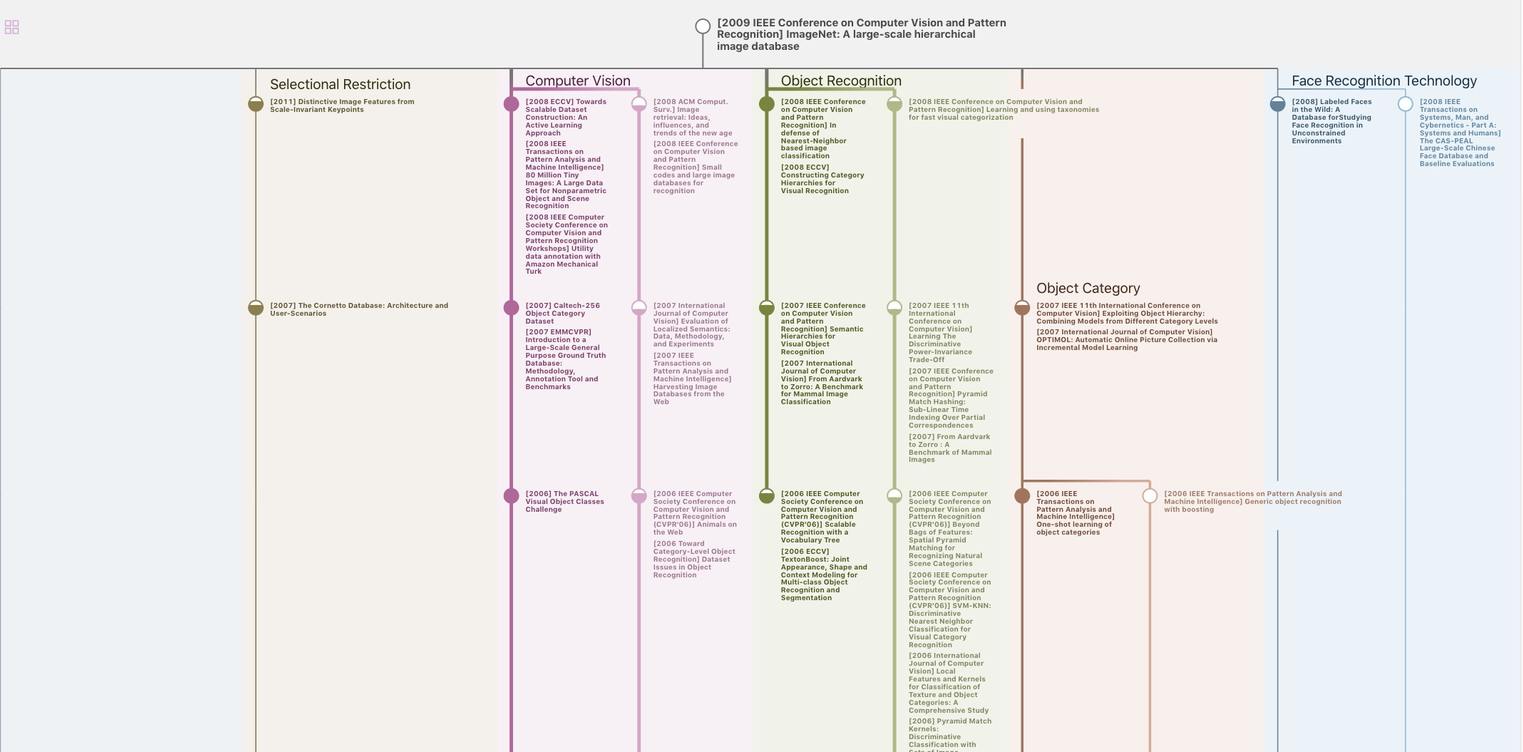
生成溯源树,研究论文发展脉络
Chat Paper
正在生成论文摘要