Towards prediction of Quality of Life aspects using wearable data with limited ground truth
PETRA(2023)
摘要
Today people live with a variety of diseases and health conditions that can impact their daily lives, from chronic illnesses such as cancer to mental health issues such as anxiety and depression. With the advancement of technology and healthcare, quality of life (QoL) prediction and improvement is becoming increasingly important to help individuals live better. In addition to data collected by questionnaires, machine learning can be employed to predict aspects of QoL, such as fatigue and anxiety, based on data from wearable devices. However, this requires ground truth information, which, in real-world cases is not always present, or is scarce. This paper addresses the problem of fatigue prediction, which is part of the overall QoL prediction. We extend previous literature by viewing the problem as regression, with the ultimate goal of transferring a model trained with a complete dataset of the literature to a real-world dataset with no ground truth, facing the challenge of only a few common features. We compare several regression and classification techniques to select the best-performing ones. We then explore the effect of using only limited important features on accuracy, which allow us to assess the applicability of transferring the models to a real-world dataset. We apply the trained model on data from the ONCORELIEF project and discuss the identified challenges.
更多查看译文
关键词
QOL,Wearable sensors,questionnaires,Machine Learning,Deep Learning
AI 理解论文
溯源树
样例
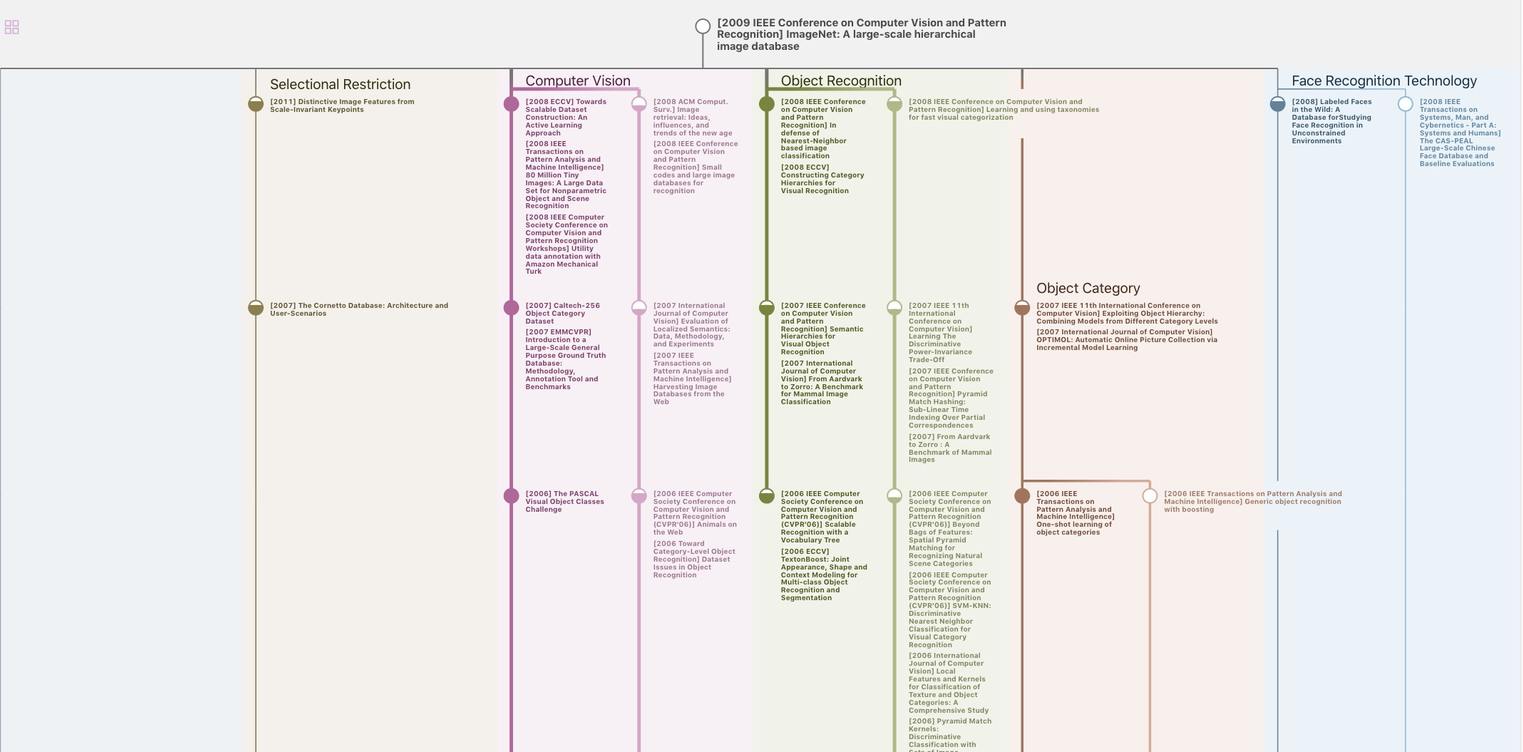
生成溯源树,研究论文发展脉络
Chat Paper
正在生成论文摘要