DSS: Synthesizing Long Digital Ink Using Data Augmentation, Style Encoding and Split Generation.
ICDAR (4)(2023)
摘要
As text generative models can give increasingly long answers, we tackle the problem of synthesizing long text in digital ink. We show that the commonly used models for this task fail to generalize to long-form data and how this problem can be solved by augmenting the training data, changing the model architecture and the inference procedure. These methods use contrastive learning technique and are tailored specifically for the handwriting domain. They can be applied to any encoder-decoder model that works with digital ink. We demonstrate that our method reduces the character error rate on long-form English data by half compared to baseline RNN and by 16% compared to the previous approach that aims at addressing the same problem. We show that all three parts of the method improve recognizability of generated inks. In addition, we evaluate synthesized data in a human study and find that people perceive most of generated data as real.
更多查看译文
关键词
long digital ink,style encoding,data augmentation,split generation
AI 理解论文
溯源树
样例
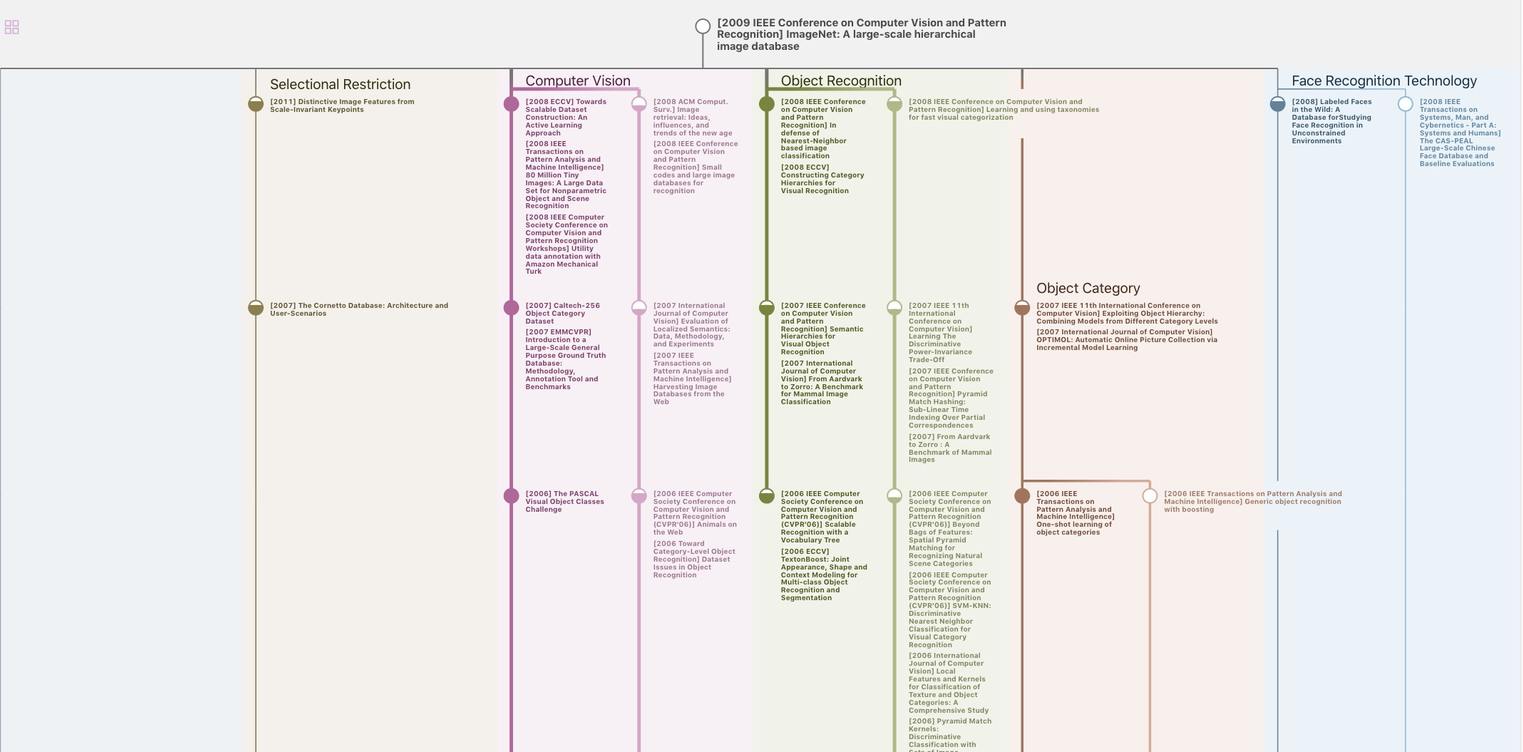
生成溯源树,研究论文发展脉络
Chat Paper
正在生成论文摘要