OSCA: Online User-managed Server Selection and Configuration Adaptation for Interactive MAR
IWQoS(2023)
摘要
Interactive mobile augmented reality (MAR) applications such as Connected Lens are becoming popular, which often rely on deep neural network (NN)-based video analytics techniques to understand the real world. However, performing computation-intensive NN inference on resource-constrained mobile devices is impractical. It is thus proposed to offload the workloads to edge servers with the help of mobile edge computing (MEC). Existing works often focus on system-wide offloading solutions, optimizing the personalized user experience for interactive applications in dynamic environments is yet rarely studied, where multiple challenges remain to be solved. First, the user has to decide the configuration for video analytics, where the inherent accuracy-cost trade-off exists. Second, it is intractable to decide the target server for offloading, since each server supports limited configurations, and a user needs to balance the experience of analytics service and the quality of interaction with others at the same time. Third, the fluctuating network information is often undisclosed to the users, and the candidate servers also vary over time. Therefore, in this paper, we propose an online user-managed server selection and configuration adaptation scheme (OSCA). Via Lyapunov optimization, we aim to maximize the long-term service experience, under the interactive quality constraint with other users. Besides, volatile multi-armed bandit (MAB) is utilized to handle the network fluctuation and the variance of the candidate servers. We conduct rigorous theoretical analysis, and the deviations of both the service experience and the interactive quality are bounded. Through extensive trace-driven experiments, we demonstrate the superior performance of OSCA.
更多查看译文
关键词
Mobile edge computing,mobile augmented reality,interactive applications,online learning
AI 理解论文
溯源树
样例
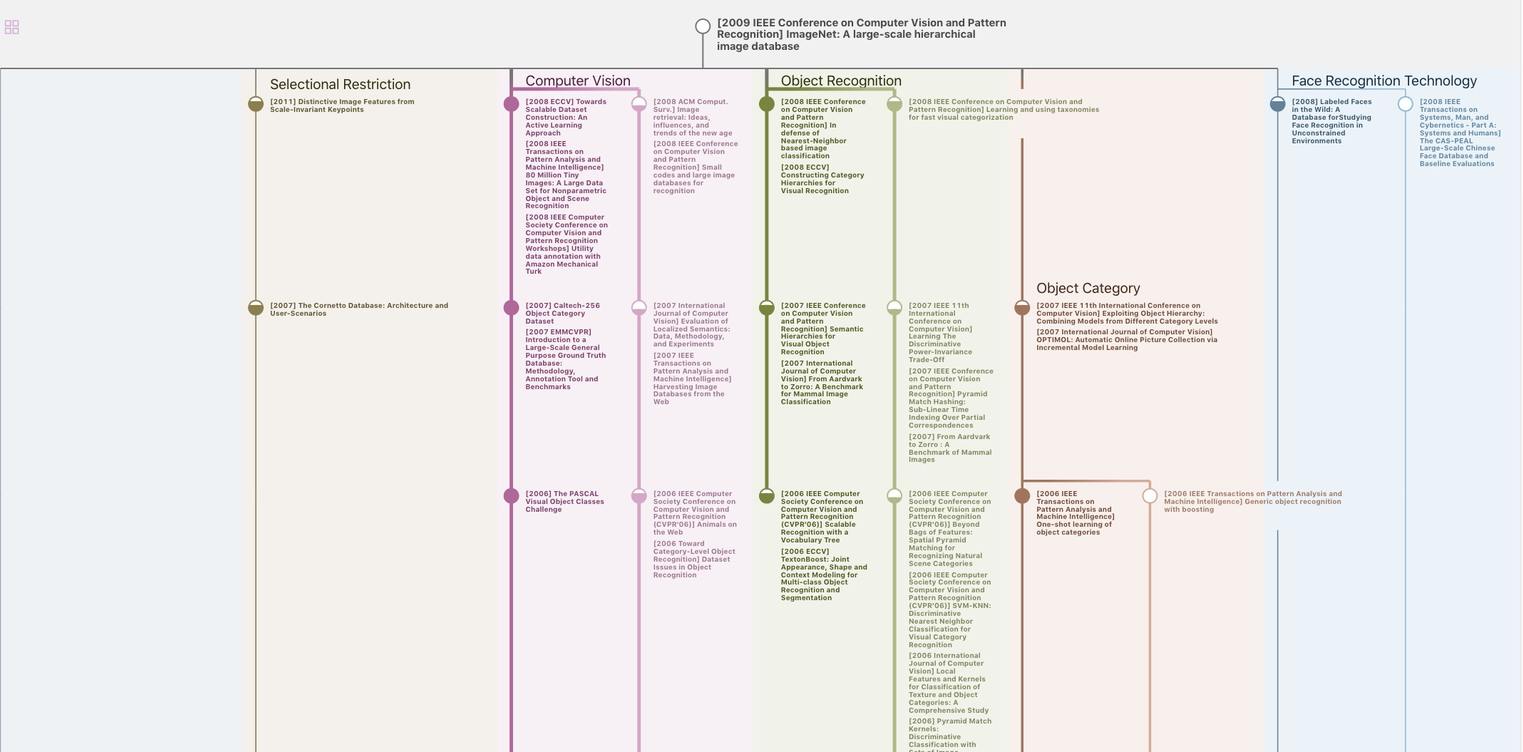
生成溯源树,研究论文发展脉络
Chat Paper
正在生成论文摘要