You Can Trade Your Experience in Distributed Multi-Agent Multi-Armed Bandits.
IWQoS(2023)
摘要
Multi-Armed Bandit (MAB) that solves the sequential decision-making to the prior-unknown settings has been extensively studied and adopted in various applications such as online recommendation, transmission rate allocation, etc. Although some recent work has investigated the multi-agent MAB model, they supposed that agents share their bandit information based on social networks but neglected the incentives and arm-pulling budget for heterogeneous agents. In this paper, we propose a transaction-based multi-agent MAB framework, where agents can trade their bandit experience with each other to improve their total individual rewards. Agents not only face the dilemma between exploitation and exploration, but also decide to post a suitable price for their bandit experience. Meanwhile, as a buyer, the agent accepts another agent whose experience will help her the most, according to the posted price and her risk-tolerance level. The key challenge lies in that the arm-pulling and experience-trading decisions affect each other. To this end, we design the transaction-based upper confidence bound to estimate the prior-unknown rewards of arms, based on which the agents pull arms or trade their experience. We prove the regret bound of the proposed algorithm for each independent agent and conduct extensive experiments to verify the performance of our solution.
更多查看译文
关键词
Multi-agent multi-armed bandits,upper confidence bound,experience transaction,posted pricing mechanism
AI 理解论文
溯源树
样例
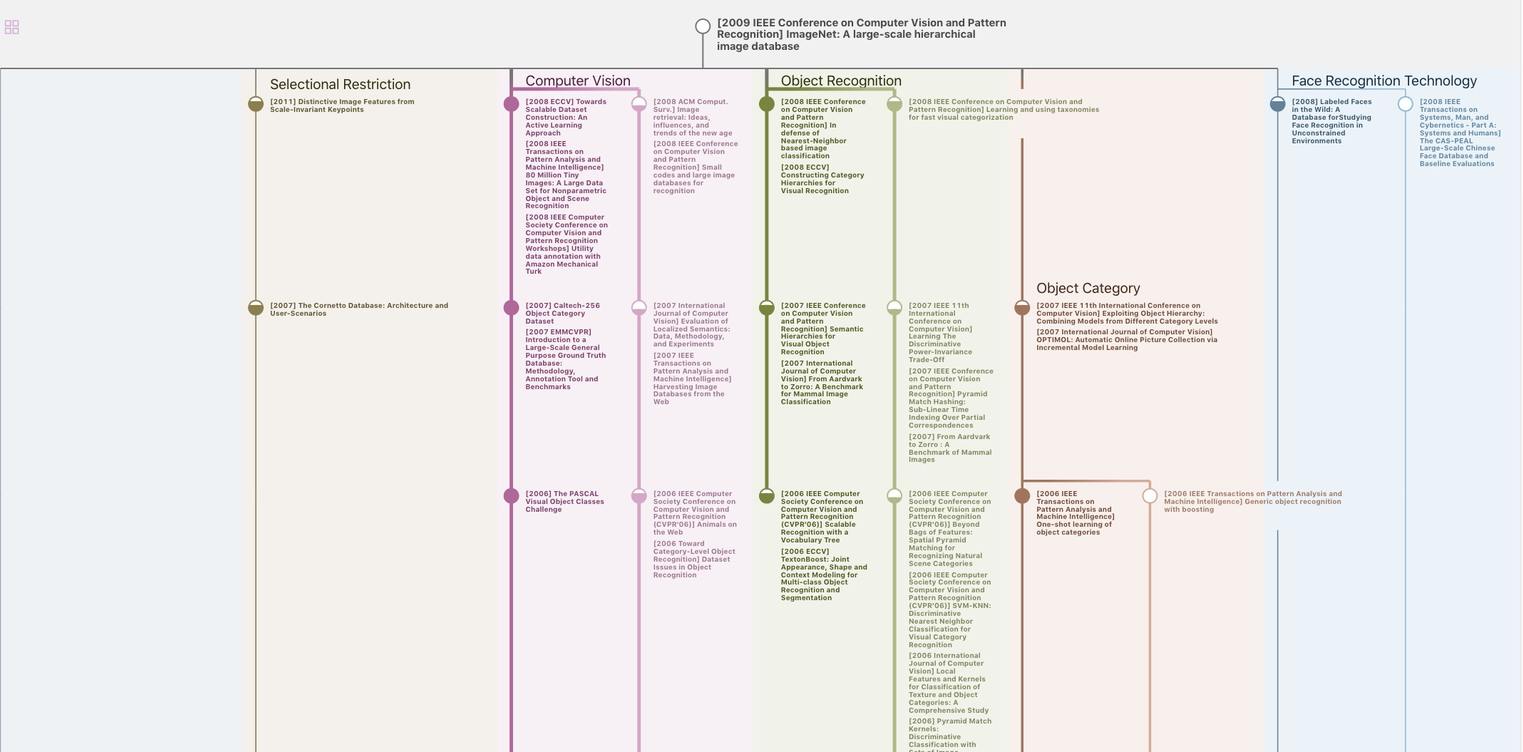
生成溯源树,研究论文发展脉络
Chat Paper
正在生成论文摘要