Optimization and Performance Evaluation of Hybrid Deep Learning Models for Traffic Flow Prediction
VTC2023-Spring(2023)
摘要
Traffic flow prediction has been regarded as a critical problem in intelligent transportation systems. An accurate prediction can help mitigate congestion and other societal problems while facilitating safer, cost and time-efficient travel. However, this requires the prediction algorithm to consider several complex characteristics of traffic flow data. These complex characteristics are an amalgamation of the spatial, temporal and periodic features exhibited by traffic flow data. To extract and leverage these features for traffic flow prediction, several hybrid deep learning models have been developed recently; however, there are still some challenges to determine the optimal architecture considering both spatial and temporal features. In this work, we perform an extensive comparison of hybrid deep learning models with and without periodicity to understand the prediction accuracy of these popular models. We propose an optimization framework that unifies genetic algorithm (GA) embedded optimization with prediction models in order to derive optimized deep learning architectures for hybrid traffic flow prediction exploring 2D spatial and temporal information. The framework enables the improvement of prediction performance and eliminates the hand-tuning process. An improved temporal convolutional network (TCN) architecture is derived using the GA driven optimization, which achieves superior traffic flow prediction accuracy compared to all other existing hybrid deep learning models on the freeway and urban traffic data from the PeMS traffic data set. We also evaluate the performance of the derived hybrid deep learning algorithms on the Raspberry PI embedded platform.
更多查看译文
关键词
Traffic flow prediction,Hybrid deep learning models,Temporal convolutional networks
AI 理解论文
溯源树
样例
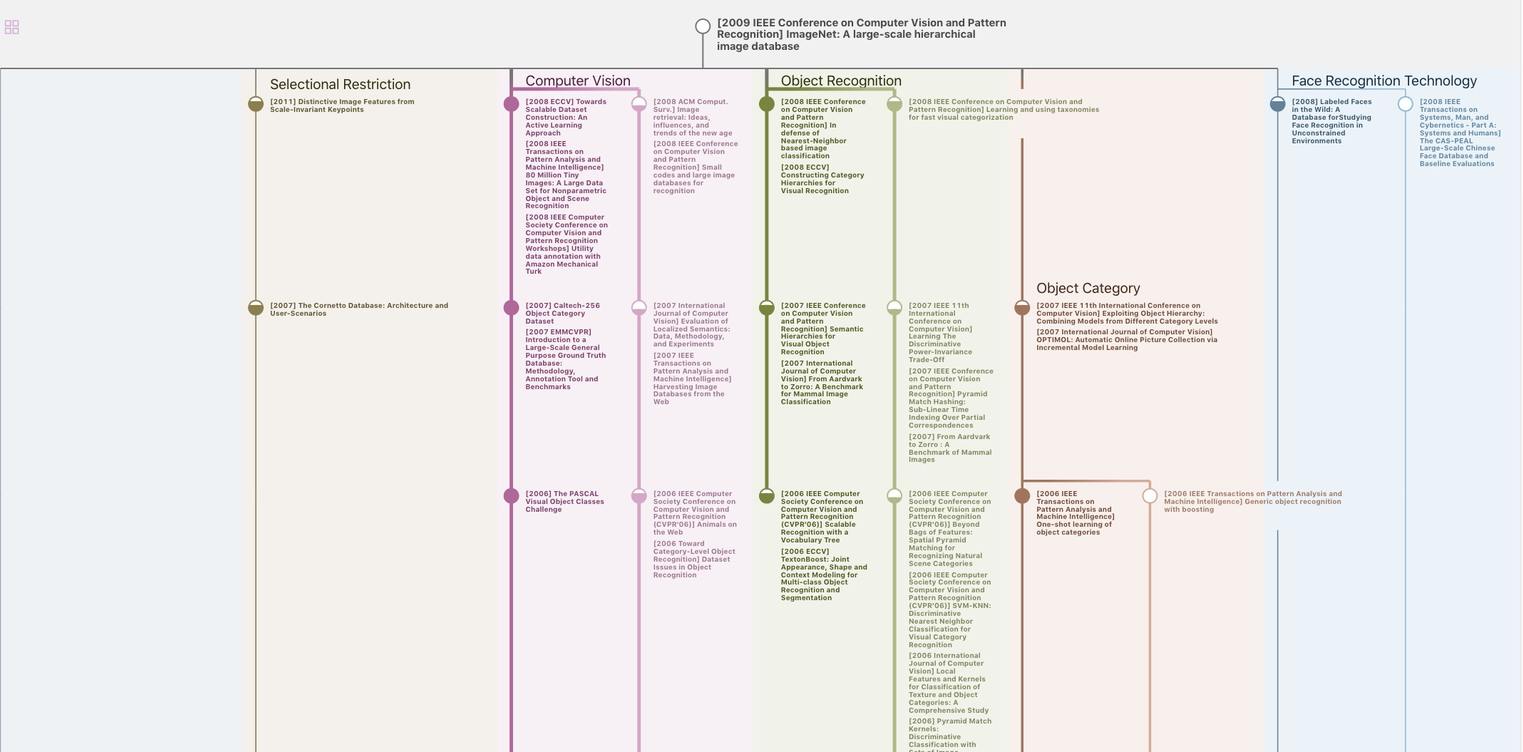
生成溯源树,研究论文发展脉络
Chat Paper
正在生成论文摘要