Safe and Fast Reinforcement Learning for Network Slicing Resource Allocation
VTC2023-Spring(2023)
摘要
Network slicing enables operators to virtually partition network resources and instantiate different virtual networks, supporting flexible quality of service, in the form of service level agreements (SLAs). Optimizing resource allocation for network slicing is a complex task, given the dynamicity and randomness of network conditions. Leveraging principles of reinforcement learning, we propose an algorithmic solution to optimize the SLA success rate across a radio access network. Tailoring the algorithm to the problem at hand, and leveraging some prior knowledge on the system to be optimized, we ensure fast convergence, data efficiency, and safe exploration. The solution is scalable both in the number of cells and in the number of slices. Extensive numerical evaluations on a simulated environment show the effectiveness of the proposed solution and its advantages versus both simple baselines and sophisticated solutions based on deep reinforcement learning, in terms of speed of convergence and SLA success rate.
更多查看译文
关键词
algorithmic solution,deep reinforcement learning,different virtual networks,leveraging principles,network conditions,network slicing resource allocation,radio access network,safe exploration,service level agreements,SLA success rate,virtually partition network resources
AI 理解论文
溯源树
样例
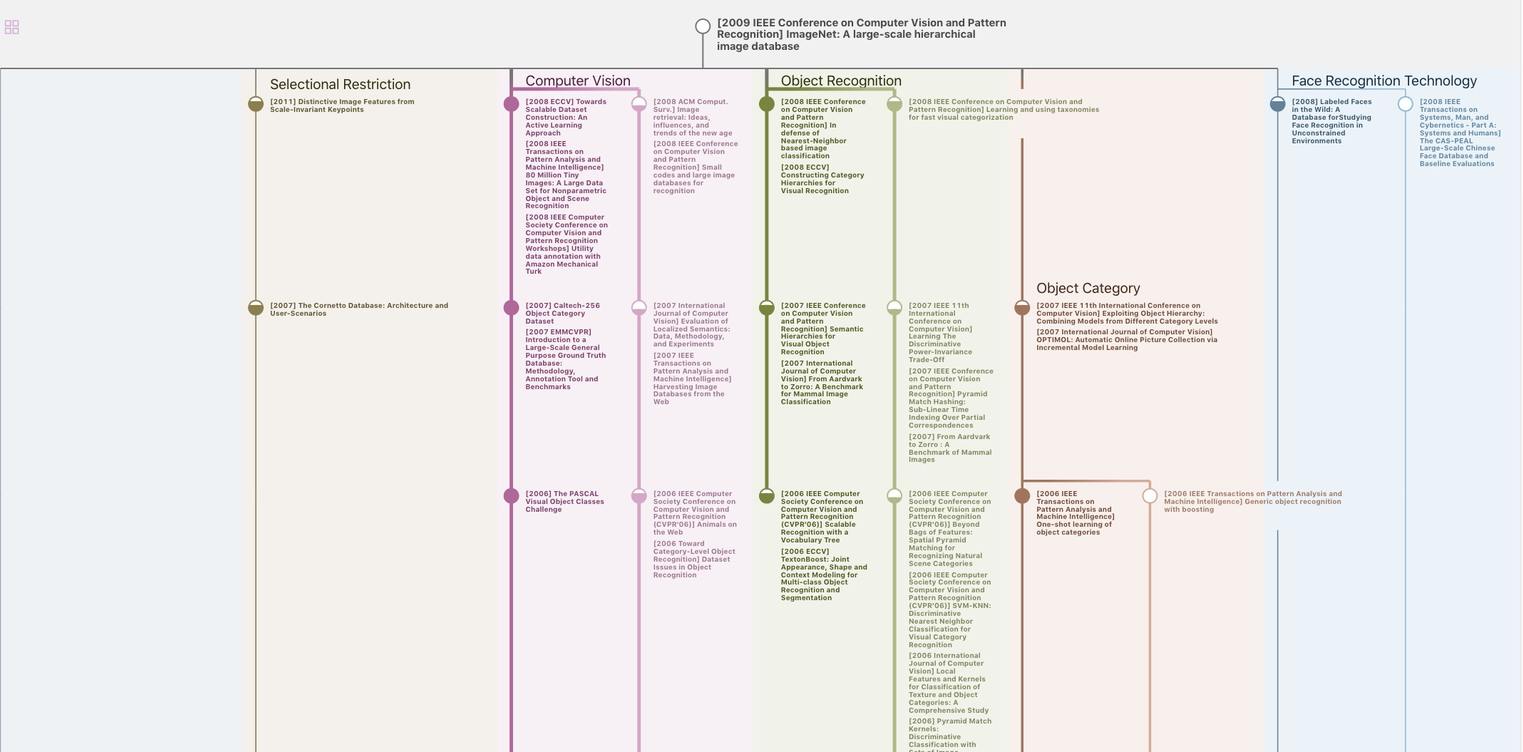
生成溯源树,研究论文发展脉络
Chat Paper
正在生成论文摘要