A Machine Learning Approach for Detecting GPS Location Spoofing Attacks in Autonomous Vehicles
VTC2023-Spring(2023)
摘要
Connected and Autonomous Vehicles (CAV) depend on satellite systems, such as the Global Positioning System (GPS), for location awareness. Location data are streamed in real-time to the CAV's perception engine from its onboard GPS receiver for autonomous driving and navigation. However, these receivers are vulnerable to location spoofing attacks that can be easily launched using Commercial-Off-The-Self (COTS) equipment and opensource software. Existing data-driven attack detection solutions typically require data associated with 'normal' and 'attack' labels. The latter are hard to collect in operational conditions or even in controlled experiments. To this end, we formulate the GPS location spoofing attack detection as an outlier detection problem. The proposed solution based on Machine Learning (ML) relies solely on normal location data for training during attack-free operation. Our solution demonstrates more than 98% detection accuracy according to standard metrics on realistic data produced with the CARLA driving simulator and outperforms by 15% another (non ML-based) state-of-the-art solution.
更多查看译文
关键词
Location spoofing,attack detection,machine learning,autonomous vehicles,cybersecurity
AI 理解论文
溯源树
样例
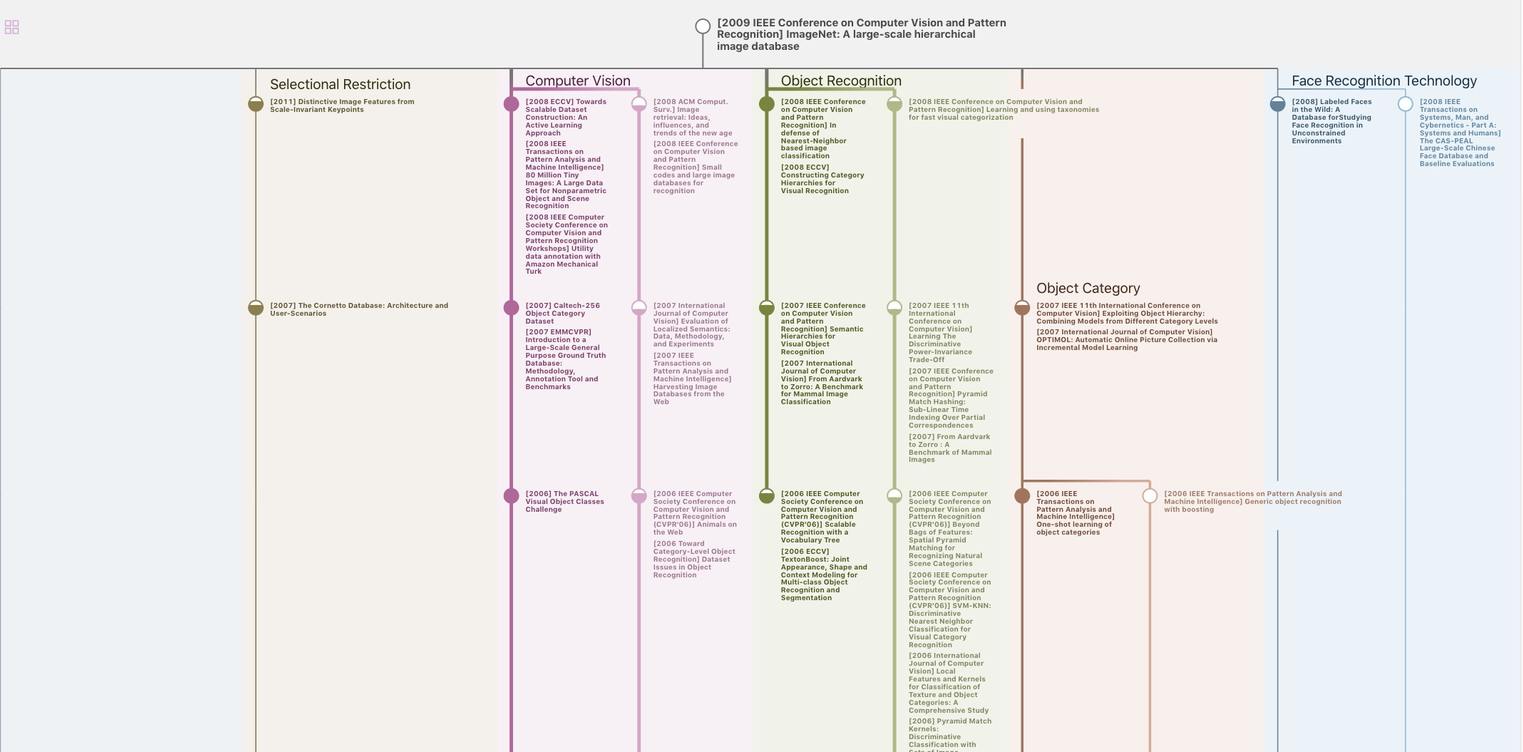
生成溯源树,研究论文发展脉络
Chat Paper
正在生成论文摘要