FedATM: Adaptive Trimmed Mean based Federated Learning against Model Poisoning Attacks
VTC2023-Spring(2023)
摘要
Federated learning (FL) has received explosive research attention in that it enables multiple clients to collaboratively train a global model without sharing raw data in between, thereby facilitating the protection of data privacy. Typically, FL can converge well after a couple of communication rounds, but its convergence is vulnerable to the model poisoning attacks induced by fake clients. Existing works have been devoted to designing various post-processing techniques to alleviate the adverse effects of the model poisoning attacks, they, however, fail to accurately trim off the local models of fake clients while keeping those of benign clients intact during model averaging. In this paper, we investigate the problem of model poisoning attacks in federated learning (FL). To cope with this problem, we design the federated adaptive trimmed mean (FedATM) algorithm, where the clients are sorted in accordance with the distances between local models, and a distance-based threshold is designed to detect the presence of fake clients, thereby preventing the fake local models from destroying the accuracy of model averaging. Simulation results show that the proposed FedATM algorithm is robust to model poisoning attacks as compared to several comparison schemes under various data heterogeneities.
更多查看译文
关键词
adaptive trimmed mean based federated learning,data heterogeneities,distance-based threshold,fake clients,fake local models,FedATM,FL,global model,model averaging,model poisoning attacks
AI 理解论文
溯源树
样例
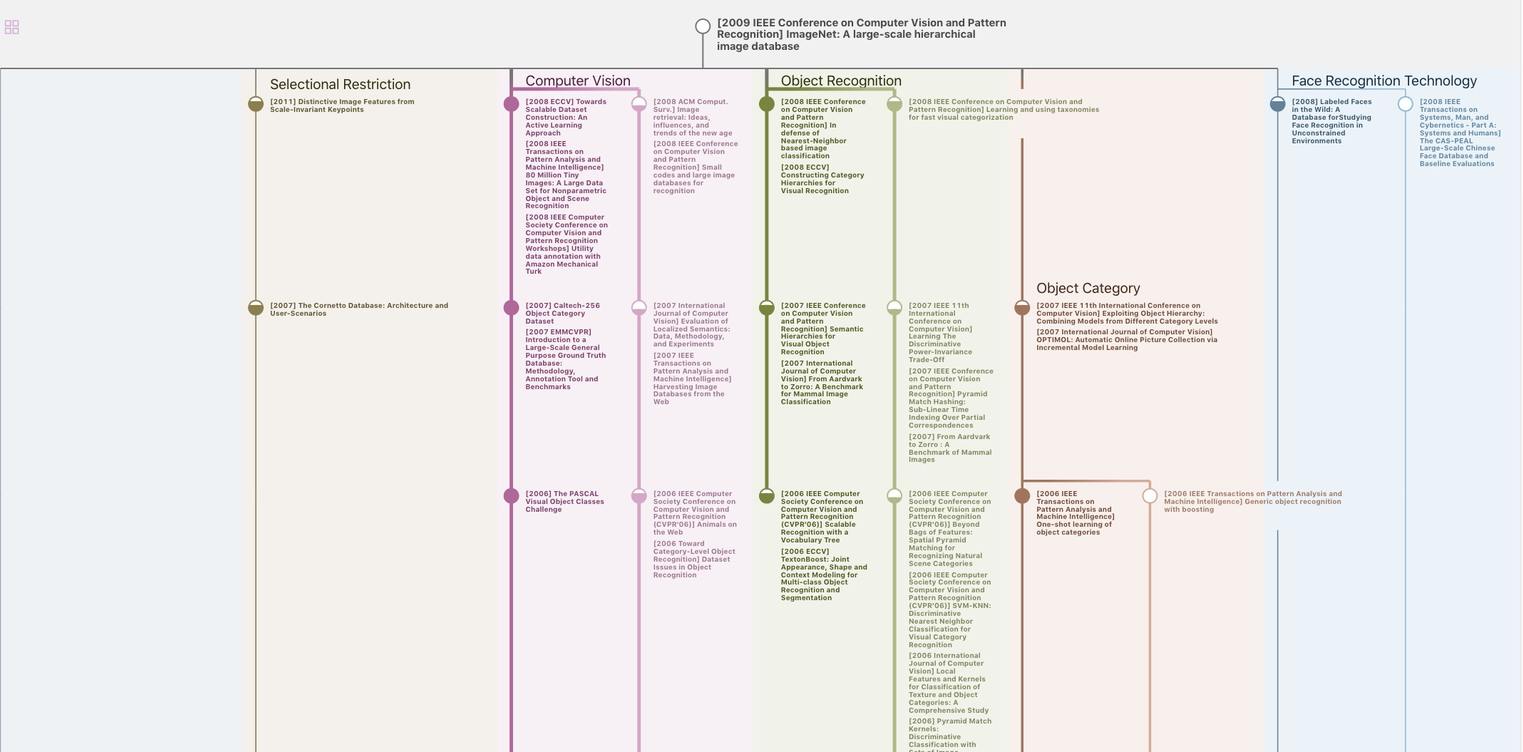
生成溯源树,研究论文发展脉络
Chat Paper
正在生成论文摘要